Sort by topics
IT Outsourcing Trends for 2025 – Key Developments to Watch
In 2025, IT outsourcing will be a vital component in the strategic development of large enterprises across industries like pharmaceuticals, automotive, education, and finance. Understanding the latest technology outsourcing trends will enable these companies to compete effectively in their respective markets, accelerate innovation, and optimize business operations. Below, we highlight the most important IT outsourcing trends to adopt now. 1. IT Outsourcing Trends – Key Directions for 2025 1.1 Expansion of Strategic Partnerships In 2025, IT outsourcing will evolve beyond mere cost reduction and task delegation. Companies will increasingly form long-term strategic partnerships with IT service providers actively involved in shaping business strategies. Such partnerships allow deeper integration within organizational structures, shared business goals, and exchange of expertise, fostering accelerated innovation and product development. 1.2 Innovation-Oriented Outsourcing By 2025, IT outsourcing trends will heavily emphasize innovation, particularly in the implementation of advanced technologies, including: Artificial Intelligence (AI), for process automation, predictive analytics, and advanced customer relationship management. Blockchain, enhancing process transparency and secure transaction management. Internet of Things (IoT), enabling intelligent asset management, production monitoring, and logistics optimization. Outsourcing providers will serve as technology integrators, helping businesses rapidly implement these technologies tailored specifically to their business needs. 1.3 Nearshoring and Reshoring Gaining Popularity Due to geopolitical instability and the necessity for uninterrupted business continuity, companies will increasingly prefer IT outsourcing providers geographically closer to their primary markets. Nearshoring ensures effective communication, collaboration within the same time zone, and high-quality services due to better understanding of local market specifics and culture. Western European companies, for instance, are increasingly partnering with providers in Poland, the Czech Republic, Romania, and Bulgaria. 1.4 AI-Driven Digital Transformation In 2025, IT outsourcing will center on digital transformation projects leveraging advanced AI solutions, machine learning, and robotic process automation (RPA). Outsourcing providers, such as Transition Technologies MS (TTMS), will support clients in: Big data analytics for informed decision-making. Implementation of intelligent chatbots and customer support systems. Automation of repetitive business processes for enhanced operational efficiency. 1.5 Data Security – A Top Priority Growing cybersecurity threats make data security a decisive factor in choosing outsourcing providers. Companies following IT offshoring trends and outsourcing must comply with stringent regulations (GDPR, NIS2) and offer comprehensive data protection strategies, including: Development of Security Operations Centers (SOC) for monitoring and rapid incident response. Advanced protection against ransomware and phishing attacks. Implementation of Zero Trust systems to minimize unauthorized access risks. 1.6 Hybrid Model and Cloud Computing Companies increasingly adopt hybrid working models, integrating internal teams with external cloud-based specialists. Cloud computing enables easier scalability, faster project deployments, and better IT resource management. Infrastructure as a Service (IaaS) and Platform as a Service (PaaS) are becoming popular, offering flexibility and responsiveness to changing market conditions, as indicated by current trends in outsourcing. 1.7 Sustainability and Responsible Practices Environmental, Social, and Governance (ESG) factors will significantly influence outsourcing decisions. Following outsourcing trends 2025, outsourcing companies will need to demonstrate their ESG strategies and tangible ecological actions. For example, Transition Technologies MS (TTMS) implements a sustainable development policy, focusing on reducing CO2 emissions, efficient energy management, promoting eco-friendly practices among employees, and supporting social and educational environmental initiatives. 2. IT Outsourcing at Transition Technologies MS (TTMS) Transition Technologies MS provides comprehensive IT outsourcing services tailored to clients’ unique needs. Our cooperation models include Staff Augmentation, Team Delivery, and Managed Services. We uphold the highest security standards, confirmed by ISO 27001:2022 and ISO 14001 certifications. We offer flexibility, rapid recruitment of top specialists, and effective team management. We invite businesses looking for sustainable, innovative, and secure IT solutions to partner with us. Contact us now. FAQ What is the difference between nearshoring and reshoring in IT outsourcing? Nearshoring involves partnering with IT providers located geographically close to your business, often in neighboring or regional countries, to ensure better collaboration, cultural alignment, and communication. Reshoring refers to bringing outsourced IT services back to your home country, typically driven by concerns over geopolitical stability, security, or quality control. Why is innovation-oriented outsourcing crucial for enterprises in 2025? Innovation-oriented outsourcing enables companies to rapidly adopt advanced technologies such as Artificial Intelligence, Blockchain, and IoT, without extensive internal investment. Providers specialized in innovation accelerate product development, streamline business processes, and ensure companies remain competitive and responsive to market changes. What is the Zero Trust model in the context of IT outsourcing and why does it matter? The Zero Trust model is a cybersecurity approach that assumes no internal or external user can be trusted by default, requiring continuous authentication and verification for access to resources. In IT outsourcing, this strategy significantly reduces vulnerabilities, prevents unauthorized access, and provides stronger protection against ransomware and phishing attacks. How can outsourcing providers help enterprises achieve sustainability goals? IT outsourcing providers contribute to sustainability goals by implementing environmentally responsible practices such as energy-efficient data centers, reducing carbon footprints, and promoting sustainable operational models. Providers like TTMS incorporate ESG (Environmental, Social, Governance) standards to help companies align their business strategies with global sustainability initiatives. What are strategic partnerships in IT outsourcing, and how do they differ from traditional outsourcing models? Strategic partnerships in IT outsourcing involve deep, long-term collaboration where providers actively participate in shaping business strategies and share common goals with their clients. Unlike traditional outsourcing, which primarily focuses on cost reduction and task delegation, strategic partnerships prioritize joint innovation, shared risks, and integrated planning for mutual business growth.
