The integration of artificial intelligence (AI) into data analysis has revolutionized how businesses extract and utilize insights, transforming raw data into actionable strategies. In the past, the sheer volume of data generated by companies often remained untapped due to the limitations of traditional analytical methods. However, AI has broken these barriers, enabling the processing and analysis of vast datasets with unprecedented speed and accuracy.
AI-driven insights are now at the core of modern business intelligence, allowing companies to make more informed decisions, optimize operations, and maintain a competitive edge in an increasingly data-driven marketplace. By leveraging machine learning algorithms, natural language processing, and advanced data analytics, businesses can uncover hidden patterns, predict future trends, and gain a deeper understanding of customer behaviors and market dynamics.
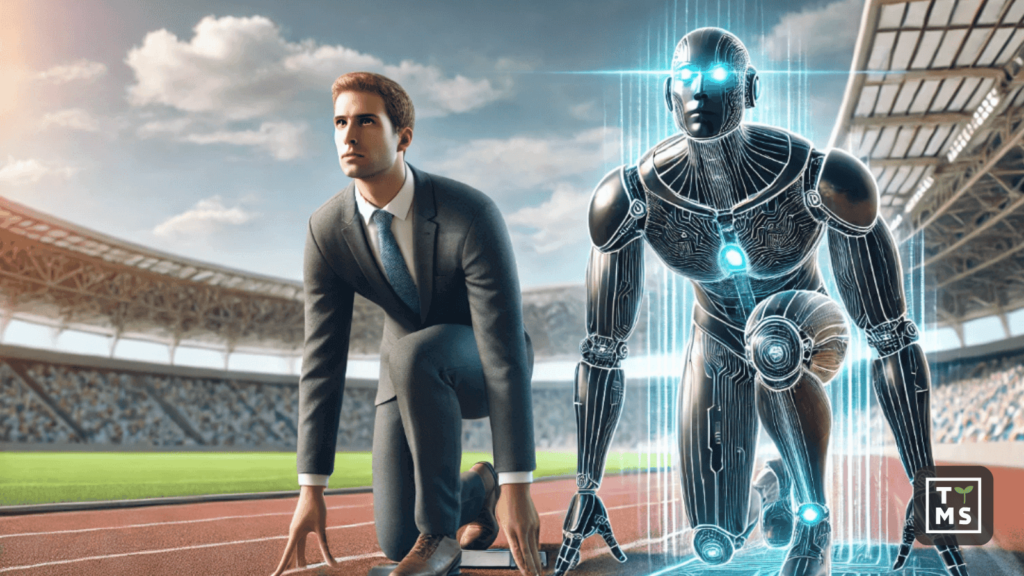
1. What Are Actionable Insights?
Actionable insights are meaningful observations derived from data analysis that provide specific recommendations for taking tangible actions. These insights help organizations make informed decisions, optimize processes, identify opportunities, and improve overall performance. The key characteristics of actionable insights include specificity, relevance, clarity, timeliness, feasibility, and measurability. Let’s delve into these characteristics with examples to illustrate their application and significance.
1.1 Specificity
Actionable insights are clearly defined and focus on particular aspects or problems, providing specific recommendations. For example, a retail company might analyze customer purchase data and discover that sales of a particular product spike during holiday seasons. An actionable insight from this analysis could be to increase inventory of that product in anticipation of holiday demand, coupled with targeted marketing campaigns to capitalize on the trend.
1.2 Relevance
Relevant insights are directly related to the organization’s goals, objectives, or challenges, addressing the specific needs and priorities of the business. For instance, a healthcare provider might use data analytics to identify that a significant number of patient readmissions are due to improper post-discharge care. The actionable insight here would be to implement a follow-up care program that includes regular check-ins with discharged patients, thereby reducing readmission rates and improving patient outcomes.
1.3 Clarity
Clear and understandable insights ensure that stakeholders can easily comprehend and act upon the information provided. For example, a marketing team might analyze customer feedback and find that users frequently mention difficulty navigating their website. The actionable insight could be to redesign the website’s user interface to make it more intuitive, thus enhancing user experience and potentially increasing conversion rates.
