Sort by topics
OpenAI’s Economic Blueprint for Europe – Analysis and Strategic Outlook
In April 2025, OpenAI published its EU Economic Blueprint, a vision of how Europe can harness the potential of artificial intelligence to drive economic growth. The Blueprint was released during a period of intense dialogue between OpenAI and European policymakers — the company’s European tour symbolically began in Warsaw. The document strongly emphasizes the idea of “AI developed in and for Europe”, meaning technology that is created and deployed by Europe, for the benefit of Europe. Below, we present a comprehensive analysis of the Blueprint’s key proposals, projections for how EU decision-makers may respond, Poland’s potential role as a leader in shaping the future of AI, and a critical look at the environmental challenges posed by the planned boom in computational power. Key Proposals in OpenAI’s Economic Blueprint OpenAI presents a range of strategic initiatives designed to accelerate the development of AI within the EU. The most important include: Triple compute capacity by 2030: The proposed AI Compute Scaling Plan aims to increase Europe’s compute infrastructure by at least 300% by 2030. It places particular emphasis on building a geographically distributed network of low-latency data centers optimized for AI, especially the inference phase — the point at which trained models are deployed and generate outputs. The EU has already begun taking steps in this direction, committing approximately €200 billion to digital infrastructure (including supercomputers), and France alone is investing €109 billion in its own national initiatives. OpenAI, however, calls for a significant acceleration of these efforts to ensure Europe does not fall behind global competitors. €1 billion AI Accelerator Fund: The creation of a dedicated €1 billion fund to finance high-impact AI pilot projects with measurable societal or economic value. The AI Accelerator Fund would help demonstrate the real-world benefits of AI in various sectors by supporting early-stage innovations that solve pressing problems. Investment in Talent and Skills: To ensure Europe has the human capital to develop and scale AI, OpenAI proposes the upskilling of 100 million Europeans in AI fundamentals by 2030. The plan includes free online courses available in all EU languages, an “AI Erasmus” program (educational exchanges and fellowships focused on AI), and an expansion of AI Centers of Excellence across Europe. The Blueprint also calls for massive reskilling programs to transition existing workers into AI-relevant roles. The aim is to leverage both Europe’s existing talent (scientists, engineers) and attract global experts — for example, through streamlined visa policies (EU Blue Card reform) and improved working conditions for non-EU AI professionals. Green AI infrastructure: AI development must go hand in hand with clean energy investments. The Blueprint emphasizes the need to build a Green AI Grid — an energy system for powering AI infrastructure based on renewables and next-generation technologies. This includes faster permitting for solar and wind farms, development of nuclear and potentially fusion power, and the modernization of electricity grids. The ultimate goal is for Europe’s AI infrastructure to become climate-neutral, in line with EU environmental ambitions — despite a dramatic increase in energy consumption from data centers. Open Data at the EU Scale: To unlock Europe’s vast data potential, OpenAI proposes the creation of EU AI Data Spaces by 2027 across key sectors (e.g. healthcare, environment, public services). Europe has a rich pool of data, but much of it is fragmented and siloed. OpenAI advocates for secure, privacy-respecting frameworks that enable cross-border and institutional data sharing. These shared data ecosystems would improve access to high-quality training datasets for AI developers and attract investors to locate compute resources and data hubs within Europe. Startup Support and a Unified EU AI Market: To enable startups to scale across the EU, OpenAI recommends establishing a pan-European legal entity for startups by 2026. This legal status would reduce regulatory complexity and allow AI firms to operate seamlessly across all 27 EU member states. The Blueprint also proposes the creation of a European AI Readiness Index — an annual ranking assessing countries’ progress in AI adoption (skills, infrastructure, regulation). By 2027, every EU country should also appoint a national AI Readiness Officer responsible for coordinating national strategy and sharing best practices at the EU level. Regulatory simplification – a lighter AI Act: “A house divided against itself cannot stand” — the Blueprint uses this quote to argue that Europe cannot support AI innovation while simultaneously stifling it with overregulation. OpenAI explicitly addresses the AI Act, the world’s first comprehensive legal framework for AI. While supporting its core objective — ensuring safe and ethical AI — OpenAI warns that overly complex regulations could burden innovators and drive AI research outside Europe. It references a report by Mario Draghi, which warned that excessive regulatory complexity in the EU poses an “existential threat” to its economic future. OpenAI calls for trimming redundant or conflicting laws and harmonizing national approaches across the EU. A coherent and simplified legal framework is crucial if AI companies are to scale efficiently — and if citizens are to benefit from innovation on equal terms throughout the single market. How Will EU Policymakers Respond to OpenAI’s Proposals? Will Europe embrace these ideas? Reactions from EU decision-makers are likely to be mixed. On the one hand, many of the Blueprint’s directions align with existing EU strategies, suggesting a positive reception. On the other hand, certain recommendations — especially around regulation — may provoke caution or even resistance from some lawmakers. Proposals for investment in infrastructure and talent are the most likely to be welcomed. The EU has long recognized that digital transformation and AI are essential for global competitiveness. Several existing initiatives already mirror OpenAI’s suggestions: multibillion-euro infrastructure funds, the EuroHPC project (developing supercomputers for researchers), the European Chips Act (€43 billion for domestic semiconductor production), and the Horizon Europe program funding AI R&D. The call to triple compute capacity by 2030 may be viewed as ambitious but justified — consistent with the EU’s broader aim of achieving technological sovereignty. Owning its own compute resources, data, and energy for AI would reduce Europe’s reliance on third-party providers — something the European Commission already considers a matter of strategic security. Similarly, the idea of a €1 billion AI Accelerator Fund sounds realistic within the EU’s economic scale. For comparison, the Digital Europe Programme has a budget of roughly €7.5 billion, part of which is earmarked for AI. It’s conceivable that the Commission or the European Investment Bank could launch a similar fund, especially under increasing competitive pressure from the U.S. and China. OpenAI’s proposals on skills and talent also resonate with current EU goals. The “Digital Decade” strategy sets targets for 2030 — including 80% of adults having basic digital skills and at least 20 million ICT specialists in the EU. Training 100 million citizens in AI basics complements these ambitions. The EU will likely welcome any initiative that strengthens Europe’s human capital in AI, especially given the widespread shortage of IT professionals. Partnerships with private firms (e.g. for multilingual online AI courses) and youth-oriented campaigns may follow. Ideas like an AI Youth Digital Agency, AI Ambassadors Corps, or an EU AI Awareness Day may seem symbolic, but they are politically neutral and easy to implement — and thus likely to gain traction. Where things may get more complex is regulation, particularly the AI Act. European institutions remain divided. Many lawmakers — especially in the European Parliament and countries like France or Germany — emphasize strong AI regulation, grounded in the precautionary principle and citizen protection. Calls to “streamline” the AI Act may be interpreted as attempts to weaken safeguards. Indeed, in 2023, OpenAI CEO Sam Altman’s warning that overly strict regulation might force OpenAI to withdraw from Europe sparked backlash. EU Commissioner Thierry Breton responded directly, stating: “There is no point in threatening to leave — clear rules do not hinder innovation.” Nevertheless, there are signs of flexibility. The Omnibus Simplification Package — a regulatory streamlining initiative launched by the Commission — reflects growing awareness of overregulation. Some EU countries, particularly those with pro-innovation agendas, may support OpenAI’s call for harmonization and a reduction in red tape. European Commission President Ursula von der Leyen has previously voiced support for creating a unified EU startup market (“EU Inc.”) and reducing legal fragmentation that limits competitiveness. In this context, the proposal for a pan-European startup legal framework could gain political momentum — especially from business-friendly governments and digital economy advocates. In summary, the EU is likely to welcome many of OpenAI’s proposals related to investment, skills, and infrastructure. However, it will likely approach regulatory simplification with more caution. Europe is striving to be both a global leader in responsible AI governance and in AI innovation — a delicate balance. The likeliest scenario is not a radical deregulation, but rather: regulatory sandboxes, tax incentives for low-risk AI projects, and more inclusive policymaking processes involving AI experts and industry stakeholders. OpenAI itself seems to acknowledge this: Altman later stated that “we will comply with whatever rules Europe adopts,” while emphasizing that Europe’s best interest lies in embracing AI adoption quickly — or risk falling behind. Poland as a Potential Leader in AI Transformation OpenAI’s choice to begin promoting the Blueprint in Warsaw was not accidental. Poland is emerging as a key player in the European AI scene — both in terms of talent and digital policymaking. Chris Lehane, OpenAI’s VP of Public Policy, remarked during his Warsaw visit: “Poland is among the global AI leaders,” citing that Poland ranks in the top five European countries for ChatGPT usage — a sign of strong interest in new technologies across society and business. Human capital is Poland’s greatest AI asset. OpenAI noted that “Polish roots run deep in OpenAI’s DNA” — with many co-founders and leading researchers having Polish backgrounds. Indeed, Polish engineers have played a central role in developing some of OpenAI’s most advanced models. Tech giants such as Google, Microsoft, and NVIDIA have R&D centers in Poland, and OpenAI is reportedly considering Warsaw as a location for its first European office — alongside London and Berlin. Sam Altman praised Poland’s “density of talent” as a decisive factor. Poland also holds political leverage. In the first half of 2025, the country holds the EU Council Presidency, allowing it to shape discussions around the EU’s digital agenda. While the AI Act is nearly finalized, Poland can still influence how EU AI strategies are implemented — especially regarding infrastructure, funding, and education programs. During OpenAI’s meetings in Warsaw, the legal environment and opportunities for Polish companies in AI were key themes. Poland appears eager to strike a balance — embracing economic opportunities offered by AI, while also shaping the rules of the game. That positioning may allow Poland to act as a bridge between Big Tech and EU regulators. Poland’s growing AI startup ecosystem and institutional support are also noteworthy. National programs such as IDEAS NCBR (an AI think tank connected to the National Center for Research and Development) and funding from institutions like NCBR and PARP support machine learning innovation. OpenAI’s collaboration with Warsaw’s AI community — including hackathons and research partnerships — reflects growing trust in Poland’s capacity as a development partner. If OpenAI’s Blueprint is adopted, Poland could pilot some of the initiatives. For example, the country could host one of the new AI data centers planned under the 300% compute expansion goal — in line with the geographical decentralization of infrastructure and bringing new investments and jobs. Poland could also become a leader in AI education. Top universities (Warsaw University of Technology, University of Warsaw, AGH, among others) already offer respected programs in AI and data science. With modest government support, Poland could position itself as a European center for AI talent development — perfectly aligned with the Blueprint’s vision of “100 million AI-ready citizens.” Politically, Poland’s voice in the EU — particularly after the 2023 change in government — may now carry more constructive weight. If Poland clearly supports parts of the Blueprint (e.g. calling for faster AI investment at European Council meetings), it could help shape EU conclusions and funding programs. In the past, Poland has taken leadership roles in EU digital policy — such as forming alliances around 5G development or advocating for a common digital market. Now, with the opportunity for a technological leap driven by AI, Poland could become not just a policy recipient, but a co-creator of Europe’s AI future. Compute Growth vs. Sustainability – A Delicate Balance The rapid growth of AI brings not only promise, but also major sustainability challenges. While OpenAI’s Blueprint calls for tripling Europe’s compute capacity, it simultaneously emphasizes the need to ensure sufficient clean energy to support this expansion in line with climate goals. But the scale of projected growth raises tough questions: can European energy systems keep up with AI’s insatiable demand for power? Already, data centers consume a significant portion of global electricity. In 2023, they accounted for approximately 4% of electricity use in the U.S., and with the rise of AI, that figure is expected to triple within five years. Some analysts warn that by 2030–2035, data centers could consume up to 20% of global electricity. Such a spike would pose a serious strain on energy grids and challenge the stability of power supplies. Europe is already in the midst of an energy transition, moving away from fossil fuels and toward renewables — but this transition is complex and time-consuming. If Europe adds a wave of new supercomputing farms and massive server hubs, without matching investments in generation and transmission, it risks blackouts or increased CO₂ emissions, especially if backup comes from coal or gas. To address this, OpenAI proposes an accelerated green transition — fast-track permits for wind and solar farms, investments in nuclear energy, and possibly new sources like fusion — all geared toward meeting AI’s demands. These ideas align with the European Green Deal, but energy infrastructure takes years to build, while compute demand is rising exponentially now. Beyond carbon emissions, other sustainability concerns include water consumption for cooling (a growing issue amid Europe’s recurring droughts), and the environmental footprint of AI hardware production. Chips and GPUs require rare-earth minerals, often sourced from countries with weak labor or environmental standards. An AI hardware boom could increase pressure on these resources — and accelerate global emissions, even if Europe keeps its own relatively low. Additionally, shorter hardware lifecycles — as firms race to adopt ever more powerful AI chips — may worsen the problem of electronic waste, a challenge Europe is already struggling to manage. Still, some solutions could help ease the conflict between growth and sustainability. First, energy efficiency must become a design priority — both at the hardware level (e.g., energy-saving chips, efficient cooling) and software level (e.g., optimizing AI models to require less compute for similar results). Researchers are already developing smaller, more efficient AI models as alternatives to massive, energy-hungry neural networks. Second, smart scheduling and