ReadNot Obvious AI Software for Law Firms – Great Corporate Tools for Legal Teams
In 2025, AI tools are becoming an essential part of modern legal practice. They offer remarkable capabilities, from document analysis to decision-making support. For many solicitors, this is an opportunity to enhance efficiency and service quality. For others, it’s a challenge that demands adaptation to new technologies. Regardless of the perspective, one thing is clear—AI is revolutionising the legal industry. In this article, we explore 10 unconventional AI tools that are shaping the future of law. 1. Introduction to AI in Law Firms. How are law firms and artificial intelligence transforming legal practice in 2025? According to the latest Market.us study, the global AI software market for the legal sector is on a path of dynamic growth. The use of artificial intelligence in law is expanding rapidly, and the numbers confirm this trend. In 2023, the AI software market for law firms in the U.S. alone was valued at $1.5 billion. However, over the next 10 years, its value is projected to rise to $19.3 billion. These optimistic forecasts demonstrate the growing demand for AI-powered tools in the legal industry. By enabling process automation, data analysis, and decision-making support, AI not only enhances law firm efficiency but also allows for more personalised client services. Integrating AI into legal work enables the rapid processing of large volumes of data, such as legal documents, contracts, and court rulings, minimising errors and significantly reducing task completion time. The increasing number of AI vendors specialising in legal technology, along with advancements in machine learning and natural language processing, indicate that artificial intelligence is becoming an integral part of the legal industry’s future. In light of these developments, a strategic approach to AI implementation is crucial to fully leverage its potential while maintaining high ethical standards and legal compliance. 2. Top AI Tools for Law Firms. Understanding the Artificial Intelligence Legal Tech Landscape. To help law firms better understand the potential of artificial intelligence, we have prepared an overview of AI-powered tools already available on the market. These solutions utilise AI in unexpected yet highly effective ways, offering significant benefits to the legal sector. Our selection includes tools that support data analysis, process automation, and innovative applications designed for document management, client service, and legal risk assessment. Our goal is to highlight the wide range of AI-driven possibilities and showcase how different tools can enhance both the efficiency and quality of legal work. 2.1 AI in Legal Practice: A Closer Look at Salesforce Salesforce, best known as a leader in customer relationship management (CRM), has been consistently expanding its AI capabilities over the past few years. In the legal sector, AI-driven tools like the Einstein module open up new opportunities for process automation, data analysis, and workflow optimisation. These innovations enable solicitors to better manage vast amounts of information, which is crucial when handling complex cases and legal document analysis. Salesforce also allows for AI customisation tailored to law firms’ specific needs. These systems can streamline document management, automate routine tasks, and enhance client communication through personalised recommendations. Law firms adopting such solutions gain a competitive edge, improving both operational efficiency and service quality. 2.2 AI-Powered Document Workflow Software for Law Firms – WEBCON and Its Platform Enhancements WEBCON BPS supports the entire contract lifecycle—from creation and negotiation to revision, signing, and archiving. Automating these processes minimises errors and significantly reduces the time needed to finalise agreements, allowing legal professionals to manage documents more efficiently and reduce the risk of losing critical information. Several solutions offered by WEBCON BPS for law firms leverage artificial intelligence (AI). For example, WEBCON BPS integrates AI-powered Optical Character Recognition (OCR) technology, enabling automatic recognition and data extraction from legal documents. This makes document digitisation and data entry faster and more efficient. Thanks to machine learning techniques, WEBCON BPS can detect irregularities in data and analyse information for compliance with historical records, providing users with practical recommendations. For instance, the system can identify an unfamiliar bank account number used by a contractor, potentially signalling a risk or anomaly. 2.3 AI Tools for the Legal Industry That Unlock Endless Possibilities – Power Apps Power Apps is a platform within the Microsoft Power Platform ecosystem, designed to enable businesses to create applications without advanced coding skills. As a low-code/no-code tool, it allows users with minimal programming knowledge to design applications using an intuitive graphical interface. Power Apps seamlessly integrates with multiple systems and services, including Microsoft 365, Dynamics 365, Azure, as well as external databases and cloud services. This flexibility enables organisations to develop customised applications that automate processes, manage data, and enhance daily workflows. AI-powered solutions in Power Apps are particularly effective due to their integration with Microsoft services, such as Azure AI, Power Automate, and Power BI. Here are some examples of how AI enhances Power Apps for legal firms: 2.3.1 Automated Legal Document Analysis (AI Builder) Power Apps integrated with AI Builder can utilise AI models to automatically read and analyse documents, such as contracts, invoices, and legal regulations. 2.3.2 Predictions and Recommendations (AI Builder) AI-driven predictive models can analyse client data, forecast case outcomes, and suggest the best course of action for legal professionals. 2.3.3 AI-Powered Chatbots (Copilot Studio). A most wanted law AI tool. AI-driven chatbots can answer client enquiries, direct them to the appropriate departments, and assist with online form submissions. 2.3.4 Sentiment and Text Analysis (Azure OpenAI Service) By integrating with Azure OpenAI, Power Apps can analyse the sentiment of emails, client feedback, and legal texts, helping law firms better understand client interactions. 2.3.5 Automated Report Generation (Power BI + AI) With Power BI, law firms can generate dynamic reports based on analysed data, enabling them to: Track case progress Forecast team workload for future periods Evaluate employee efficiency AI capabilities in Power BI also allow for natural language queries, enabling users to “converse with data” and extract insights without manually creating reports. 