1.4 Timeliness
Timely insights allow businesses to act promptly, resolving urgent issues and making relevant decisions quickly. For example, an airline might use real-time data analytics to monitor weather conditions and predict flight delays. The actionable insight would be to proactively inform passengers about potential delays and adjust flight schedules accordingly, thereby improving customer satisfaction and operational efficiency.
1.5 Feasibility
Feasible insights take into account the organization’s capabilities and resources, suggesting practical and realistic actions. For instance, a small business might analyze its sales data and find that a significant portion of revenue comes from repeat customers. An actionable insight would be to implement a loyalty program to further encourage repeat business. The feasibility aspect ensures that the business can afford and manage such a program within its existing operational framework.
1.6 Measurability
Measurable insights often come with key performance indicators (KPIs), allowing organizations to track progress and assess the impact of their actions. For example, a tech company might use data analysis to identify a decline in user engagement on their app. An actionable insight could be to introduce new features aimed at boosting engagement, with KPIs set to measure the increase in user interactions post-implementation.
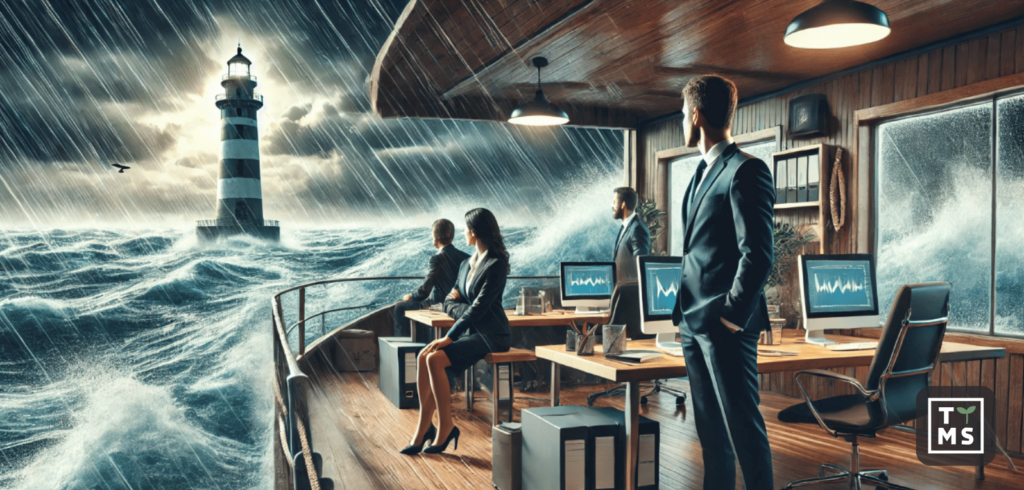
2. Practical Applications of Actionable Insights
2.1 Predictive Maintenance in Manufacturing
In manufacturing, predictive maintenance uses AI to analyze machine data and predict equipment failures before they happen. For example, sensors on a production line might monitor vibrations, temperatures, and other parameters. If the data indicates an unusual pattern that typically precedes a machine breakdown, the actionable insight would be to schedule maintenance immediately, thereby preventing costly downtime and extending the lifespan of the machinery.
2.2 Customer Segmentation in Marketing
A marketing team might use AI to segment customers based on purchasing behavior, demographics, and engagement metrics. For instance, if data analysis reveals that a specific segment of customers responds well to email promotions while another prefers social media ads, the actionable insight would be to tailor marketing campaigns to these preferences. This targeted approach can significantly improve marketing ROI and customer satisfaction.
2.3 Inventory Management in Retail
Retailers can use AI-driven insights to optimize inventory management. For example, if data analysis shows that certain products have seasonal demand peaks, the actionable insight would be to adjust inventory levels accordingly. This ensures that the right products are available at the right time, reducing the risk of stockouts or overstock situations.
2.4 Fraud Detection in Finance
Financial institutions can leverage AI to detect fraudulent activities by analyzing transaction patterns. If the system identifies transactions that deviate from a customer’s usual behavior, the actionable insight would be to flag these transactions for further investigation. This proactive approach helps in preventing fraud and protecting customers’ assets.