grid management can make a difference — for instance, running AI workloads during off-peak hours or in regions with surplus renewable energy. Third, AI itself can support energy optimization, managing smart grids, forecasting demand, and helping reduce waste — turning AI into both a challenge and a solution. OpenAI’s Blueprint recognizes these trade-offs and calls for AI investments that also accelerate Europe’s green transition. For EU policymakers, this will be non-negotiable: any AI strategy will be judged through the lens of the Green Deal. A 300% compute increase will need to come with clear plans for emissions reduction, energy mix transformation, and possibly green AI standards — such as carbon footprint reporting for large AI projects, or tax incentives for climate-neutral compute centers. Ultimately, responsible AI growth must be both ethical and ecological. If not, AI’s short-term gains could come at the expense of Europe’s long-term sustainability goals. However, AI can also support sustainability — through energy optimization, predictive maintenance, and smart grid management. OpenAI’s emphasis on Green AI by design suggests that AI can be both a challenge and a solution — if developed responsibly. Conclusion OpenAI’s Economic Blueprint offers Europe a strategic vision: a roadmap for becoming a global AI hub through investment, simplification, and sustainable growth. Many of its proposals are compatible with EU priorities — especially in talent development and infrastructure. Regulatory aspects, particularly the push to lighten the AI Act, will provoke more debate but could influence future implementation strategies. Poland, with its tech talent and increasing international visibility, is well-positioned to champion parts of this agenda. By aligning national initiatives with European goals, it could become a key testing ground for OpenAI’s ideas — and a regional leader in responsible AI development. Ultimately, the challenge for the EU will be to combine innovation, regulation, and sustainability into a coherent AI strategy. OpenAI’s Blueprint provides momentum — but Europe must now decide how to channel it into actionable, inclusive, and forward-looking policies that benefit all its citizens. What is the main goal of OpenAI’s Economic Blueprint for Europe? The Blueprint aims to help Europe become a global leader in AI innovation and deployment. It proposes strategic investments in infrastructure, talent development, and regulatory simplification to accelerate economic growth and technological sovereignty while aligning with European values and sustainability goals. What does “inference” mean in the context of AI infrastructure? Inference refers to the process of using a trained AI model to generate predictions, answers, or actions in real-world applications — for example, when ChatGPT replies to a prompt. While training a model is resource-intensive, inference also requires significant compute power, especially at scale. OpenAI emphasizes optimizing infrastructure for inference because it represents the day-to-day, operational side of AI use in businesses and public services. What is meant by a “pan-European legal entity” for startups? OpenAI proposes creating a unified legal status that startups can adopt to operate seamlessly across all EU countries. Currently, launching or expanding an AI business in multiple EU member states involves navigating diverse regulatory, tax, and legal systems. A pan-European legal entity would reduce fragmentation and allow for faster scaling — similar to how the “European Company” (Societas Europaea) structure works in traditional industries. What are “AI Data Spaces” and why are they important? AI Data Spaces are sector-specific digital ecosystems where organizations (public and private) share high-quality datasets under common rules and standards. For example, a European Health Data Space would allow hospitals, research institutions, and companies to securely share anonymized medical data to develop better AI diagnostics. The goal is to overcome data silos while ensuring privacy, interoperability, and legal clarity across borders. What is the concept of “AI Readiness Officers” in the EU context? OpenAI recommends that each EU country appoint an AI Readiness Officer — a high-level coordinator responsible for aligning national AI strategies with EU goals. These officers would track progress, share best practices, and ensure effective implementation of AI-related initiatives across education, infrastructure, and regulation. The role is inspired by similar coordination positions in climate and cybersecurity governance. What can businesses do today to prepare for the AI-driven transformation outlined in the Blueprint? Firms can begin by assessing their current digital maturity and identifying areas where AI can drive efficiency or innovation. Investing in upskilling employees — especially through accessible online AI courses — will help build internal capabilities. Additionally, businesses should monitor developments in EU AI regulation (such as the AI Act), participate in national or sectoral AI pilot programs, and explore partnerships in shared data initiatives. Early engagement with these trends can position companies as frontrunners once EU-wide initiatives, like AI Data Spaces or talent programs, become operational.
ReadMust-Have Features in AI Tools for Training & Development – and Their Benefits in 2025
Not so long ago, employee training meant thick manuals, static presentations, and hours spent in meeting rooms with a trainer. But times have changed. Today, companies aren’t just wondering if they should bring AI into learning and development — they’re asking how to do it smartly. In a fast-moving world where business needs evolve month by month, more organizations are turning to AI to make learning more flexible, targeted, and scalable. Because when training feels relevant, adaptive, and easy to access — it actually works. So here’s the real question: Is your company ready to tap into the potential of AI to help your people grow? 1. The Potential of AI Tools for Training and Development The integration of AI tools for training and development represents a paradigm shift in how organizations approach employee learning. These powerful technologies don’t simply automate existing processes—they fundamentally transform the entire learning ecosystem by introducing capabilities that weren’t previously possible at scale. 1.1 Understanding AI in Learning and Development AI in L&D encompasses a wide range of technologies designed to enhance how knowledge is created, delivered, and absorbed. At its core, AI learning and development tools leverage machine learning algorithms to analyze data patterns, adapt to user behaviors, and deliver increasingly relevant content to learners. These systems continuously improve by processing feedback and interaction data. The strategic implementation of AI tools for learning and development enables organizations to move beyond the traditional one-size-fits-all approach. For instance, natural language processing can power intelligent content recommendations while predictive analytics identifies skill gaps before they impact business outcomes. Computer vision technologies even allow for analyzing learner engagement during video-based training. TTMS has observed that organizations implementing AI L&D tools typically experience 40-60% improvements in training completion rates and knowledge retention. This happens because these systems can identify precisely when learners are struggling and provide targeted interventions before disengagement occurs. Rather than replacing human trainers, AI augments their capabilities, handling repetitive tasks while allowing L&D professionals to focus on high-value strategic work. The most successful implementations start with clear learning objectives and gradually incorporate AI capabilities that directly address specific organizational challenges. 2. Benefits of Integrating AI in Training Programs The strategic implementation of AI in training and development is revolutionizing how organizations approach workforce education. With AI training tools becoming increasingly sophisticated, companies are discovering numerous advantages that extend far beyond simple automation. Let’s explore these benefits in detail. 2.1 Accelerated Content Creation and Translation AI for training and development has dramatically transformed content creation timelines. What previously took weeks of instructional design can now be accomplished in days or even hours. AI training tools can generate initial drafts of training materials, repurpose existing content into different formats, and even create simulations based on company-specific scenarios. Content translation, historically a major bottleneck for global organizations, has been streamlined through AI-powered solutions. These systems can instantly translate training materials into dozens of languages while maintaining contextual accuracy and cultural nuances. TTMS has observed that companies implementing these solutions report 70% faster deployment of global training programs. Organizations leveraging AI employee training for multilingual content have seen particularly impressive results in technical fields where specialized terminology presents unique challenges. The technology continuously improves translations based on industry-specific datasets, ensuring consistency across all learning materials. 2.2 Smarter Content Delivery through AI AI has fundamentally changed how training content reaches learners. Rather than pushing standardized materials to everyone simultaneously, AI systems analyze numerous factors to determine optimal delivery timing, format, and scope for each individual. These systems track learner behavior patterns to identify when employees are most receptive to new information. For example, AI might recognize that certain team members engage better with training during morning hours or after completing specific tasks, and adjust delivery accordingly. The result is significantly higher completion rates and knowledge retention. Content sequencing has also improved through intelligent recommendation engines similar to those used by streaming platforms. By analyzing which learning paths lead to the best outcomes for similar employees, these systems can suggest optimal progression routes through complex training materials. 2.3 Personalized and Adaptive Learning Experiences Perhaps the most transformative benefit of AI in training and development is the ability to truly personalize learning at scale. Traditional approaches forced organizations to choose between customized experiences (expensive) or standardized programs (ineffective). AI eliminates this compromise. Modern AI learning platforms continuously assess learner competencies, adjusting content difficulty, pace, and examples based on individual progress. This dynamic approach ensures employees remain in their optimal learning zone—challenged enough to remain engaged but not overwhelmed to the point of frustration. The customization extends to content formats as well. AI can identify whether a particular employee learns better through visual demonstrations, written instructions, or interactive exercises, then prioritize those formats accordingly. This adaptivity has proven particularly valuable for technical skill development where learning approaches vary significantly among individuals. 2.4 Enhanced Learner Engagement and Interactivity AI employee training systems have transformed passive learning experiences into highly interactive journeys. Gamification elements powered by AI provide meaningful challenges calibrated to each learner’s skill level, while virtual role-playing scenarios adapt in real-time based on learner decisions and responses. These interactive elements generate rich engagement data that AI systems analyze to identify potential knowledge gaps or misconceptions. When patterns emerge suggesting confusion about specific concepts, the system can automatically provide additional explanations or practice opportunities before the learner becomes disengaged. Emotion recognition technologies integrated into video-based learning can even detect when learners appear confused or frustrated, triggering appropriate interventions. This level of responsiveness was previously impossible in traditional training environments. 2.5 Improved Cost and Time Efficiency The economic benefits of integrating AI into training and development are significant. Organizations that adopt these technologies often report 30–50% reductions in training-related costs, while simultaneously enhancing learning outcomes. These savings are driven by factors such as faster content development, reduced reliance on live instruction, and minimized logistical expenses. AI-powered onboarding systems are especially effective in cutting costs, as they can automate up to 80% of standard orientation tasks while delivering personalized experiences to new employees. This approach shortens onboarding timelines and helps new hires become productive more quickly. Efficiency gains also extend to compliance training. AI systems can monitor regulatory updates in real time and automatically adjust learning content, ensuring that employees always have access to up-to-date, accurate information—without the need for constant manual revisions. 2.6 AI-Supported Role Evolution within L&D Far from replacing L&D professionals, AI is elevating their roles to more strategic positions. By automating routine tasks like content updates, assessment grading, and basic question answering, these technologies free L&D teams to focus on high-value activities like learning strategy development and performance consulting. This evolution requires L&D professionals to develop new competencies around AI implementation, ethical considerations, and strategic integration with business objectives. Those embracing this shift are finding themselves in increasingly influential positions within their organizations. 2.7 Automated Workflows and Task Management Administrative efficiency represents another major benefit of AI training tools. These systems can automate enrollment processes, generate completion certificates, send targeted reminders to learners, and maintain comprehensive training records with minimal human intervention. Compliance tracking, historically a labor-intensive process, has been particularly transformed. AI systems can monitor completion rates in real-time, automatically identify non-compliant employees, and generate appropriate notifications. This automation not only reduces administrative burden but also significantly improves compliance rates. 2.8 Advanced Data Analysis and Insights The analytical capabilities of AI in training and development provide unprecedented visibility into learning effectiveness. These systems can correlate training activities with on-the-job performance indicators, helping organizations understand which learning experiences truly impact business outcomes. Predictive analytics tools can identify employees at risk of knowledge gaps before those gaps impact performance. By analyzing patterns across thousands of learner interactions, these systems can recommend targeted interventions that prevent potential issues rather than simply reacting to them. 2.9 Virtual Assistants, Chatbots, and AI Coaching AI-powered learning support systems have transformed how employees access help during the learning process. Virtual assistants can answer questions 24/7, provide clarification on complex concepts, and direct learners to relevant resources. This immediate feedback dramatically improves the learning experience compared to waiting for instructor responses. More sophisticated AI coaching systems can provide personalized guidance throughout the learning journey. These tools analyze numerous factors—from quiz responses to practical application attempts—and offer tailored recommendations for improvement. Some advanced systems can even simulate conversation practice for customer service training or leadership development. 