2.3.6 Image and Text Recognition (AI Builder) AI Builder tools can process images and text, such as recognising scanned documents and converting them into digital data for further analysis. 2.3.7 Personalised and Optimised Client Service AI in Power Apps analyses client data, contact history, and preferences to deliver personalised experiences, including: Automated reminders for deadlines Recommendations for additional services based on client data analysis By leveraging AI-driven automation and intelligent data processing, Power Apps helps law firms streamline operations, improve efficiency, and deliver enhanced legal services. 2.4 AI for Legal Professionals – Microsoft Power BI Microsoft Power BI is an incredibly versatile tool that can significantly support law firms by providing advanced data analysis and intuitive information visualisation. Highly valued in the corporate world, Power BI has been helping managers make data-driven decisions for years, thanks to its flexibility and adaptability to diverse business needs. One of its key features is the ability to create interactive reports that analyse data from multiple integrated sources. This allows law firms to monitor key performance indicators, identify trends, and make informed decisions faster and more effectively. Power BI can be used in various ways to enhance legal operations. It enables case analysis and performance tracking by creating reports and dashboards that help monitor case progress, track team workload, and assess key performance indicators. This allows firms to detect delays, compare workload distribution among solicitors, and optimise resource management. It also supports financial monitoring by analysing costs, revenue, court fees, invoices, and case budgets. With these insights, law firms can track expenses, identify the most profitable clients and services, and create revenue forecasts, helping them make strategic business decisions. Another important application is client analysis. By examining demographic data, collaboration history, and feedback, law firms can better understand client needs, personalise their services, and identify new business opportunities. Contract and risk management is also improved with Power BI, as it enables efficient monitoring of contract deadlines, identification of risky clauses, and tracking negotiation statuses, minimising various legal and financial risks. Additionally, it helps ensure more precise scheduling and increases operational efficiency. Power BI also offers seamless integration with other systems, such as CRM, ERP, document management tools, and email platforms. Consolidating data from multiple sources in one place makes analysis and management easier. Moreover, its predictive analytics capabilities allow law firms to assess risks related to case outcomes, financial challenges, or operational issues. By using historical data, firms can identify potential risks, improve decision-making, and prepare for possible challenges. 2.5 AI-Powered Tools for Lawyers – Adobe Experience Manager (AEM) Adobe Experience Manager (AEM) integrates advanced AI-powered solutions to streamline the creation, management, and optimisation of digital content. These AI-driven features enable law firms to enhance their content strategies and improve client engagement. One of the most valuable functions of AEM is AI-generated content variations. The platform uses generative AI to create multiple versions of legal content based on given prompts. The “Generate Variations” feature allows for the rapid development of personalised content, accelerating marketing processes and increasing audience engagement. Law firms can use this capability to efficiently produce different versions of legal articles, newsletters, and service descriptions, adapting them to various client groups and legal requirements. Another key feature of AEM is its ability to personalise content. By integrating with Adobe Target, the platform analyses user behaviour and delivers relevant content in real-time. This ensures that each visitor receives materials that are best suited to their needs, making communication more effective. For example, clients searching for information about family law will be presented with articles on divorce, custody, and parental rights, increasing the relevance of the content provided. AEM also integrates with Adobe Experience Platform, offering an AI assistant that helps users analyse data, automate tasks, and generate content. Law firms can use this tool to gain insights into client behaviour, predict their needs, and automate marketing activities. This enables more effective management of legal marketing campaigns and a better alignment of services with client expectations. By leveraging AI-powered solutions like Microsoft Power BI and Adobe Experience Manager, law firms can enhance efficiency, improve decision-making, and optimise client communication. These technologies not only support internal processes but also enable firms to reach potential clients more effectively, ensuring personalised interactions and streamlined operations. In an increasingly digital legal landscape, AI is becoming an essential tool for staying competitive and delivering high-quality legal services. 2.6 Is ChatGPT the Most Popular AI Technology in Law and Why? ChatGPT, based on advanced artificial intelligence algorithms, opens up new opportunities for law firms to optimise processes and enhance service quality. With its ability to deeply understand context and generate human-like responses, ChatGPT stands out among other tools available on the market, making it particularly useful in the dynamic and demanding legal industry. However, it is difficult to say that ChatGPT is the most popular AI technology in law. While its popularity is growing rapidly, its applications differ from more specialised AI tools designed specifically for the legal sector. 2.6.1 Legal Document Creation and Editing ChatGPT can generate initial drafts of contracts, legal pleadings, and other legal documents, speeding up the document creation process. This allows solicitors to focus on substantive analysis while saving time on routine tasks. 2.6.2 Analysis and Processing of Large Data Sets The model can quickly search and analyse extensive databases, identifying key information, precedents, or court rulings. This enables more effective case strategy preparation and a better understanding of the legal context. 2.6.3 Automation of Routine Tasks ChatGPT can automate repetitive tasks, such as drafting standard responses to client enquiries or generating reports. This helps optimise team workflow and reduce administrative workload. 