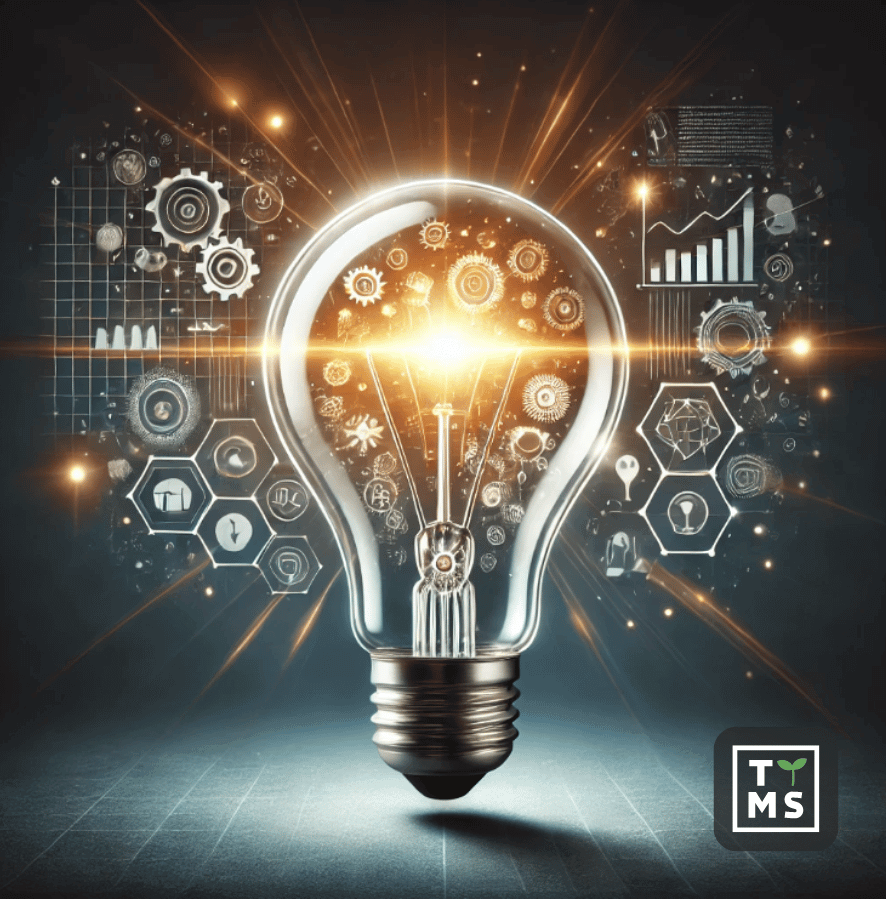
3. Benefits of Using AI in Data Analysis
The integration of artificial intelligence (AI) into data analysis offers a myriad of benefits that can significantly enhance business operations and strategy. Here are some of the key advantages:
3.1 Improved Decision-Making
AI-driven data analysis provides businesses with precise and actionable insights, enabling them to make well-informed decisions. By analyzing vast amounts of data quickly and accurately, AI helps companies identify trends, patterns, and anomalies that might not be apparent through traditional methods. This data-driven approach reduces reliance on intuition and guesswork, thereby increasing the accuracy and effectiveness of business decisions.
3.2 Enhanced Efficiency and Productivity
One of the most notable benefits of AI in data analysis is the automation of time-consuming tasks. AI algorithms can process and analyze data at a speed and scale that human analysts cannot match. This efficiency allows businesses to handle larger datasets and more complex analyses without proportionally increasing their resources. For example, AI can automate the identification of key trends and insights from big data, freeing up human analysts to focus on strategic decision-making and innovation.
3.3 Predictive Analytics
AI excels in predictive analytics by using historical data to forecast future trends and behaviors. This capability is invaluable for businesses looking to anticipate market shifts, customer behaviors, and operational challenges. For instance, retailers can use predictive analytics to forecast inventory needs based on seasonal demand patterns, while financial institutions can predict credit risk and fraud.
3.4 Personalized Customer Experiences
AI-driven data analysis allows businesses to deliver highly personalized customer experiences. By analyzing customer data, including purchase history, browsing behavior, and social media activity, AI can generate tailored recommendations and targeted marketing campaigns. This personalization not only enhances customer satisfaction but also increases engagement and loyalty, driving higher sales and retention rates.
3.5 Cost Reduction
The efficiency gains from AI can lead to significant cost savings. By automating routine data analysis tasks, businesses can reduce labor costs and minimize the potential for human error. Additionally, predictive maintenance enabled by AI can help companies avoid costly downtime by identifying and addressing equipment issues before they result in failures.