2.10 Innovative Uses of AI in Corporate Settings Beyond traditional implementations, pioneering organizations are leveraging AI learning tools in increasingly creative ways to address complex development challenges. Conflict Resolution and Emotional Intelligence Development Several organizations are deploying sophisticated AI L&D tools to address the challenging area of workplace conflict and emotional intelligence. These systems analyze communication patterns, identify potential conflicts before they escalate, and provide tailored guidance for resolution. More importantly, they help employees develop emotional intelligence skills by providing private feedback on communication styles and suggesting alternative approaches for difficult conversations. Predictive Career Pathing AI learning and development tools are increasingly being used to create highly personalized career development journeys. These systems analyze thousands of career progression patterns within organizations to identify optimal development paths for individual employees based on their unique skills, interests, and performance indicators. By matching employees with precise learning experiences that align with both their aspirations and organizational needs, these systems create unprecedented alignment between individual development and business requirements. Knowledge Retention Reinforcement Addressing the challenge of post-training knowledge decay, several organizations have implemented AI systems that use principles of cognitive science to maximize retention. These platforms analyze individual learning patterns to determine optimal reinforcement timing and deliver micro-learning experiences that significantly improve long-term knowledge retention. Immersive Simulations The most sophisticated AI tools for training and development are creating unprecedented immersive learning experiences. Using technologies like natural language processing, computer vision, and generative AI, these systems create highly realistic scenarios that adapt in real-time to learner decisions. For example, sales professionals can practice complex negotiations with AI-powered virtual customers that demonstrate realistic emotional reactions and unpredictable objections, providing practice opportunities that were previously impossible outside of real customer interactions. These innovative applications demonstrate the expanding possibilities of AI in L&D beyond simple automation or content creation. As these technologies continue to evolve, organizations that strategically implement them are creating significant competitive advantages through superior talent development capabilities. 3. Key Considerations and Future Outlook As organizations increasingly adopt AI in training and development, several critical factors deserve careful attention to ensure successful implementation and sustainable results. Understanding these considerations will help learning leaders navigate the evolving landscape of AI training tools while maximizing their effectiveness. 3.1 Ethical Implementation and Governance Organizations implementing AI for training and development must establish robust ethical frameworks governing these systems. Transparency around how AI evaluates learner performance, makes recommendations, or generates content is essential for maintaining trust. Employees need a clear understanding of when they’re interacting with AI versus human instructors, and how their learning data is being utilized. Data privacy concerns require particular attention when deploying AI employee training systems. Organizations must implement strong safeguards protecting potentially sensitive information gathered during learning activities. This includes establishing clear data retention policies, anonymization practices, and appropriate access controls. TTMS recommends developing specific AI governance committees with cross-functional representation to oversee these critical aspects. Algorithmic bias presents another significant challenge requiring proactive monitoring. Without careful oversight, AI training tools may unintentionally perpetuate existing biases or create new ones. Regular auditing of AI recommendations and outcomes across different demographic groups helps identify potential issues before they impact learning effectiveness or employee advancement opportunities. 3.2 Integration with Existing Systems and Workflows The most successful AI training for employees doesn’t exist in isolation but integrates seamlessly with existing technology ecosystems and workflows. Organizations should prioritize solutions that connect with current learning management systems, talent management platforms, and performance evaluation tools. This integration enables comprehensive tracking of development activities and their impact on business outcomes. Change management represents perhaps the greatest implementation challenge. Even the most sophisticated AI in training and development will fail without effective strategies for user adoption. Organizations should begin with clear communication about how AI will enhance (not replace) human capabilities, followed by phased implementation that demonstrates tangible benefits to both learners and L&D professionals. 3.3 Development of AI-Related Competencies As AI transforms workplace learning, organizations must simultaneously develop AI literacy across their workforce. Employees need sufficient understanding of AI capabilities, limitations, and appropriate uses to effectively collaborate with these systems. This creates an interesting paradox where AI training tools are increasingly used to develop AI-related competencies. L&D professionals require particular attention in upskilling initiatives. Their roles are evolving from content creators to learning experience architects who design effective human-AI collaborative learning environments. Organizations should invest in specialized development for these teams, focusing on competencies like AI implementation oversight, ethical governance, and strategic integration with business objectives. 3.4 Measurement and Continuous Improvement Measuring the effectiveness of AI for training and development requires sophisticated analytics beyond traditional completion metrics. Organizations should establish comprehensive dashboards tracking not only learning outcomes but also their correlation with business performance indicators. This connection between learning activities and business results provides the strongest justification for continued investment in AI-powered learning. Continuous improvement mechanisms should be built into any AI implementation from the beginning. These systems improve through usage, making it essential to establish feedback loops that capture both quantitative performance data and qualitative user experiences. Regular review cycles analyzing this information help organizations continuously refine their approach and maximize return on investment. 3.5 Future Outlook: Emerging Trends and Opportunities Looking ahead, several emerging trends will likely shape the evolution of AI in training and development Multimodal Learning Systems Next-generation AI training tools will seamlessly integrate multiple learning modalities (text, audio, video, simulation, AR/VR) into cohesive experiences that adapt to individual learning preferences. These systems will automatically determine the optimal combination of modalities for each learner and concept, creating unprecedented personalization at scale. Emotion-Aware Learning Advanced AI employee training systems will increasingly incorporate emotional intelligence capabilities, recognizing and responding to learner emotional states. These systems will detect frustration, confusion, boredom, or engagement through multiple inputs (facial expressions, voice tone, interaction patterns) and adjust content delivery accordingly to optimize the learning experience. Collaborative AI Learning Environments Rather than focusing exclusively on individual learning journeys, future AI systems will facilitate collaborative learning by identifying optimal peer pairings, facilitating group problem-solving, and providing targeted interventions to improve team dynamics. These capabilities will be particularly valuable for developing complex collaborative skills that require interaction with others. Knowledge Network Development Future AI in training and development will focus not just on individual competency development but on optimizing organizational knowledge networks. These systems will map knowledge flows across organizations, identify critical knowledge bottlenecks, and recommend strategic interventions to improve collective intelligence rather than just individual capabilities. Human-AI Teaching Partnerships The most sophisticated implementations will create effective partnerships between human instructors and AI systems, with each handling components that leverage their unique strengths. AI might manage personalized practice sessions and basic question answering, while human instructors focus on complex concept explanation, motivation, and addressing unique learning challenges. 3.6 The Path Forward As organizations navigate this rapidly evolving landscape, maintaining balance between technological innovation and human connection will be critical. The most successful implementations of AI in training and development will not simply automate existing approaches but fundamentally reimagine how learning happens within organizations. Organizations should begin with clear learning strategies aligned with business objectives, then thoughtfully implement AI capabilities that directly support these strategies. Starting with well-defined use cases that address specific challenges helps demonstrate value while building organizational capability for more sophisticated applications over time. The future of AI training tools is not about replacing human elements in learning but about amplifying human potential through increasingly intelligent technological partnerships. Organizations that approach implementation with this mindset will create significant competitive advantages through superior talent development capabilities. 4. Turn AI Tools for Training and Development into Real Results — With TTMS by Your Side Implementing AI tools for learning and development requires more than simply purchasing new technology—it demands strategic vision, technical expertise, and change management capabilities. Organizations achieving the greatest success typically partner with experienced implementation experts who understand both the technological and human dimensions of this transformation. 4.1 Why Expert Partnership Matters The landscape of AI L&D tools is evolving rapidly, making it challenging for internal teams to stay current with emerging capabilities and best practices. Working with a specialized partner like TTMS provides access to continuously updated expertise and implementation methodologies refined through multiple successful deployments across industries. Many organizations struggle to connect AI learning initiatives to measurable business outcomes. TTMS approaches implementation with a clear focus on business impact, helping clients define specific success metrics and establish measurement frameworks that demonstrate tangible value. This business-first approach ensures AI in L&D investments generates meaningful returns rather than simply introducing interesting technology. 4.2 TTMS’s Comprehensive Approach to AI Learning Solutions As a global IT company with extensive experience in digital transformation, TTMS brings unique capabilities to AI learning and development implementations. The company’s approach integrates technical expertise with deep understanding of learning methodologies and organizational change management. TTMS offers end-to-end solutions covering the entire AI learning transformation journey: Strategic Assessment and Roadmap Development: Before recommending specific AI tools for training and development, TTMS conducts thorough assessments of current learning ecosystems, organizational readiness, and specific business challenges. This diagnostic approach ensures solutions address genuine needs rather than implementing technology for its own sake. The resulting roadmap provides a clear implementation sequence aligned with organizational priorities and capabilities. Custom AI Learning Solution Development: While many providers offer one-size-fits-all solutions, TTMS specializes in developing customized AI learning platforms tailored to each organization’s unique requirements. As certified partners of technology leaders including Microsoft, Salesforce, and Adobe Experience Manager, TTMS creates solutions that leverage these powerful platforms while addressing specific learning challenges. The company’s E-Learning administration services ensure seamless implementation and ongoing management of AI learning platforms. This includes content migration, user management, and integration with existing HR and talent management systems—critical factors for successful adoption that are often overlooked. Process Automation for Learning Operations: Beyond learner-facing applications, TTMS’s expertise in process automation helps streamline learning operations through. These automation capabilities are particularly valuable for compliance training management, certification tracking, and skills gap analysis. Data Integration and Analytics: The true power of AI in L&D emerges through comprehensive data analytics that connect learning activities to business outcomes. These tools provide unprecedented visibility into learning effectiveness and its impact on operational performance. Additional we offer: E-learning consulting empowers organizations to design scalable, high-impact digital learning solutions tailored to business goals. Consultants assess existing learning ecosystems, recommend optimal LMS or LXP platforms, and define content strategies based on target audience needs and learning analytics. They support the integration of AI, microlearning, gamification, and other modern technologies to boost engagement and retention. This strategic guidance ensures faster implementation, better ROI, and measurable improvements in workforce performance. E-learning development team outsourcing provides companies with immediate access to a skilled, cross-functional team specializing in instructional design, multimedia production, and learning technologies. Instead of building in-house capabilities, organizations can scale faster by leveraging external experts to design, develop, and deliver high-quality digital training. The outsourced team can handle end-to-end development—from needs analysis and storyboard creation to SCORM-compliant modules and platform integration. 4.3 Getting Started with AI Learning Transformation. Where should we begin? For organizations beginning their journey with AI tools for learning and development, TTMS recommends a phased approach: Discovery Workshop: Begin with a focused session exploring current learning challenges, business objectives, and potential AI applications. This workshop helps identify high-value use cases and build internal alignment. Pilot Implementation: Start with a contained implementation addressing a specific learning challenge. This approach demonstrates value quickly while building organizational experience with AI learning tools. Measurement Framework: Establish clear metrics connecting learning activities to business outcomes before expanding implementation. This foundation ensures continued investment generates demonstrable returns. Scaled Deployment: With proven results from the pilot, expand implementation across additional use cases and organizational areas, applying lessons learned to optimize adoption. Continuous Optimization: Implement regular review cycles to assess effectiveness and incorporate emerging AI capabilities that address evolving learning needs. With the pace of change accelerating, organizations must prioritize workforce development to stay relevant and competitive.By working with TTMS to introduce AI-powered tools for training and development, companies can reshape their learning environments, speed up skill-building, and gain a lasting competitive edge through stronger talent capabilities. As AI continues to redefine how we learn at work, the real question isn’t if we should use these technologies — but how to do it right. With TTMS’s deep expertise in both the tech and human sides of learning transformation, your organization can move forward with confidence, turning the potential of AI into real, measurable business impact. Contact us now!