2.6.4 Support for Legal Research With access to a vast knowledge base, ChatGPT can provide information on applicable laws, legal interpretations, and recent legislative changes, assisting solicitors in their daily work. 2.6.5 Improving Client Communication The model can generate clear and understandable explanations of complex legal issues, improving communication with clients and increasing their satisfaction with legal services. 2.6.7 Education and Training by Legal AI Tools ChatGPT can serve as a tool for creating training materials or simulating legal cases, supporting the professional development of law firm employees. 2.6.8 Personalisation of Legal Services By analysing client data and preferences, ChatGPT can help develop personalised offers and legal strategies tailored to individual needs. It is important to note that using ChatGPT also comes with challenges, such as ensuring data confidentiality and verifying generated content for compliance with current legal regulations. Therefore, integrating this tool into law firm operations should be carefully considered and adapted to the firm’s specific needs. 2.7 Does Microsoft Offer the Best AI Tools for the Legal Industry? Microsoft provides a wide range of AI tools that can be highly useful for the legal industry, but whether they are the “best” depends on the specific needs of a law firm and how they compare to competing solutions. In addition to the previously mentioned Power Apps and Power BI, Microsoft has been heavily investing in the development of another key tool: Microsoft Copilot. Microsoft Copilot is a suite of AI-powered tools integrated with Microsoft products such as Microsoft 365, Dynamics 365, and Azure. Once integrated, Copilot works seamlessly across applications like Word, Excel, PowerPoint, Outlook, and Teams, enabling automation of various tasks. For example, in Word, Copilot can generate draft documents based on input data or transform text into different writing styles. In Excel, it can analyse large datasets, suggest appropriate charts if needed, and process natural language queries, such as “Show me data from the last three months.” This makes Copilot an ideal AI tool for automating routine tasks within Microsoft software. But what specific benefits can it bring to law firms? The answer is quite clear. Copilot enables rapid searching and analysis of large sets of legal documents, identifying key clauses and potential risks. This allows solicitors to focus on the more complex aspects of their cases while saving time on routine tasks. With its integration into Microsoft 365 applications, such as Word and PowerPoint, Copilot supports the creation of initial drafts for contracts, legal pleadings, and presentations. It can also suggest both stylistic and substantive edits, streamlining the document review process. Copilot is also a valuable tool for quickly locating legal precedents, court rulings, and legislative changes, providing up-to-date information that is essential for legal proceedings. Moreover, its integration with tools like Power Automate allows law firms to automate routine tasks, such as managing deadlines, tracking case progress, and generating reports, ultimately improving operational efficiency. Another noteworthy feature of Copilot is its ability to generate meeting summaries and draft responses to client enquiries, enhancing communication with both clients and business partners. By implementing Microsoft Copilot, law firms can not only increase productivity but also improve the quality of their services, adapting to the rapidly evolving legal landscape. Microsoft also places strong emphasis on data security. All data processed by Copilot complies with Microsoft’s privacy policies and is fully protected against unauthorised access. 3. Evaluating AI Software for Law Firms: A Strategic Approach Selecting the right AI software for a law firm requires a strategic approach that considers the organisation’s specific needs and objectives. A key part of this process is identifying the areas where AI can deliver the greatest benefits, such as automating routine tasks, analysing legal documents, or optimising case management processes. Once these areas are defined, a thorough assessment of available solutions must be conducted, focusing on functionality, compliance with legal regulations, data security, and integration with existing systems. Another crucial step is evaluating implementation costs in relation to potential savings and efficiency improvements. Finally, choosing a provider who not only delivers the right technology but also offers implementation support and team training is essential. Taking a strategic approach to evaluating AI software enables law firms to maximise the value of their investment while minimising the risks associated with adopting new technologies. 4. Effective Implementation of AI Software in Legal Practices Successfully implementing AI software in law firms requires a well-thought-out approach that combines both the technical aspects of deployment and the necessary adjustments to workflow within the team. The first step is to thoroughly understand the firm’s needs and identify the areas where artificial intelligence can bring the most value, such as automating repetitive tasks, analysing legal documents, or predicting case outcomes. Selecting the right software is a crucial stage in this process. The chosen solution should not only meet current needs but also be flexible and scalable to accommodate future technological advancements. It is equally important to ensure that the selected tool complies with existing legal regulations, such as GDPR, and adheres to high standards of data security, which is critical when handling sensitive client information. Once the software has been selected, it is essential to provide proper training for the team, allowing solicitors and administrative staff to integrate the new tool into their daily workflows effectively. Appointing technology leaders within the firm can also be beneficial, as they can assist colleagues in adapting to and fully leveraging the capabilities of AI solutions. AI software for law firms should also be continuously monitored and evaluated to measure its effectiveness. Analysing results helps identify areas for further optimisation and improvements that can enhance the software’s performance and value to the firm. In this way, artificial intelligence becomes an integral part of the firm’s strategy, contributing to higher-quality legal services and strengthening its competitive edge. 5. How Can TTMS Support the Implementation of AI Solutions Tailored to Your Needs? TTMS (Transition Technologies Managed Services) is a trusted partner in the implementation of advanced technologies, offering