3.6 Enhanced Data Accuracy and Quality
AI systems are adept at cleaning and preprocessing data, ensuring higher accuracy and consistency. This capability is crucial for making reliable business decisions. AI can identify and correct errors, fill in missing values, and standardize data formats, which enhances the overall quality of the dataset being analyzed.
3.7 Competitive Advantage
Leveraging AI for data analysis provides a significant competitive edge. Companies that effectively use AI to extract insights from their data can react more quickly to market changes, capitalize on emerging opportunities, and mitigate risks more effectively than their competitors. This agility is a crucial factor in maintaining and enhancing a company’s market position.
3.8 Innovation and Product Development
AI-driven insights can fuel innovation by identifying gaps in the market and areas for improvement in existing products and services. For example, by analyzing customer feedback and usage data, businesses can uncover unmet needs and preferences, guiding the development of new features or entirely new products that better meet customer demands.
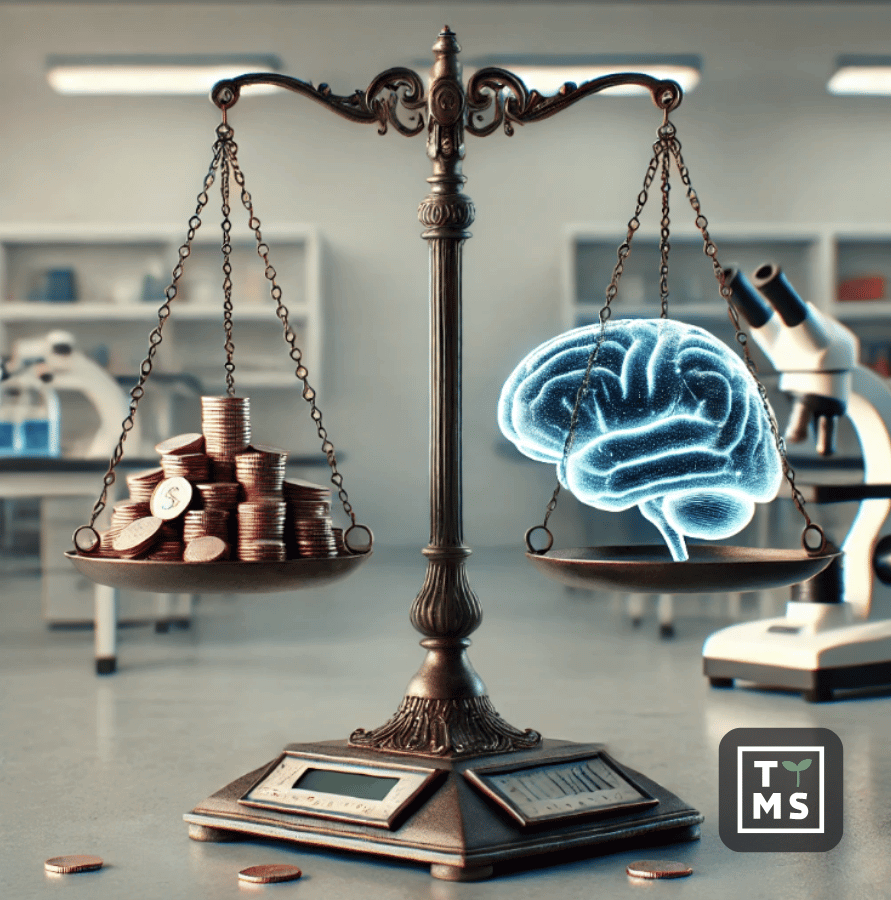
4. The Process of Extracting Actionable Insights
Effectively utilizing AI in data analysis requires several key steps:
- Define Goals and Objectives: Clearly define what the company aims to achieve through data analysis. For example, a company may want to understand why certain products sell better than others or identify new market opportunities.
- Identify Data Sources: Identify both internal and external data sources relevant to the analysis. These might include sales data, customer reviews, social media data, or market reports.
- Ensure Data Quality: Data must be accurate and consistent. This process involves cleaning the data, removing errors, and filling in missing values. For instance, a company might decide to remove duplicate entries in a customer database to ensure consistent analyses.