ReadIT Outsourcing Trends for 2025 – Key Developments to Watch
In 2025, IT outsourcing will be a vital component in the strategic development of large enterprises across industries like pharmaceuticals, automotive, education, and finance. Understanding the latest technology outsourcing trends will enable these companies to compete effectively in their respective markets, accelerate innovation, and optimize business operations. Below, we highlight the most important IT outsourcing trends to adopt now. 1. IT Outsourcing Trends – Key Directions for 2025 1.1 Expansion of Strategic Partnerships In 2025, IT outsourcing will evolve beyond mere cost reduction and task delegation. Companies will increasingly form long-term strategic partnerships with IT service providers actively involved in shaping business strategies. Such partnerships allow deeper integration within organizational structures, shared business goals, and exchange of expertise, fostering accelerated innovation and product development. 1.2 Innovation-Oriented Outsourcing By 2025, IT outsourcing trends will heavily emphasize innovation, particularly in the implementation of advanced technologies, including: Artificial Intelligence (AI), for process automation, predictive analytics, and advanced customer relationship management. Blockchain, enhancing process transparency and secure transaction management. Internet of Things (IoT), enabling intelligent asset management, production monitoring, and logistics optimization. Outsourcing providers will serve as technology integrators, helping businesses rapidly implement these technologies tailored specifically to their business needs. 1.3 Nearshoring and Reshoring Gaining Popularity Due to geopolitical instability and the necessity for uninterrupted business continuity, companies will increasingly prefer IT outsourcing providers geographically closer to their primary markets. Nearshoring ensures effective communication, collaboration within the same time zone, and high-quality services due to better understanding of local market specifics and culture. Western European companies, for instance, are increasingly partnering with providers in Poland, the Czech Republic, Romania, and Bulgaria. 1.4 AI-Driven Digital Transformation In 2025, IT outsourcing will center on digital transformation projects leveraging advanced AI solutions, machine learning, and robotic process automation (RPA). Outsourcing providers, such as Transition Technologies MS (TTMS), will support clients in: Big data analytics for informed decision-making. Implementation of intelligent chatbots and customer support systems. Automation of repetitive business processes for enhanced operational efficiency. 1.5 Data Security – A Top Priority Growing cybersecurity threats make data security a decisive factor in choosing outsourcing providers. Companies following IT offshoring trends and outsourcing must comply with stringent regulations (GDPR, NIS2) and offer comprehensive data protection strategies, including: Development of Security Operations Centers (SOC) for monitoring and rapid incident response. Advanced protection against ransomware and phishing attacks. Implementation of Zero Trust systems to minimize unauthorized access risks. 1.6 Hybrid Model and Cloud Computing Companies increasingly adopt hybrid working models, integrating internal teams with external cloud-based specialists. Cloud computing enables easier scalability, faster project deployments, and better IT resource management. Infrastructure as a Service (IaaS) and Platform as a Service (PaaS) are becoming popular, offering flexibility and responsiveness to changing market conditions, as indicated by current trends in outsourcing. 1.7 Sustainability and Responsible Practices Environmental, Social, and Governance (ESG) factors will significantly influence outsourcing decisions. Following outsourcing trends 2025, outsourcing companies will need to demonstrate their ESG strategies and tangible ecological actions. For example, Transition Technologies MS (TTMS) implements a sustainable development policy, focusing on reducing CO2 emissions, efficient energy management, promoting eco-friendly practices among employees, and supporting social and educational environmental initiatives. 2. IT Outsourcing at Transition Technologies MS (TTMS) Transition Technologies MS provides comprehensive IT outsourcing services tailored to clients’ unique needs. Our cooperation models include Staff Augmentation, Team Delivery, and Managed Services. We uphold the highest security standards, confirmed by ISO 27001:2022 and ISO 14001 certifications. We offer flexibility, rapid recruitment of top specialists, and effective team management. We invite businesses looking for sustainable, innovative, and secure IT solutions to partner with us. Contact us now. FAQ What is the difference between nearshoring and reshoring in IT outsourcing? Nearshoring involves partnering with IT providers located geographically close to your business, often in neighboring or regional countries, to ensure better collaboration, cultural alignment, and communication. Reshoring refers to bringing outsourced IT services back to your home country, typically driven by concerns over geopolitical stability, security, or quality control. Why is innovation-oriented outsourcing crucial for enterprises in 2025? Innovation-oriented outsourcing enables companies to rapidly adopt advanced technologies such as Artificial Intelligence, Blockchain, and IoT, without extensive internal investment. Providers specialized in innovation accelerate product development, streamline business processes, and ensure companies remain competitive and responsive to market changes. What is the Zero Trust model in the context of IT outsourcing and why does it matter? The Zero Trust model is a cybersecurity approach that assumes no internal or external user can be trusted by default, requiring continuous authentication and verification for access to resources. In IT outsourcing, this strategy significantly reduces vulnerabilities, prevents unauthorized access, and provides stronger protection against ransomware and phishing attacks. How can outsourcing providers help enterprises achieve sustainability goals? IT outsourcing providers contribute to sustainability goals by implementing environmentally responsible practices such as energy-efficient data centers, reducing carbon footprints, and promoting sustainable operational models. Providers like TTMS incorporate ESG (Environmental, Social, Governance) standards to help companies align their business strategies with global sustainability initiatives. What are strategic partnerships in IT outsourcing, and how do they differ from traditional outsourcing models? Strategic partnerships in IT outsourcing involve deep, long-term collaboration where providers actively participate in shaping business strategies and share common goals with their clients. Unlike traditional outsourcing, which primarily focuses on cost reduction and task delegation, strategic partnerships prioritize joint innovation, shared risks, and integrated planning for mutual business growth.
ReadNot Obvious AI Software for Law Firms – Great Corporate Tools for Legal Teams
In 2025, AI tools are becoming an essential part of modern legal practice. They offer remarkable capabilities, from document analysis to decision-making support. For many solicitors, this is an opportunity to enhance efficiency and service quality. For others, it’s a challenge that demands adaptation to new technologies. Regardless of the perspective, one thing is clear—AI is revolutionising the legal industry. In this article, we explore 10 unconventional AI tools that are shaping the future of law. 1. Introduction to AI in Law Firms. How are law firms and artificial intelligence transforming legal practice in 2025? According to the latest Market.us study, the global AI software market for the legal sector is on a path of dynamic growth. The use of artificial intelligence in law is expanding rapidly, and the numbers confirm this trend. In 2023, the AI software market for law firms in the U.S. alone was valued at $1.5 billion. However, over the next 10 years, its value is projected to rise to $19.3 billion. These optimistic forecasts demonstrate the growing demand for AI-powered tools in the legal industry. By enabling process automation, data analysis, and decision-making support, AI not only enhances law firm efficiency but also allows for more personalised client services. Integrating AI into legal work enables the rapid processing of large volumes of data, such as legal documents, contracts, and court rulings, minimising errors and significantly reducing task completion time. The increasing number of AI vendors specialising in legal technology, along with advancements in machine learning and natural language processing, indicate that artificial intelligence is becoming an integral part of the legal industry’s future. In light of these developments, a strategic approach to AI implementation is crucial to fully leverage its potential while maintaining high ethical standards and legal compliance. 2. Top AI Tools for Law Firms. Understanding the Artificial Intelligence Legal Tech Landscape. To help law firms better understand the potential of artificial intelligence, we have prepared an overview of AI-powered tools already available on the market. These solutions utilise AI in unexpected yet highly effective ways, offering significant benefits to the legal sector. Our selection includes tools that support data analysis, process automation, and innovative applications designed for document management, client service, and legal risk assessment. Our goal is to highlight the wide range of AI-driven possibilities and showcase how different tools can enhance both the efficiency and quality of legal work. 2.1 AI in Legal Practice: A Closer Look at Salesforce Salesforce, best known as a leader in customer relationship management (CRM), has been consistently expanding its AI capabilities over the past few years. In the legal sector, AI-driven tools like the Einstein module open up new opportunities for process automation, data analysis, and workflow optimisation. These innovations enable solicitors to better manage vast amounts of information, which is crucial when handling complex cases and legal document analysis. Salesforce also allows for AI customisation tailored to law firms’ specific needs. These systems can streamline document management, automate routine tasks, and enhance client communication through personalised recommendations. Law firms adopting such solutions gain a competitive edge, improving both operational efficiency and service quality. 2.2 AI-Powered Document Workflow Software for Law Firms – WEBCON and Its Platform Enhancements WEBCON BPS supports the entire contract lifecycle—from creation and negotiation to revision, signing, and archiving. Automating these processes minimises errors and significantly reduces the time needed to finalise agreements, allowing legal professionals to manage documents more efficiently and reduce the risk of losing critical information. Several solutions offered by WEBCON BPS for law firms leverage artificial intelligence (AI). For example, WEBCON BPS integrates AI-powered Optical Character Recognition (OCR) technology, enabling automatic recognition and data extraction from legal documents. This makes document digitisation and data entry faster and more efficient. Thanks to machine learning techniques, WEBCON BPS can detect irregularities in data and analyse information for compliance with historical records, providing users with practical recommendations. For instance, the system can identify an unfamiliar bank account number used by a contractor, potentially signalling a risk or anomaly. 2.3 AI Tools for the Legal Industry That Unlock Endless Possibilities – Power Apps Power Apps is a platform within the Microsoft Power Platform ecosystem, designed to enable businesses to create applications without advanced coding skills. As a low-code/no-code tool, it allows users with minimal programming knowledge to design applications using an intuitive graphical interface. Power Apps seamlessly integrates with multiple systems and services, including Microsoft 365, Dynamics 365, Azure, as well as external databases and cloud services. This flexibility enables organisations to develop customised applications that automate processes, manage data, and enhance daily workflows. AI-powered solutions in Power Apps are particularly effective due to their integration with Microsoft services, such as Azure AI, Power Automate, and Power BI. Here are some examples of how AI enhances Power Apps for legal firms: 2.3.1 Automated Legal Document Analysis (AI Builder) Power Apps integrated with AI Builder can utilise AI models to automatically read and analyse documents, such as contracts, invoices, and legal regulations. 2.3.2 Predictions and Recommendations (AI Builder) AI-driven predictive models can analyse client data, forecast case outcomes, and suggest the best course of action for legal professionals. 2.3.3 AI-Powered Chatbots (Copilot Studio). A most wanted law AI tool. AI-driven chatbots can answer client enquiries, direct them to the appropriate departments, and assist with online form submissions. 2.3.4 Sentiment and Text Analysis (Azure OpenAI Service) By integrating with Azure OpenAI, Power Apps can analyse the sentiment of emails, client feedback, and legal texts, helping law firms better understand client interactions. 2.3.5 Automated Report Generation (Power BI + AI) With Power BI, law firms can generate dynamic reports based on analysed data, enabling them to: Track case progress Forecast team workload for future periods Evaluate employee efficiency AI capabilities in Power BI also allow for natural language queries, enabling users to “converse with data” and extract insights without manually creating reports. 