comprehensive support in developing and deploying AI solutions tailored to the unique needs of law firms. Through its AI4Legal offering, TTMS enables law firms to fully harness the potential of artificial intelligence in key areas such as document automation, legal data analysis, and case management optimisation. TTMS experts combine deep technological knowledge with extensive experience in legal sector implementations, ensuring the development of customised solutions that are both highly efficient and fully compliant with legal regulations. The implementation process includes an in-depth analysis of client requirements, the design and deployment of best legal AI tools, and comprehensive training for legal professionals to ensure a smooth and effective transition to modern technologies. Moreover, TTMS continues to support its clients post-implementation by providing maintenance and ongoing development services, enabling law firms to continuously improve their operational efficiency. TTMS is the ideal partner for law firms looking to invest in innovation while maintaining the highest standards of security and service quality. Contact us now! Check our related case studies: Case Study – AI Implementation for Court Document Analysis Using AI in Corporate Training Development: Case Study AI-Driven SEO Meta Optimisation in AEM: Stäubli Case Study Didn’t find the answers to your questions in this article? Check out the FAQ section. What is AI-powered legal software? AI-powered legal software refers to technological solutions designed to assist solicitors in document analysis, process automation, and decision-making. It utilises advanced AI algorithms, such as natural language processing (NLP) and machine learning, to quickly search databases, identify key information, and suggest solutions. These tools can draft contracts, assess legal risks, and provide predictions on case outcomes. By reducing the time and costs associated with routine tasks, AI-driven legal software enhances law firm productivity. It is particularly useful in due diligence analysis, contract management, and regulatory compliance. What are the key characteristics of AI-powered legal technology? Legal technology powered by artificial intelligence is characterised by the automation of processes such as contract analysis and creation, legal research, and case management. By leveraging natural language processing (NLP), AI can quickly scan legal documents, identify key clauses, and suggest modifications, improving efficiency and accuracy in legal workflows. What challenges will law firms and AI in the legal sector face in 2025? Law firms and the use of AI in law will face significant challenges in 2025. Among the most pressing issues are client data protection, compliance with AI-related legal regulations, and liability for errors generated by AI algorithms. Additionally, the adoption of AI in law firms requires investment in technology infrastructure and employee training. There are also concerns related to ethics and the potential replacement of human roles by technology. However, firms that successfully integrate AI into their operations can gain a competitive advantage through process automation and increased efficiency. How popular is artificial intelligence in law in the USA compared to Europe? AI in law is gaining traction in both the USA and Europe, but adoption is generally faster in the USA. American law firms are more open to AI-driven automation, especially for legal research, document analysis, and contract management. Europe, while embracing AI, faces stricter regulations, such as GDPR, which impact AI implementation. The USA has a stronger start-up ecosystem for legal AI, whereas Europe focuses more on compliance and ethical concerns. Despite differences, both regions recognise AI’s potential in improving efficiency and reducing costs. Is legal AI technology the same in the USA and Europe, or are there significant differences in its development and regulation? Legal AI technology is similar in both regions in terms of capabilities, but there are key differences in regulation and adoption speed. The USA has a more flexible regulatory environment, allowing for faster innovation and AI integration in legal services. Europe, on the other hand, has stricter data protection laws, such as GDPR, which influence how AI can be used in legal practices. Additionally, some European countries have specific guidelines on AI ethics and transparency, impacting AI deployment in law firms. These regulatory differences mean that legal AI adoption in Europe often requires additional compliance measures. How much of a competitive advantage does artificial intelligence legal software give a law firm in winning a case? AI legal software provides a significant advantage by improving research speed, document review, and case prediction. AI tools can analyse vast amounts of legal data in seconds, identifying relevant precedents and potential risks more efficiently than humans. However, AI alone does not guarantee winning a case—it serves as a support tool that enhances decision-making rather than replacing legal expertise. The firms that integrate AI with experienced legal professionals gain the most competitive edge. Ultimately, AI boosts efficiency and accuracy, but legal strategy and human judgement remain crucial. Is the use of artificial intelligence in law in court proceedings accepted by the justice system? The acceptance of artificial intelligence in law in court proceedings varies depending on the jurisdiction. In the USA, AI is increasingly used for legal research, case analysis, and document automation, but courts remain cautious about AI-generated legal arguments and decisions. In Europe, AI tools are used primarily for administrative and analytical support, while direct AI involvement in judicial decision-making is heavily regulated. Many legal systems require human oversight to ensure fairness, accuracy, and accountability in legal proceedings. While AI is a valuable tool, its role in court is still limited to supporting, not replacing, human judgement. Is it possible to quickly gain the skills needed to effectively use law firm AI software? Yes, many law firm AI tools are designed to be user-friendly and do not require advanced technical knowledge. Training programmes and onboarding sessions provided by software vendors help legal professionals adapt quickly. However, mastering AI-assisted legal research and document automation may take time, depending on the complexity of the software. Continuous learning is essential, as AI capabilities evolve and new features are introduced. While basic use can be learnt quickly, maximising AI’s potential requires ongoing training and adaptation.