- Choose Analytical Techniques: Apply appropriate methods such as statistical analysis, data mining, machine learning, or data visualization to uncover patterns and trends. For example, a company might use machine learning algorithms to predict future sales trends based on historical data.
- Extract and Present Insights: Present insights clearly and understandably for all stakeholders. Utilizing data visualization tools can significantly simplify the interpretation of results and their implementation in business strategies.
5. Practical Applications of AI-Driven Insights
AI-driven insights have broad applications across various business functions. In the financial sector, AI can assist in credit risk analysis, fraud detection, and investment portfolio optimization. By analyzing transaction data, AI can identify patterns indicative of potential fraud, allowing for immediate intervention and loss prevention. In credit risk analysis, AI models can assess the likelihood of a borrower defaulting on a loan by evaluating historical data and other relevant factors, thus enabling more accurate lending decisions.
In healthcare, AI can analyze patient data to improve diagnostics and personalize treatment plans. For instance, machine learning algorithms can analyze medical records to identify patients at high risk of certain conditions, enabling early intervention and tailored treatment plans. Additionally, AI can streamline administrative tasks, such as appointment scheduling and patient follow-ups, improving overall efficiency and patient satisfaction.
A retail company can use AI to analyze customer purchasing preferences, enabling personalized offers and increasing sales. By analyzing purchase history and demographic data, AI can help create personalized marketing campaigns that engage customers more effectively and increase loyalty. For example, if data analysis reveals that a segment of customers frequently buys eco-friendly products, the company can target this group with specific promotions for sustainable products, thus boosting engagement and sales.
6. The Future of AI-Driven Insights
The future of AI in data analysis is promising. As AI technologies evolve, they will provide even deeper and more nuanced insights, allowing businesses to better understand their customers and markets. Advanced predictive models, for example, can enable companies to anticipate market changes and adjust strategies in real-time, giving them a significant competitive advantage.
Moreover, the integration of AI with other emerging technologies, such as the Internet of Things (IoT) and blockchain, will further enhance data analysis capabilities. IoT devices can generate vast amounts of data in real-time, while blockchain can ensure data integrity and security. Combining these technologies with AI-driven insights will enable businesses to make even more informed and timely decisions.
7. Conclusion
Integrating AI into data analysis transforms how companies make decisions and develop business strategies. AI-driven insights enable businesses to turn vast amounts of data into actionable strategies that drive growth, optimize operations, and enhance customer experiences. As AI technology continues to evolve, companies that leverage these capabilities will be better positioned to achieve their strategic goals and maintain a competitive edge in the market.
8. How TTMS’s AI Solutions Can Solve Your Business Challenges
TTMS can effectively address business challenges by leveraging AI solutions tailored to enhance operational efficiency and decision-making processes. Their AI services include predictive analytics, machine learning, and automation, enabling businesses to transform raw data into actionable insights. TTMS’s solutions help optimize processes, uncover hidden patterns, and provide strategic guidance, ensuring businesses stay competitive and agile. With their expertise, TTMS supports organizations in deploying AI technologies that drive innovation and growth. Explore more about their AI capabilities at TTMS AI Solutions. If you have any question, you can always contact us!
How has AI improved the speed of data analysis?
AI significantly enhances the speed of data analysis by automating the processing of vast datasets. Traditional methods often require manual intervention and can be time-consuming. AI algorithms, on the other hand, can rapidly process large volumes of data in real-time, enabling businesses to quickly extract insights and respond to market changes or operational needs without delay.
What role does machine learning play in AI-driven data analysis?
Machine learning is a core component of AI-driven data analysis. It involves training algorithms on historical data to recognize patterns and make predictions about future data. Machine learning models can adapt and improve over time, allowing businesses to identify trends, forecast outcomes, and automate decision-making processes based on data-driven insights.
How does AI enhance the accuracy of data analysis?
AI enhances accuracy by using sophisticated algorithms that clean and preprocess data, identifying and correcting errors, filling in missing values, and standardizing data formats. This ensures the data is reliable and consistent, reducing the likelihood of inaccurate insights. Additionally, AI can handle complex datasets that are too large or intricate for traditional methods, providing more precise and actionable results.
Can AI-driven data analysis help in personalizing customer experiences?