2.3.6 Image and Text Recognition (AI Builder) AI Builder tools can process images and text, such as recognising scanned documents and converting them into digital data for further analysis. 2.3.7 Personalised and Optimised Client Service AI in Power Apps analyses client data, contact history, and preferences to deliver personalised experiences, including: Automated reminders for deadlines Recommendations for additional services based on client data analysis By leveraging AI-driven automation and intelligent data processing, Power Apps helps law firms streamline operations, improve efficiency, and deliver enhanced legal services. 2.4 AI for Legal Professionals – Microsoft Power BI Microsoft Power BI is an incredibly versatile tool that can significantly support law firms by providing advanced data analysis and intuitive information visualisation. Highly valued in the corporate world, Power BI has been helping managers make data-driven decisions for years, thanks to its flexibility and adaptability to diverse business needs. One of its key features is the ability to create interactive reports that analyse data from multiple integrated sources. This allows law firms to monitor key performance indicators, identify trends, and make informed decisions faster and more effectively. Power BI can be used in various ways to enhance legal operations. It enables case analysis and performance tracking by creating reports and dashboards that help monitor case progress, track team workload, and assess key performance indicators. This allows firms to detect delays, compare workload distribution among solicitors, and optimise resource management. It also supports financial monitoring by analysing costs, revenue, court fees, invoices, and case budgets. With these insights, law firms can track expenses, identify the most profitable clients and services, and create revenue forecasts, helping them make strategic business decisions. Another important application is client analysis. By examining demographic data, collaboration history, and feedback, law firms can better understand client needs, personalise their services, and identify new business opportunities. Contract and risk management is also improved with Power BI, as it enables efficient monitoring of contract deadlines, identification of risky clauses, and tracking negotiation statuses, minimising various legal and financial risks. Additionally, it helps ensure more precise scheduling and increases operational efficiency. Power BI also offers seamless integration with other systems, such as CRM, ERP, document management tools, and email platforms. Consolidating data from multiple sources in one place makes analysis and management easier. Moreover, its predictive analytics capabilities allow law firms to assess risks related to case outcomes, financial challenges, or operational issues. By using historical data, firms can identify potential risks, improve decision-making, and prepare for possible challenges. 2.5 AI-Powered Tools for Lawyers – Adobe Experience Manager (AEM) Adobe Experience Manager (AEM) integrates advanced AI-powered solutions to streamline the creation, management, and optimisation of digital content. These AI-driven features enable law firms to enhance their content strategies and improve client engagement. One of the most valuable functions of AEM is AI-generated content variations. The platform uses generative AI to create multiple versions of legal content based on given prompts. The “Generate Variations” feature allows for the rapid development of personalised content, accelerating marketing processes and increasing audience engagement. Law firms can use this capability to efficiently produce different versions of legal articles, newsletters, and service descriptions, adapting them to various client groups and legal requirements. Another key feature of AEM is its ability to personalise content. By integrating with Adobe Target, the platform analyses user behaviour and delivers relevant content in real-time. This ensures that each visitor receives materials that are best suited to their needs, making communication more effective. For example, clients searching for information about family law will be presented with articles on divorce, custody, and parental rights, increasing the relevance of the content provided. AEM also integrates with Adobe Experience Platform, offering an AI assistant that helps users analyse data, automate tasks, and generate content. Law firms can use this tool to gain insights into client behaviour, predict their needs, and automate marketing activities. This enables more effective management of legal marketing campaigns and a better alignment of services with client expectations. By leveraging AI-powered solutions like Microsoft Power BI and Adobe Experience Manager, law firms can enhance efficiency, improve decision-making, and optimise client communication. These technologies not only support internal processes but also enable firms to reach potential clients more effectively, ensuring personalised interactions and streamlined operations. In an increasingly digital legal landscape, AI is becoming an essential tool for staying competitive and delivering high-quality legal services. 2.6 Is ChatGPT the Most Popular AI Technology in Law and Why? ChatGPT, based on advanced artificial intelligence algorithms, opens up new opportunities for law firms to optimise processes and enhance service quality. With its ability to deeply understand context and generate human-like responses, ChatGPT stands out among other tools available on the market, making it particularly useful in the dynamic and demanding legal industry. However, it is difficult to say that ChatGPT is the most popular AI technology in law. While its popularity is growing rapidly, its applications differ from more specialised AI tools designed specifically for the legal sector. 2.6.1 Legal Document Creation and Editing ChatGPT can generate initial drafts of contracts, legal pleadings, and other legal documents, speeding up the document creation process. This allows solicitors to focus on substantive analysis while saving time on routine tasks. 2.6.2 Analysis and Processing of Large Data Sets The model can quickly search and analyse extensive databases, identifying key information, precedents, or court rulings. This enables more effective case strategy preparation and a better understanding of the legal context. 2.6.3 Automation of Routine Tasks ChatGPT can automate repetitive tasks, such as drafting standard responses to client enquiries or generating reports. This helps optimise team workflow and reduce administrative workload. 2.6.4 Support for Legal Research With access to a vast knowledge base, ChatGPT can provide information on applicable laws, legal interpretations, and recent legislative changes, assisting solicitors in their daily work. 2.6.5 Improving Client Communication The model can generate clear and understandable explanations of complex legal issues, improving communication with clients and increasing their satisfaction with legal services. 2.6.7 Education and Training by Legal AI Tools ChatGPT can serve as a tool for creating training materials or simulating legal cases, supporting the professional development of law firm employees. 2.6.8 Personalisation of Legal Services By analysing client data and preferences, ChatGPT can help develop personalised offers and legal strategies tailored to individual needs. It is important to note that using ChatGPT also comes with challenges, such as ensuring data confidentiality and verifying generated content for compliance with current legal regulations. Therefore, integrating this tool into law firm operations should be carefully considered and adapted to the firm’s specific needs. 2.7 Does Microsoft Offer the Best AI Tools for the Legal Industry? Microsoft provides a wide range of AI tools that can be highly useful for the legal industry, but whether they are the “best” depends on the specific needs of a law firm and how they compare to competing solutions. In addition to the previously mentioned Power Apps and Power BI, Microsoft has been heavily investing in the development of another key tool: Microsoft Copilot. Microsoft Copilot is a suite of AI-powered tools integrated with Microsoft products such as Microsoft 365, Dynamics 365, and Azure. Once integrated, Copilot works seamlessly across applications like Word, Excel, PowerPoint, Outlook, and Teams, enabling automation of various tasks. For example, in Word, Copilot can generate draft documents based on input data or transform text into different writing styles. In Excel, it can analyse large datasets, suggest appropriate charts if needed, and process natural language queries, such as “Show me data from the last three months.” This makes Copilot an ideal AI tool for automating routine tasks within Microsoft software. But what specific benefits can it bring to law firms? The answer is quite clear. Copilot enables rapid searching and analysis of large sets of legal documents, identifying key clauses and potential risks. This allows solicitors to focus on the more complex aspects of their cases while saving time on routine tasks. With its integration into Microsoft 365 applications, such as Word and PowerPoint, Copilot supports the creation of initial drafts for contracts, legal pleadings, and presentations. It can also suggest both stylistic and substantive edits, streamlining the document review process. Copilot is also a valuable tool for quickly locating legal precedents, court rulings, and legislative changes, providing up-to-date information that is essential for legal proceedings. Moreover, its integration with tools like Power Automate allows law firms to automate routine tasks, such as managing deadlines, tracking case progress, and generating reports, ultimately improving operational efficiency. Another noteworthy feature of Copilot is its ability to generate meeting summaries and draft responses to client enquiries, enhancing communication with both clients and business partners. By implementing Microsoft Copilot, law firms can not only increase productivity but also improve the quality of their services, adapting to the rapidly evolving legal landscape. Microsoft also places strong emphasis on data security. All data processed by Copilot complies with Microsoft’s privacy policies and is fully protected against unauthorised access. 3. Evaluating AI Software for Law Firms: A Strategic Approach Selecting the right AI software for a law firm requires a strategic approach that considers the organisation’s specific needs and objectives. A key part of this process is identifying the areas where AI can deliver the greatest benefits, such as automating routine tasks, analysing legal documents, or optimising case management processes. Once these areas are defined, a thorough assessment of available solutions must be conducted, focusing on functionality, compliance with legal regulations, data security, and integration with existing systems. Another crucial step is evaluating implementation costs in relation to potential savings and efficiency improvements. Finally, choosing a provider who not only delivers the right technology but also offers implementation support and team training is essential. Taking a strategic approach to evaluating AI software enables law firms to maximise the value of their investment while minimising the risks associated with adopting new technologies. 4. Effective Implementation of AI Software in Legal Practices Successfully implementing AI software in law firms requires a well-thought-out approach that combines both the technical aspects of deployment and the necessary adjustments to workflow within the team. The first step is to thoroughly understand the firm’s needs and identify the areas where artificial intelligence can bring the most value, such as automating repetitive tasks, analysing legal documents, or predicting case outcomes. Selecting the right software is a crucial stage in this process. The chosen solution should not only meet current needs but also be flexible and scalable to accommodate future technological advancements. It is equally important to ensure that the selected tool complies with existing legal regulations, such as GDPR, and adheres to high standards of data security, which is critical when handling sensitive client information. Once the software has been selected, it is essential to provide proper training for the team, allowing solicitors and administrative staff to integrate the new tool into their daily workflows effectively. Appointing technology leaders within the firm can also be beneficial, as they can assist colleagues in adapting to and fully leveraging the capabilities of AI solutions. AI software for law firms should also be continuously monitored and evaluated to measure its effectiveness. Analysing results helps identify areas for further optimisation and improvements that can enhance the software’s performance and value to the firm. In this way, artificial intelligence becomes an integral part of the firm’s strategy, contributing to higher-quality legal services and strengthening its competitive edge. 5. How Can TTMS Support the Implementation of AI Solutions Tailored to Your Needs? TTMS (Transition Technologies Managed Services) is a trusted partner in the implementation of advanced technologies, offering comprehensive support in developing and deploying AI solutions tailored to the unique needs of law firms. Through its AI4Legal offering, TTMS enables law firms to fully harness the potential of artificial intelligence in key areas such as document automation, legal data analysis, and case management optimisation. TTMS experts combine deep technological knowledge with extensive experience in legal sector implementations, ensuring the development of customised solutions that are both highly efficient and fully compliant