ReadChatGPT 4.5 – What’s New? Practical Examples and Applications
OpenAI has released a long-awaited update to its popular language model, ChatGPT 4.5, also known as Orion. GPT-4.5 is OpenAI’s largest and most advanced language model to date. The new version of the model brings significant improvements in creativity, emotional intelligence, information accuracy, and context understanding. So let’s take a closer look at it. 1. Why does GPT-4.5 understand the world better? GPT-4.5 better “understands the world” thanks to several key improvements in the way it was designed and trained: Advanced unsupervised learning scaling: The model was trained on massive text datasets without direct supervision, allowing it to “autonomously” discover linguistic structures, word relationships, and contexts. This gives GPT-4.5 a more intuitive grasp of linguistic nuances, which translates into a better understanding of content and user intent. Intensive training on Microsoft Azure AI supercomputers: The use of powerful computing resources has enabled the processing of much larger amounts of data and the use of more complex model architectures. Such mass-scale training allows for: Expanding the knowledge base, which results in a deeper understanding of reality. Improving pattern recognition mechanisms, which helps minimize errors such as so-called “hallucinations” – i.e. generating inaccurate or false information. Better combining unsupervised learning with reasoning: Although GPT-4.5 relies primarily on unsupervised learning, modern techniques also allow it to effectively use elements of reasoning. This allows the model to not only analyze data, but also interpret and apply it in context, resulting in more precise and accurate answers. Optimization of training architecture and techniques: Improvements in the model architecture and the use of advanced training techniques (such as a combination of traditional training and reinforcement learning) allow for better detection of subtle relationships and contexts. As a result, GPT-4.5 is better able to “understand” both the literal and figurative meaning of texts, making it more flexible and reliable in interpreting human speech. In short, by combining extensive, scalable training on massive datasets, a modern architecture, and powerful computing resources, GPT-4.5 gains the ability to understand the world more deeply and intuitively. This approach enables it to provide more accurate, consistent, and empathetic responses, which is crucial in everyday interactions with users. 2. Does GPT-4.5 actually generate more creative responses? Early tests show that interactions with GPT-4.5 are much more natural, fluid, and creative. The model generates higher quality, more engaging, and vivid statements, better tailored to the user’s intent. Let’s compare the capabilities of Orion and the previous version of the model using 3 prompts: Prompt 1: “Describe briefly but creatively an autumn afternoon.” GPT-4: “The autumn afternoon is cool and leaves are falling from the trees.” GPT-4.5: “The autumn afternoon smells of wet earth and warm tea, while the sun streaks golden through the reddening leaves. The wind whispers softly through the branches, carrying memories of summer past.” Prompt 2: “Describe briefly but creatively a morning walk in a city park.” GPT-4: “A morning walk in a city park is a peaceful moment when nature comes to life and people enjoy the fresh air.” GPT-4.5: “A morning walk in a city park is like immersing yourself in living poetry – fog gently drifts between the trees and the first rays of sunlight break through the leaves, waking up the city to the joyful symphony of the day.” Prompt 3: “Create a creative vision of the future where nature and technology coexist.” GPT-4: “In the future, nature and technology will coexist, creating a harmonious reality where people enjoy advanced technology while caring for the environment.” GPT-4.5: “Imagine a world where glass skyscrapers blend into lush, green forests, and digital trees grow alongside real ones. Interactive gardens pulsate with energy, and the symbiosis of technology and nature creates a poetic mosaic of a new era.” Of particular note is the direct address to the recipient (“Imagine…”) and… the response time. Generating the text (which, by the way, is longer) took noticeably less time in the case of Chat GPT 4.5. 3. How does GPT-4.5 deal with emotions? GPT-4.5 shows significant improvement in emotional intelligence by using Reinforcement Learning with Human Feedback (RLHF). This is a training technique in which the AI model learns not only from raw data, but also from feedback from humans evaluating its responses. Experts analyze the different variants of the responses generated by the model and choose the ones that are most relevant, empathetic, and in line with the user’s intention. Based on this, a special reward model is created that teaches GPT-4.5 what responses it should prefer to be more useful, natural, and supportive in the conversation. This allows the model to better interpret emotions, avoid inappropriate responses, and provide more empathetic and personalized responses. New training techniques increase the model’s ability to pick up on subtle emotional cues and intentions, which translates into more empathetic, natural, and situationally appropriate responses. The model not only understands words, but also the emotional context, making it a better conversation partner. 3.1 How does GPT-4.5 interpret emotions? GPT-4.5 is trained on human interactions and expert ratings, allowing it to: Recognize tone of voice – it can distinguish between a happy tone and a sad or sarcastic one. Adjust response style – when a user is expressing frustration, the model will respond with a more calm, supportive tone, while in a happy context it may use more enthusiastic language. Better response to sensitive topics – with RLHF, the model avoids trivializing difficult emotions and instead offers more supportive and empathetic responses. 3.2 Empathy in practice Through RLHF, GPT-4.5 learned to adjust his responses to sound more natural and appropriate to the situation: Example: User prompt: “I feel down today.” GPT-4 (without RLHF): “I’m sorry to hear that. I hope it gets better.” GPT-4.5 (with RLHF): “I’m sorry you feel that way. Do you want to talk about it? Maybe I can help, suggest something to cheer you up or take your mind off things?” We see that the GPT-4.5 response is more caring, attuned to the user’s emotions, and offers the opportunity to continue the conversation in a supportive way. 3.3 Fewer “emotional” errors and more naturalness Thanks to RLHF, the model avoids misinterpreting emotions that could lead to inappropriate reactions. GPT-4.5’s responses are more natural, fluid, and tailored to the user’s needs, making the conversation with AI more human. In short, GPT-4.5 not only understands emotions better, but also responds to them appropriately, making it a more effective tool in interactions that require empathy and sensitivity. 