Yes, AI-driven data analysis can significantly personalize customer experiences. By analyzing data such as purchase history, browsing behavior, and social media interactions, AI can generate personalized recommendations, targeted marketing campaigns, and customized offers. This level of personalization increases customer satisfaction, engagement, and loyalty, ultimately driving higher sales and retention rates.
How does AI contribute to predictive maintenance in manufacturing?
AI contributes to predictive maintenance by analyzing data from sensors and other monitoring devices on manufacturing equipment. It identifies patterns and anomalies that precede equipment failures, allowing maintenance to be scheduled before a breakdown occurs. This proactive approach reduces downtime, extends the lifespan of machinery, and lowers maintenance costs by preventing unexpected failures.
What are some practical applications of AI-driven insights in finance?
In finance, AI-driven insights can be used for various applications such as fraud detection, credit risk assessment, and investment portfolio optimization. AI analyzes transaction patterns to detect fraudulent activities, enabling immediate intervention. It also evaluates borrowers’ creditworthiness by analyzing historical data and other relevant factors, resulting in more accurate lending decisions. Additionally, AI helps optimize investment portfolios by identifying trends and predicting market movements.
How do businesses ensure the quality of data used for AI analysis?
Ensuring data quality involves several steps: data cleaning to remove errors and duplicates, data integration to combine information from different sources, and data validation to ensure accuracy and consistency. Businesses also employ data governance practices to maintain high standards for data quality. High-quality data is crucial for reliable AI analysis and actionable insights.
How can AI-driven insights improve marketing strategies?
AI-driven insights enhance marketing strategies by enabling more precise customer segmentation and targeting. By analyzing customer data, AI can identify distinct segments based on behaviors, preferences, and demographics. This allows marketers to tailor their campaigns to specific audiences, improving engagement and conversion rates. Additionally, AI can optimize ad spend by predicting the most effective channels and times to reach target customers.
What benefits does AI offer in terms of cost reduction for businesses?
AI offers significant cost reduction benefits by automating routine data analysis tasks, which reduces the need for manual labor and minimizes human error. It also enables predictive maintenance, preventing costly equipment failures and downtime. Furthermore, AI can streamline operations and improve efficiency, leading to overall cost savings in various business processes.
How does AI help in identifying hidden patterns in data?
AI helps identify hidden patterns in data by using advanced techniques such as machine learning, neural networks, and deep learning. These techniques can uncover complex relationships and correlations that might not be visible through traditional analysis methods. By continuously learning from new data, AI can reveal insights that drive strategic decisions and innovation.
Can AI-driven data analysis improve operational efficiency?
Yes, AI-driven data analysis can greatly improve operational efficiency. By automating data processing and analysis, AI frees up human resources to focus on strategic tasks. It also provides real-time insights that help optimize workflows, reduce bottlenecks, and enhance overall productivity. For example, in supply chain management, AI can predict demand fluctuations and optimize inventory levels.
How do AI insights support strategic decision-making?
AI insights support strategic decision-making by providing data-driven evidence that informs business strategies. AI analyzes large datasets to identify trends, opportunities, and risks, offering a comprehensive view of the business landscape. This enables leaders to make informed decisions with greater confidence, reducing reliance on intuition and guesswork, and increasing the likelihood of achieving business objectives.
What is the future of AI-driven data analysis?
The future of AI-driven data analysis is promising, with advancements in AI technologies leading to even deeper and more nuanced insights. Future developments include more sophisticated predictive models, integration with IoT and blockchain for enhanced data collection and security, and real-time analysis capabilities that allow businesses to adapt quickly to changes. These advancements will further empower businesses to make proactive, data-driven decisions.
How does AI enhance the timeliness of business insights?
AI enhances the timeliness of business insights by processing data in real-time and providing immediate analysis. This allows businesses to quickly identify and respond to emerging trends, operational issues, or market changes. For example, real-time analytics can help a retailer adjust pricing strategies on the fly or enable a financial institution to detect and address fraudulent transactions as they occur.
What impact does AI have on customer engagement and loyalty?
AI positively impacts customer engagement and loyalty by delivering highly personalized experiences. By analyzing customer data, AI can create tailored recommendations, targeted marketing, and personalized communication. This approach makes customers feel valued and understood, enhancing their overall experience. Increased satisfaction and engagement lead to stronger loyalty, repeat business, and positive word-of-mouth referrals.