with legal regulations. The implementation process includes an in-depth analysis of client requirements, the design and deployment of best legal AI tools, and comprehensive training for legal professionals to ensure a smooth and effective transition to modern technologies. Moreover, TTMS continues to support its clients post-implementation by providing maintenance and ongoing development services, enabling law firms to continuously improve their operational efficiency. TTMS is the ideal partner for law firms looking to invest in innovation while maintaining the highest standards of security and service quality. Contact us now! Check our related case studies: Case Study – AI Implementation for Court Document Analysis Using AI in Corporate Training Development: Case Study AI-Driven SEO Meta Optimisation in AEM: Stäubli Case Study Didn’t find the answers to your questions in this article? Check out the FAQ section. What is AI-powered legal software? AI-powered legal software refers to technological solutions designed to assist solicitors in document analysis, process automation, and decision-making. It utilises advanced AI algorithms, such as natural language processing (NLP) and machine learning, to quickly search databases, identify key information, and suggest solutions. These tools can draft contracts, assess legal risks, and provide predictions on case outcomes. By reducing the time and costs associated with routine tasks, AI-driven legal software enhances law firm productivity. It is particularly useful in due diligence analysis, contract management, and regulatory compliance. What are the key characteristics of AI-powered legal technology? Legal technology powered by artificial intelligence is characterised by the automation of processes such as contract analysis and creation, legal research, and case management. By leveraging natural language processing (NLP), AI can quickly scan legal documents, identify key clauses, and suggest modifications, improving efficiency and accuracy in legal workflows. What challenges will law firms and AI in the legal sector face in 2025? Law firms and the use of AI in law will face significant challenges in 2025. Among the most pressing issues are client data protection, compliance with AI-related legal regulations, and liability for errors generated by AI algorithms. Additionally, the adoption of AI in law firms requires investment in technology infrastructure and employee training. There are also concerns related to ethics and the potential replacement of human roles by technology. However, firms that successfully integrate AI into their operations can gain a competitive advantage through process automation and increased efficiency. How popular is artificial intelligence in law in the USA compared to Europe? AI in law is gaining traction in both the USA and Europe, but adoption is generally faster in the USA. American law firms are more open to AI-driven automation, especially for legal research, document analysis, and contract management. Europe, while embracing AI, faces stricter regulations, such as GDPR, which impact AI implementation. The USA has a stronger start-up ecosystem for legal AI, whereas Europe focuses more on compliance and ethical concerns. Despite differences, both regions recognise AI’s potential in improving efficiency and reducing costs. Is legal AI technology the same in the USA and Europe, or are there significant differences in its development and regulation? Legal AI technology is similar in both regions in terms of capabilities, but there are key differences in regulation and adoption speed. The USA has a more flexible regulatory environment, allowing for faster innovation and AI integration in legal services. Europe, on the other hand, has stricter data protection laws, such as GDPR, which influence how AI can be used in legal practices. Additionally, some European countries have specific guidelines on AI ethics and transparency, impacting AI deployment in law firms. These regulatory differences mean that legal AI adoption in Europe often requires additional compliance measures. How much of a competitive advantage does artificial intelligence legal software give a law firm in winning a case? AI legal software provides a significant advantage by improving research speed, document review, and case prediction. AI tools can analyse vast amounts of legal data in seconds, identifying relevant precedents and potential risks more efficiently than humans. However, AI alone does not guarantee winning a case—it serves as a support tool that enhances decision-making rather than replacing legal expertise. The firms that integrate AI with experienced legal professionals gain the most competitive edge. Ultimately, AI boosts efficiency and accuracy, but legal strategy and human judgement remain crucial. Is the use of artificial intelligence in law in court proceedings accepted by the justice system? The acceptance of artificial intelligence in law in court proceedings varies depending on the jurisdiction. In the USA, AI is increasingly used for legal research, case analysis, and document automation, but courts remain cautious about AI-generated legal arguments and decisions. In Europe, AI tools are used primarily for administrative and analytical support, while direct AI involvement in judicial decision-making is heavily regulated. Many legal systems require human oversight to ensure fairness, accuracy, and accountability in legal proceedings. While AI is a valuable tool, its role in court is still limited to supporting, not replacing, human judgement. Is it possible to quickly gain the skills needed to effectively use law firm AI software? Yes, many law firm AI tools are designed to be user-friendly and do not require advanced technical knowledge. Training programmes and onboarding sessions provided by software vendors help legal professionals adapt quickly. However, mastering AI-assisted legal research and document automation may take time, depending on the complexity of the software. Continuous learning is essential, as AI capabilities evolve and new features are introduced. While basic use can be learnt quickly, maximising AI’s potential requires ongoing training and adaptation.
ReadChatGPT 4.5 – What’s New? Practical Examples and Applications
OpenAI has released a long-awaited update to its popular language model, ChatGPT 4.5, also known as Orion. GPT-4.5 is OpenAI’s largest and most advanced language model to date. The new version of the model brings significant improvements in creativity, emotional intelligence, information accuracy, and context understanding. So let’s take a closer look at it. 1. Why does GPT-4.5 understand the world better? GPT-4.5 better “understands the world” thanks to several key improvements in the way it was designed and trained: Advanced unsupervised learning scaling: The model was trained on massive text datasets without direct supervision, allowing it to “autonomously” discover linguistic structures, word relationships, and contexts. This gives GPT-4.5 a more intuitive grasp of linguistic nuances, which translates into a better understanding of content and user intent. Intensive training on Microsoft Azure AI supercomputers: The use of powerful computing resources has enabled the processing of much larger amounts of data and the use of more complex model architectures. Such mass-scale training allows for: Expanding the knowledge base, which results in a deeper understanding of reality. Improving pattern recognition mechanisms, which helps minimize errors such as so-called “hallucinations” – i.e. generating inaccurate or false information. Better combining unsupervised learning with reasoning: Although GPT-4.5 relies primarily on unsupervised learning, modern techniques also allow it to effectively use elements of reasoning. This allows the model to not only analyze data, but also interpret and apply it in context, resulting in more precise and accurate answers. Optimization of training architecture and techniques: Improvements in the model architecture and the use of advanced training techniques (such as a combination of traditional training and reinforcement learning) allow for better detection of subtle relationships and contexts. As a result, GPT-4.5 is better able to “understand” both the literal and figurative meaning of texts, making it more flexible and reliable in interpreting human speech. In short, by combining extensive, scalable training on massive datasets, a modern architecture, and powerful computing resources, GPT-4.5 gains the ability to understand the world more deeply and intuitively. This approach enables it to provide more accurate, consistent, and empathetic responses, which is crucial in everyday interactions with users. 2. Does GPT-4.5 actually generate more creative responses? Early tests show that interactions with GPT-4.5 are much more natural, fluid, and creative. The model generates higher quality, more engaging, and vivid statements, better tailored to the user’s intent. Let’s compare the capabilities of Orion and the previous version of the model using 3 prompts: Prompt 1: “Describe briefly but creatively an autumn afternoon.” GPT-4: “The autumn afternoon is cool and leaves are falling from the trees.” GPT-4.5: “The autumn afternoon smells of wet earth and warm tea, while the sun streaks golden through the reddening leaves. The wind whispers softly through the branches, carrying memories of summer past.” Prompt 2: “Describe briefly but creatively a morning walk in a city park.” GPT-4: “A morning walk in a city park is a peaceful moment when nature comes to life and people enjoy the fresh air.” GPT-4.5: “A morning walk in a city park is like immersing yourself in living poetry – fog gently drifts between the trees and the first rays of sunlight break through the leaves, waking up the city to the joyful symphony of the day.” Prompt 3: “Create a creative vision of the future where nature and technology coexist.” GPT-4: “In the future, nature and technology will coexist, creating a harmonious reality where people enjoy advanced technology while caring for the environment.” GPT-4.5: “Imagine a world where glass skyscrapers blend into lush, green forests, and digital trees grow alongside real ones. Interactive gardens pulsate with energy, and the symbiosis of technology and nature creates a poetic mosaic of a new era.” Of particular note is the direct address to the recipient (“Imagine…”) and… the response time. Generating the text (which, by the way, is longer) took noticeably less time in the case of Chat GPT 4.5. 3. How does GPT-4.5 deal with emotions? GPT-4.5 shows significant improvement in emotional intelligence by using Reinforcement Learning with Human Feedback (RLHF). This is a training technique in which the AI model learns not only from raw data, but also from feedback from humans evaluating its responses. Experts analyze the different variants of the responses generated by the model and choose the ones that are most relevant, empathetic, and in line with the user’s intention. Based on this, a special reward model is created that teaches GPT-4.5 what responses it should prefer to be more useful, natural, and supportive in the conversation. This allows the model to better interpret emotions, avoid inappropriate responses, and provide more empathetic and personalized responses. New training techniques increase the model’s ability to pick up on subtle emotional cues and intentions, which translates into more empathetic, natural, and situationally appropriate responses. The model not only understands words, but also the emotional context, making it a better conversation partner. 3.1 How does GPT-4.5 interpret emotions? GPT-4.5 is trained on human interactions and expert ratings, allowing it to: Recognize tone of voice – it can distinguish between a happy tone and a sad or sarcastic one. Adjust response style – when a user is expressing frustration, the model will respond with a more calm, supportive tone, while in a happy context it may use more enthusiastic language. Better response to sensitive topics – with RLHF, the model avoids trivializing difficult emotions and instead offers more supportive and empathetic responses. 3.2 Empathy in practice Through RLHF, GPT-4.5 learned to adjust his responses to sound more natural and appropriate to the situation: Example: User prompt: “I feel down today.” GPT-4 (without RLHF): “I’m sorry to hear that. I hope it gets better.” GPT-4.5 (with RLHF): “I’m sorry you feel that way. Do you want to talk about it? Maybe I can help, suggest something to cheer you up or take your mind off things?” We see that the GPT-4.5 response is more caring, attuned to the user’s emotions, and offers the opportunity to continue the conversation in a supportive way. 3.3 Fewer “emotional” errors and more naturalness Thanks to RLHF, the model avoids misinterpreting emotions that could lead to inappropriate reactions. GPT-4.5’s responses are more natural, fluid, and tailored to the user’s needs, making the conversation with AI more human. In short, GPT-4.5 not only understands emotions better, but also responds to them appropriately, making it a more effective tool in interactions that require empathy and sensitivity. 