4. Does GPT-4.5 make fewer errors? GPT-4.5 has significantly reduced the number of so-called “hallucinations”—false or fictitious information that AI models generate when they don’t have enough data to provide an accurate answer. Hallucinations can include false facts, misinterpretations, or even completely made-up content that sounds plausible at first glance. To mitigate this problem, OpenAI has made several significant improvements to the new version of the model. GPT-4.5 has been trained on an even larger and more diverse dataset, allowing it to better understand reality and fill in missing information with guesswork less often. At the same time, the new model architecture improves the way it processes information and recognizes patterns, which increases the consistency and precision of the answers it generates. In addition, the use of reinforcement learning based on human feedback (RLHF) plays an important role. Thanks to this technique, experts evaluate the model’s responses and indicate which are more accurate and consistent with reality, which allows GPT-4.5 to distinguish true information from false information more effectively. As a result, the model is less likely to provide non-existent facts as certainties. Uncertainty detection mechanisms have also been improved, thanks to which GPT-4.5 better recognizes situations in which it lacks data. Instead of providing false information with confidence, it uses more cautious formulations, suggesting the user to check reliable sources. Another new feature is greater flexibility in updating knowledge through integration with dynamic data sources and the ability to adjust the model to specific needs through fine-tuning. Thanks to this, GPT-4.5 reduces the risk of providing outdated information and better adapts to real, changing conditions. While no AI is completely free from errors, the improvements in this version make the model much more precise, logical, and aware of its own limitations, making its answers more reliable and useful in everyday use. 5. Is GPT-4.5 the basis for future reasoning models? Chat GPT-4.5, also known as Orion, is a significant step forward in the development of language models, focusing on advanced unsupervised learning. OpenAI plans for such models to become a solid foundation for future systems developing advanced logical and technical reasoning capabilities. In the future, it is expected to integrate unsupervised learning methods with reasoning techniques, which will increase the versatility of the AI. In terms of further plans, OpenAI is working on the GPT-5 model, which is expected to introduce significant improvements. According to the information, GPT-5, also known as Orion, has been in development for 18 months, but has encountered delays and high costs associated with training the model. Challenges include a lack of sufficient and high-quality data and competition for computing resources. To overcome these limitations, OpenAI is hiring experts to generate new data and is exploring the possibility of using synthetic data created by existing AI models, although this is associated with certain risks. Despite these challenges, Microsoft is preparing to host the upcoming GPT-4.5 and GPT-5 models on its servers. GPT-5, integrating more OpenAI technologies, including the new o3 reasoning model, is expected around the end of May. The goal is to create a more advanced AI system, approaching artificial general intelligence (AGI). It also plans to unify the o-series and GPT models to improve user experience by eliminating the need to choose the right model for a specific task. The introduction of GPT-5 also aims to simplify OpenAI’s product offering. Currently, users have to choose between different models, which can be complicated. The new system is supposed to automatically analyze content and choose the best model, increasing usability in different contexts. Importantly, GPT-5 is to be available in an “unrestricted way” as a free version, which could increase its accessibility to a wider range of users. 6. How does GPT-4.5 ensure user security? Security remains a key aspect of all OpenAI models, and GPT-4.5 is designed to minimize the risk of erroneous, malicious, or inappropriate responses. The model has undergone extensive testing against a comprehensive Preparedness Framework that includes analyzing potential threats, mitigating the risk of generating malicious content, and implementing measures to prevent misuse. Using advanced supervision, the model is constantly monitored for correctness and security. One key element of ensuring security is the combination of traditional supervised training (SFT) and reinforcement learning based on human feedback (RLHF). This allows the model to better understand the context and intent of the user, allowing it to avoid inappropriate content and adapt responses in a more ethical and consistent way. Human judgment also helps eliminate biases and reduce the risk of generating content that could be disinformative, aggressive, or dangerous. Additionally, GPT-4.5 has been equipped with uncertainty detection mechanisms that allow it to better recognize situations where it does not have enough data to provide a confident response. Rather than providing misinformation, the model is more likely to suggest fact-checking with credible sources or being cautious with its claims. Another important aspect of security is implementing content filters and abuse mitigation systems that help detect and block potentially harmful queries. 7. Who can use GPT-4.5 and what are the costs? Thanks to its numerous improvements, GPT-4.5 is widely used in many areas, where its ability to generate natural, contextually tailored and precise responses can significantly improve various processes. In customer service, the model works as a tool supporting interactions with users, providing more natural, empathetic and personalized responses. Thanks to a better understanding of the context and intentions of customers, it can help solve problems, answer queries more precisely and effectively establish dialogue, which increases the level of user satisfaction. Integration of GPT-4.5 with chatbots and automated service systems allows for faster and more accurate responses, while reducing the burden on support staff. In marketing and copywriting: the model is a powerful tool for generating attractive advertising content, social media posts, slogans or even comprehensive blog articles. Thanks to the ability to create creative and engaging texts, it can support marketers in creating promotional campaigns tailored to different groups of recipients. What’s more, GPT-4.5 can analyze data and adapt its message to the brand’s tone and style, which allows for consistent communication and better targeting of customer needs. In psychological support: the model can act as a first line of emotional support, offering users support in difficult moments. Thanks to increased emotional intelligence and the ability to recognize subtle emotional cues, GPT-4.5 can adjust the tone of speech to the situation, providing more empathetic and caring responses. Although it does not replace professional therapy, it can act as an assistant supporting people looking for comfort, motivation or strategies to cope with everyday emotional challenges. In education: the model works perfectly as a tool supporting the learning process. Thanks to its ability to precisely answer questions of pupils and students, it can help in acquiring knowledge, explaining complex issues in an accessible way and providing interactive educational materials. It can also support teachers in creating tests, teaching materials or lesson plans, as well as help students learn foreign languages through interactive conversations and error correction. Thanks to its advanced natural language processing mechanisms, GPT-4.5 can also be used in many other areas, such as data analysis, scientific research, software development, and even supporting business decision-making. Its versatility and improved information processing capabilities make it an extremely useful tool in the modern digital world. 