4. Does GPT-4.5 make fewer errors? GPT-4.5 has significantly reduced the number of so-called “hallucinations”—false or fictitious information that AI models generate when they don’t have enough data to provide an accurate answer. Hallucinations can include false facts, misinterpretations, or even completely made-up content that sounds plausible at first glance. To mitigate this problem, OpenAI has made several significant improvements to the new version of the model. GPT-4.5 has been trained on an even larger and more diverse dataset, allowing it to better understand reality and fill in missing information with guesswork less often. At the same time, the new model architecture improves the way it processes information and recognizes patterns, which increases the consistency and precision of the answers it generates. In addition, the use of reinforcement learning based on human feedback (RLHF) plays an important role. Thanks to this technique, experts evaluate the model’s responses and indicate which are more accurate and consistent with reality, which allows GPT-4.5 to distinguish true information from false information more effectively. As a result, the model is less likely to provide non-existent facts as certainties. Uncertainty detection mechanisms have also been improved, thanks to which GPT-4.5 better recognizes situations in which it lacks data. Instead of providing false information with confidence, it uses more cautious formulations, suggesting the user to check reliable sources. Another new feature is greater flexibility in updating knowledge through integration with dynamic data sources and the ability to adjust the model to specific needs through fine-tuning. Thanks to this, GPT-4.5 reduces the risk of providing outdated information and better adapts to real, changing conditions. While no AI is completely free from errors, the improvements in this version make the model much more precise, logical, and aware of its own limitations, making its answers more reliable and useful in everyday use. 5. Is GPT-4.5 the basis for future reasoning models? Chat GPT-4.5, also known as Orion, is a significant step forward in the development of language models, focusing on advanced unsupervised learning. OpenAI plans for such models to become a solid foundation for future systems developing advanced logical and technical reasoning capabilities. In the future, it is expected to integrate unsupervised learning methods with reasoning techniques, which will increase the versatility of the AI. In terms of further plans, OpenAI is working on the GPT-5 model, which is expected to introduce significant improvements. According to the information, GPT-5, also known as Orion, has been in development for 18 months, but has encountered delays and high costs associated with training the model. Challenges include a lack of sufficient and high-quality data and competition for computing resources. To overcome these limitations, OpenAI is hiring experts to generate new data and is exploring the possibility of using synthetic data created by existing AI models, although this is associated with certain risks. Despite these challenges, Microsoft is preparing to host the upcoming GPT-4.5 and GPT-5 models on its servers. GPT-5, integrating more OpenAI technologies, including the new o3 reasoning model, is expected around the end of May. The goal is to create a more advanced AI system, approaching artificial general intelligence (AGI). It also plans to unify the o-series and GPT models to improve user experience by eliminating the need to choose the right model for a specific task. The introduction of GPT-5 also aims to simplify OpenAI’s product offering. Currently, users have to choose between different models, which can be complicated. The new system is supposed to automatically analyze content and choose the best model, increasing usability in different contexts. Importantly, GPT-5 is to be available in an “unrestricted way” as a free version, which could increase its accessibility to a wider range of users. 6. How does GPT-4.5 ensure user security? Security remains a key aspect of all OpenAI models, and GPT-4.5 is designed to minimize the risk of erroneous, malicious, or inappropriate responses. The model has undergone extensive testing against a comprehensive Preparedness Framework that includes analyzing potential threats, mitigating the risk of generating malicious content, and implementing measures to prevent misuse. Using advanced supervision, the model is constantly monitored for correctness and security. One key element of ensuring security is the combination of traditional supervised training (SFT) and reinforcement learning based on human feedback (RLHF). This allows the model to better understand the context and intent of the user, allowing it to avoid inappropriate content and adapt responses in a more ethical and consistent way. Human judgment also helps eliminate biases and reduce the risk of generating content that could be disinformative, aggressive, or dangerous. Additionally, GPT-4.5 has been equipped with uncertainty detection mechanisms that allow it to better recognize situations where it does not have enough data to provide a confident response. Rather than providing misinformation, the model is more likely to suggest fact-checking with credible sources or being cautious with its claims. Another important aspect of security is implementing content filters and abuse mitigation systems that help detect and block potentially harmful queries. 7. Who can use GPT-4.5 and what are the costs? Thanks to its numerous improvements, GPT-4.5 is widely used in many areas, where its ability to generate natural, contextually tailored and precise responses can significantly improve various processes. In customer service, the model works as a tool supporting interactions with users, providing more natural, empathetic and personalized responses. Thanks to a better understanding of the context and intentions of customers, it can help solve problems, answer queries more precisely and effectively establish dialogue, which increases the level of user satisfaction. Integration of GPT-4.5 with chatbots and automated service systems allows for faster and more accurate responses, while reducing the burden on support staff. In marketing and copywriting: the model is a powerful tool for generating attractive advertising content, social media posts, slogans or even comprehensive blog articles. Thanks to the ability to create creative and engaging texts, it can support marketers in creating promotional campaigns tailored to different groups of recipients. What’s more, GPT-4.5 can analyze data and adapt its message to the brand’s tone and style, which allows for consistent communication and better targeting of customer needs. In psychological support: the model can act as a first line of emotional support, offering users support in difficult moments. Thanks to increased emotional intelligence and the ability to recognize subtle emotional cues, GPT-4.5 can adjust the tone of speech to the situation, providing more empathetic and caring responses. Although it does not replace professional therapy, it can act as an assistant supporting people looking for comfort, motivation or strategies to cope with everyday emotional challenges. In education: the model works perfectly as a tool supporting the learning process. Thanks to its ability to precisely answer questions of pupils and students, it can help in acquiring knowledge, explaining complex issues in an accessible way and providing interactive educational materials. It can also support teachers in creating tests, teaching materials or lesson plans, as well as help students learn foreign languages through interactive conversations and error correction. Thanks to its advanced natural language processing mechanisms, GPT-4.5 can also be used in many other areas, such as data analysis, scientific research, software development, and even supporting business decision-making. Its versatility and improved information processing capabilities make it an extremely useful tool in the modern digital world. 8. ChatGPT 4.5 – A game-changing AI? GPT-4.5 is a significant step forward in the development of artificial intelligence, significantly improving the quality of interactions between users and the AI model. With better understanding of context, greater creativity, more empathetic responses and error reduction, the new version of the model becomes an even more versatile tool. It is used in customer service, marketing, education, data analysis and even emotional support, making it an invaluable support for business and everyday users. Artificial intelligence is not only the technology of the future, but a tool that is already revolutionizing the way we work and communicate. At Transition Technologies MS, we specialize in providing advanced AI solutions for business that support process automation, operation optimization and efficiency improvement in various industries. Contact us! What is Orion, and how does it relate to ChatGPT 4.5? Orion is the internal codename for ChatGPT 4.5, used by OpenAI to differentiate this upgraded model from previous versions. While the name “ChatGPT 4.5” is used publicly, “Orion” is often mentioned in internal and technical discussions. This version brings significant improvements in creativity, emotional intelligence, accuracy, and contextual understanding, making interactions more fluid and natural. What is unsupervised learning, and how does GPT-4.5 use it? Unsupervised learning is a machine learning technique where a model learns patterns, relationships, and structures from data without explicit human-labeled annotations. In GPT-4.5, unsupervised learning enables the model to absorb vast amounts of text data, recognize language patterns, and generate human-like responses without requiring direct supervision. This approach allows the AI to refine its understanding of language, context, and nuance, improving its ability to generate coherent and contextually relevant answers. What is RLHF, and why is it important for ChatGPT 4.5? Reinforcement Learning from Human Feedback (RLHF) is a training method that improves AI models by incorporating human feedback. In this process, human evaluators assess AI-generated responses, ranking them based on quality, accuracy, and ethical considerations. The model then learns from this feedback through reinforcement learning, adjusting its responses to align better with human expectations. RLHF in GPT-4.5 enhances its emotional intelligence, reduces misinformation, and ensures that generated responses are more aligned with user intent, making interactions more natural and empathetic. What is the Preparedness Framework, and how does it ensure safety in GPT-4.5? The Preparedness Framework is a structured safety and risk assessment approach used by OpenAI to evaluate AI models before deployment. It focuses on identifying potential risks such as misinformation, bias, security vulnerabilities, and harmful content generation. By implementing this framework, OpenAI ensures that GPT-4.5 meets safety standards, minimizes harmful outputs, and adheres to ethical guidelines. The model undergoes extensive testing to refine its responses and reduce risks associated with AI-driven conversations. What is SFT, and how does it contribute to model improvement? Supervised Fine-Tuning (SFT) is a training technique where AI models are improved using high-quality, human-annotated datasets. Unlike unsupervised learning, where the model learns from raw data without guidance, SFT involves explicitly labeled examples to correct and refine the model’s outputs. For GPT-4.5, SFT helps improve factual accuracy, coherence, and ethical alignment by reinforcing desired behaviors and eliminating biases. This fine-tuning process is essential for ensuring that the model generates reliable, safe, and contextually appropriate responses.
ReadBoost Operational Efficiency with AI – Speed up Your Business
In today’s fast-paced business environment, artificial intelligence (AI) is a game-changer for operational efficiency. Companies leveraging AI streamline processes, reduce costs, and improve performance, gaining a competitive edge. AI goes beyond automation—it provides data-driven insights that enhance decision-making and precision. This article explores practical AI applications across industries and strategies to help your business achieve greater efficiency. 1. How AI Transforms Operational Efficiency 1.1 What Is Operational Efficiency? Operational efficiency means delivering products or services in the most cost-effective way while maintaining quality. Businesses that optimize processes experience lower costs, faster workflows, and higher customer satisfaction. However, traditional methods often fall short in managing complex operations. 1.2 AI’s Evolving Role in Operations Management AI in operations management is no longer just about automating tasks—it’s revolutionizing efficiency. By analyzing vast datasets, AI identifies optimization opportunities beyond human capabilities. AI operational efficiency enhances decision-making, reduces errors, and streamlines resource allocation. Companies leveraging artificial intelligence efficiency gain a competitive advantage through predictive maintenance, intelligent supply chain management, and automated workflows. AI in operations adapts over time, continuously improving efficiency. Organizations that strategically implement AI for operations can unlock new business models, redefining industry standards. 2. Key Benefits of AI in Boosting Operational Efficiency 2.1 Process Automation: Reducing Errors and Increasing Productivity AI operational efficiency allows businesses to automate repetitive tasks, reducing human error and freeing employees for strategic work. Efficiency AI solutions improve accuracy, ensuring consistent performance without fatigue. Many industries report reduced production time and improved workflows with AI in operations management. 2.1.1 Better Decision-Making with AI Efficiency AI-driven analytics transform vast data into actionable insights, enhancing decision-making. AI for operations enables predictive analytics, helping businesses optimize inventory, resource allocation, and maintenance. Artificial intelligence in operations management ensures organizations shift from reactive to proactive strategies, increasing efficiency and performance. 2.1.2 Cost Reduction and Revenue Growth AI operational efficiency drives cost savings through process optimization, waste reduction, and predictive maintenance. AI in operations minimizes downtime and extends asset lifespan. Artificial intelligence efficiency also enhances revenue generation by improving customer experiences and accelerating product development. By leveraging AI and efficiency strategies, companies streamline operations, reduce costs, and gain a competitive edge. 2.2 Practical Applications of AI Across Industries 2.2.1 AI in Healthcare: Enhancing Patient Care and Operational Efficiency AI in operations management is transforming healthcare by optimizing both clinical and administrative processes. AI-driven diagnostics, such as IBM Watson Health, analyze vast medical datasets to improve disease detection and treatment recommendations. AI operational efficiency enhances hospital management by predicting patient admissions, optimizing bed allocation, and automating scheduling. Efficiency AI solutions also streamline administrative workflows, reducing paperwork and freeing medical staff for patient care. AI for operations in early disease detection identifies patterns in medical images, allowing for faster and more accurate diagnoses. Artificial intelligence in operations management not only improves patient outcomes but also reduces operational costs, making healthcare more efficient. 2.2.2 AI in Energy: Optimizing Grid Management and Predictive Maintenance The energy sector benefits significantly from AI operational efficiency, particularly in grid optimization and predictive maintenance. AI in operations enhances energy distribution, reduces downtime, and improves demand forecasting. AI-driven predictive analytics help energy companies anticipate equipment failures, extending the lifespan of critical infrastructure and minimizing costly repairs. TTMS has developed scalable AI efficiency solutions that consolidate multiple systems for a leading energy provider. By implementing artificial intelligence in operations management, companies in the energy sector can reduce operational costs, improve resource management, and enhance sustainability efforts. 2.2.3 AI for Legal: Automating Document Analysis and Risk Assessment AI in operations is revolutionizing legal services by automating time-consuming processes like contract review and risk assessment. AI-powered tools analyze thousands of legal documents in seconds, improving accuracy and reducing workload. Efficiency AI applications in law firms streamline case research, identify precedents, and predict litigation outcomes. AI operational efficiency enhances compliance monitoring, ensuring firms stay updated with regulatory changes. With artificial intelligence in operations management, legal teams improve productivity, minimize errors, and focus on higher-value tasks. 2.2.4 AI in Manufacturing: Quality Control and Predictive Maintenance Manufacturing is one of the most AI-driven industries, leveraging artificial intelligence efficiency to enhance production quality and reduce downtime. AI-powered predictive maintenance analyzes sensor data to prevent unexpected equipment failures, increasing productivity and reducing costs. Computer vision systems also play a key role in AI in operations by detecting defects with higher accuracy than manual inspections, improving product quality while minimizing waste. AI operational efficiency allows manufacturers to optimize supply chains and streamline production workflows. 2.2.5 AI in Retail: Personalized Customer Experiences and Supply Chain Optimization AI in operations management has reshaped retail by optimizing both customer interactions and logistics. AI-driven demand forecasting predicts inventory needs, reducing stock shortages and excess supply. AI operational efficiency enhances pricing strategies with real-time adjustments based on demand trends. On the customer-facing side, artificial intelligence in operations management personalizes shopping experiences with recommendation engines, increasing conversions and customer satisfaction. AI-powered chatbots further enhance efficiency AI solutions in customer support, resolving inquiries instantly. 2.2.6 AI in Finance: Fraud Detection and Risk Management Financial institutions leverage AI in operations to detect fraud and improve risk assessment. AI-driven fraud detection systems analyze thousands of transactions per second, identifying suspicious patterns and preventing fraudulent activities in real-time. AI operational efficiency also improves credit risk assessments by analyzing both traditional and alternative data sources, ensuring better lending decisions. Artificial intelligence efficiency in financial operations streamlines compliance monitoring and regulatory reporting. 2.2.7 AI in Telecom: Network Optimization and Event Forecasting AI for operations in telecom focuses on network optimization and congestion management. AI-driven systems analyze historical network data, event calendars, and real-time demand to prevent service disruptions. By leveraging AI operational efficiency, telecom providers can allocate network resources dynamically, ensuring uninterrupted service during peak demand. Artificial intelligence in operations management enhances customer satisfaction while optimizing infrastructure investments. 3. AI Technologies Driving Operational Transformation 3.1 Machine Learning: Smarter, Adaptive Decision-Making Machine learning is the backbone of AI in operations, enabling systems to continuously learn and improve. Unlike static automation, machine learning-driven AI operational efficiency enhances decision-making by analyzing vast datasets and detecting hidden patterns. AI for operations leverages predictive analytics to optimize maintenance schedules, detect anomalies, and refine resource allocation. Deep learning, a subset of machine learning, expands artificial intelligence efficiency by processing unstructured data, such as images and speech, further enhancing operational insights. 3.2 Natural Language Processing (NLP): Automating Communication and Data Analysis AI efficiency solutions powered by NLP transform how businesses handle communication and documentation. AI in operations management enables chatbots and virtual assistants to handle customer inquiries 24/7, reducing response times and improving service quality. NLP also streamlines internal operations by analyzing and summarizing vast amounts of text data, such as contracts, emails, and reports. AI operational efficiency in this area eliminates manual review, reducing processing times and improving accuracy. 3.3 Robotic Process Automation (RPA): Automating Routine Tasks with AI AI-enhanced RPA automates repetitive, rules-based tasks with precision, freeing employees for higher-value work. AI in operations allows businesses to integrate automation with machine learning, enabling systems to adapt to process variations rather than following rigid scripts. Efficiency AI applications in RPA are widely used for data entry, invoice processing, and workflow automation. AI operational efficiency ensures near-perfect accuracy and faster execution, reducing costs and minimizing errors. 3.4 Computer Vision: Enhancing Quality Control and Security AI operational efficiency extends beyond digital processes through computer vision, which interprets visual data for real-world applications. AI in operations management improves manufacturing quality control, detecting product defects more accurately than human inspectors. In security and logistics, AI for operations enhances monitoring by analyzing surveillance footage in real-time, identifying safety hazards and unauthorized access. Artificial intelligence efficiency in these applications improves safety, compliance, and operational performance. 4. Concluding Insights: Embracing AI for Sustainable Operational Success 4.1 Key Strategies for Business Leaders To boost operational efficiency with AI, businesses must take a strategic approach. Successful AI adoption starts with identifying critical inefficiencies and selecting AI solutions that deliver measurable value. AI operational efficiency depends on high-quality data—without a solid data infrastructure, even advanced AI systems will underperform. Cross-functional collaboration is crucial. AI in operations management works best when technical teams, business leaders, and end-users align their goals. Training employees to work alongside AI enhances adoption and maximizes returns. AI operational efficiency should complement human expertise, not replace it. Governance and ethical oversight are equally important. Organizations must ensure AI in operations adheres to regulatory standards while maintaining transparency and accountability. A well-structured AI strategy prevents risks while driving long-term benefits. 4.2 Long-Term Benefits of AI Adoption AI efficiency compounds over time, delivering enhanced operational efficiency through continuous learning and adaptation. Businesses leveraging AI for operations gain agility, allowing them to respond faster to market changes and customer needs. AI operational efficiency also improves decision-making by refining analytics models, leading to smarter, data-driven strategies. Additionally, artificial intelligence efficiency in customer interactions increases satisfaction and retention, driving revenue growth. Companies that integrate AI in operations management effectively will achieve a sustainable competitive edge. The key is ongoing refinement—organizations must continuously optimize their AI strategies to stay ahead in an increasingly AI-driven business landscape. 5. How TTMS can help you with implementing AI for Boosting Operational Efficiency? 5.1 How TTMS Can Help You Implement AI for Boosting Operational Efficiency At TTMS, we specialize in delivering AI-powered solutions that enhance operational efficiency across industries. Our expertise in AI in operations management allows businesses to streamline workflows, reduce costs, and gain a competitive edge. 5.1.1 Tailored AI Strategies for Your Business We start with an in-depth analysis of your current processes to identify key areas where AI operational efficiency can deliver measurable improvements. Our experts develop customized AI solutions that integrate seamlessly with your existing infrastructure, ensuring minimal disruption and maximum impact. 5.1.2 Seamless AI Implementation and Integration TTMS specializes in deploying AI for operations with a focus on scalability and flexibility. Whether it’s process automation, predictive analytics, or AI-driven decision-making, we provide end-to-end implementation tailored to your needs. As partners of leading technology providers such as AEM, Salesforce, and Microsoft, we ensure that our solutions align with industry best practices. 5.1.3 AI-Powered Process Automation and Business Intelligence We help businesses automate repetitive tasks. Our Business Intelligence (BI) solutions, powered by tools like Snowflake DWH and Power BI, transform raw data into actionable insights, supporting enhanced operational efficiency and data-driven decision-making. 5.1.4 Long-Term Support and Continuous Optimization AI is not a one-time implementation—it requires ongoing optimization. TTMS has extensive experience in building long-term partnerships, continuously supporting clients in optimizing and evolving their AI solutions. Our dedicated teams ensure that your AI-driven processes remain efficient, adaptable, and aligned with your business goals as they grow and change. By partnering with TTMS, you gain access to a team that understands AI in operations and is committed to delivering artificial intelligence efficiency that drives sustainable success. 5.2 Let’s Talk About AI for Your Business Looking to boost operational efficiency with AI? Get in touch with our experts at TTMS to explore how AI can transform your business operations. We’ll help you identify opportunities, implement tailored solutions, and support you every step of the way. FAQ How does AI improve efficiency? AI boosts operational efficiency by automating repetitive tasks, reducing human errors, and optimizing decision-making. AI-driven analytics process vast amounts of data, uncovering patterns that improve workflows, resource allocation, and predictive maintenance. With AI in operations, businesses shift from reactive to proactive strategies, minimizing downtime and maximizing productivity. What is operational efficiency in business? Operational efficiency is the ability to deliver products or services with minimal waste while maintaining quality. AI operational efficiency enhances process automation, resource utilization, and decision-making, helping businesses reduce costs and improve performance. How does AI increase efficiency? AI increases efficiency by automating workflows, analyzing data for better decision-making, and predicting outcomes to optimize operations. AI for operations reduces errors, speeds up processes, and ensures optimal resource allocation, resulting in cost savings and improved performance. How can artificial intelligence help managers enhance business operations? AI in operations management helps managers make data-driven decisions, optimize resource allocation, and improve forecasting. AI operational efficiency automates routine tasks, allowing managers to focus on strategic initiatives while enhancing overall business agility and performance.
ReadThe world’s largest corporations have trusted us

We hereby declare that Transition Technologies MS provides IT services on time, with high quality and in accordance with the signed agreement. We recommend TTMS as a trustworthy and reliable provider of Salesforce IT services.

TTMS has really helped us thorough the years in the field of configuration and management of protection relays with the use of various technologies. I do confirm, that the services provided by TTMS are implemented in a timely manner, in accordance with the agreement and duly.
Ready to take your business to the next level?
Let’s talk about how TTMS can help.
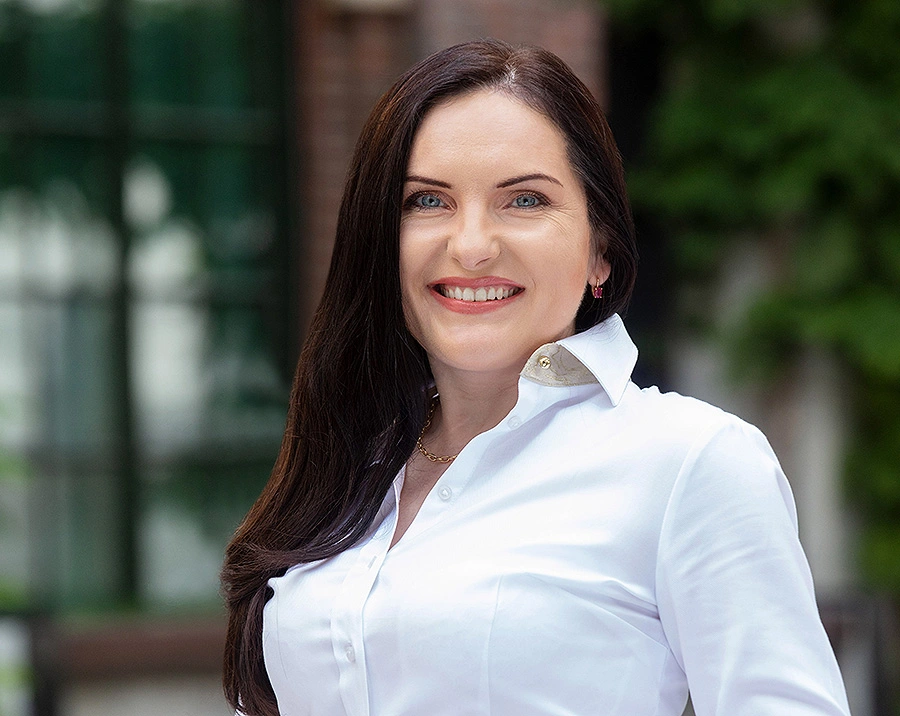