8. ChatGPT 4.5 – A game-changing AI? GPT-4.5 is a significant step forward in the development of artificial intelligence, significantly improving the quality of interactions between users and the AI model. With better understanding of context, greater creativity, more empathetic responses and error reduction, the new version of the model becomes an even more versatile tool. It is used in customer service, marketing, education, data analysis and even emotional support, making it an invaluable support for business and everyday users. Artificial intelligence is not only the technology of the future, but a tool that is already revolutionizing the way we work and communicate. At Transition Technologies MS, we specialize in providing advanced AI solutions for business that support process automation, operation optimization and efficiency improvement in various industries. Contact us! What is Orion, and how does it relate to ChatGPT 4.5? Orion is the internal codename for ChatGPT 4.5, used by OpenAI to differentiate this upgraded model from previous versions. While the name “ChatGPT 4.5” is used publicly, “Orion” is often mentioned in internal and technical discussions. This version brings significant improvements in creativity, emotional intelligence, accuracy, and contextual understanding, making interactions more fluid and natural. What is unsupervised learning, and how does GPT-4.5 use it? Unsupervised learning is a machine learning technique where a model learns patterns, relationships, and structures from data without explicit human-labeled annotations. In GPT-4.5, unsupervised learning enables the model to absorb vast amounts of text data, recognize language patterns, and generate human-like responses without requiring direct supervision. This approach allows the AI to refine its understanding of language, context, and nuance, improving its ability to generate coherent and contextually relevant answers. What is RLHF, and why is it important for ChatGPT 4.5? Reinforcement Learning from Human Feedback (RLHF) is a training method that improves AI models by incorporating human feedback. In this process, human evaluators assess AI-generated responses, ranking them based on quality, accuracy, and ethical considerations. The model then learns from this feedback through reinforcement learning, adjusting its responses to align better with human expectations. RLHF in GPT-4.5 enhances its emotional intelligence, reduces misinformation, and ensures that generated responses are more aligned with user intent, making interactions more natural and empathetic. What is the Preparedness Framework, and how does it ensure safety in GPT-4.5? The Preparedness Framework is a structured safety and risk assessment approach used by OpenAI to evaluate AI models before deployment. It focuses on identifying potential risks such as misinformation, bias, security vulnerabilities, and harmful content generation. By implementing this framework, OpenAI ensures that GPT-4.5 meets safety standards, minimizes harmful outputs, and adheres to ethical guidelines. The model undergoes extensive testing to refine its responses and reduce risks associated with AI-driven conversations. What is SFT, and how does it contribute to model improvement? Supervised Fine-Tuning (SFT) is a training technique where AI models are improved using high-quality, human-annotated datasets. Unlike unsupervised learning, where the model learns from raw data without guidance, SFT involves explicitly labeled examples to correct and refine the model’s outputs. For GPT-4.5, SFT helps improve factual accuracy, coherence, and ethical alignment by reinforcing desired behaviors and eliminating biases. This fine-tuning process is essential for ensuring that the model generates reliable, safe, and contextually appropriate responses.
ReadBoost Operational Efficiency with AI – Speed up Your Business
In today’s fast-paced business environment, artificial intelligence (AI) is a game-changer for operational efficiency. Companies leveraging AI streamline processes, reduce costs, and improve performance, gaining a competitive edge. AI goes beyond automation—it provides data-driven insights that enhance decision-making and precision. This article explores practical AI applications across industries and strategies to help your business achieve greater efficiency. 1. How AI Transforms Operational Efficiency 1.1 What Is Operational Efficiency? Operational efficiency means delivering products or services in the most cost-effective way while maintaining quality. Businesses that optimize processes experience lower costs, faster workflows, and higher customer satisfaction. However, traditional methods often fall short in managing complex operations. 1.2 AI’s Evolving Role in Operations Management AI in operations management is no longer just about automating tasks—it’s revolutionizing efficiency. By analyzing vast datasets, AI identifies optimization opportunities beyond human capabilities. AI operational efficiency enhances decision-making, reduces errors, and streamlines resource allocation. Companies leveraging artificial intelligence efficiency gain a competitive advantage through predictive maintenance, intelligent supply chain management, and automated workflows. AI in operations adapts over time, continuously improving efficiency. Organizations that strategically implement AI for operations can unlock new business models, redefining industry standards. 2. Key Benefits of AI in Boosting Operational Efficiency 2.1 Process Automation: Reducing Errors and Increasing Productivity AI operational efficiency allows businesses to automate repetitive tasks, reducing human error and freeing employees for strategic work. Efficiency AI solutions improve accuracy, ensuring consistent performance without fatigue. Many industries report reduced production time and improved workflows with AI in operations management. 2.1.1 Better Decision-Making with AI Efficiency AI-driven analytics transform vast data into actionable insights, enhancing decision-making. AI for operations enables predictive analytics, helping businesses optimize inventory, resource allocation, and maintenance. Artificial intelligence in operations management ensures organizations shift from reactive to proactive strategies, increasing efficiency and performance. 2.1.2 Cost Reduction and Revenue Growth AI operational efficiency drives cost savings through process optimization, waste reduction, and predictive maintenance. AI in operations minimizes downtime and extends asset lifespan. Artificial intelligence efficiency also enhances revenue generation by improving customer experiences and accelerating product development. By leveraging AI and efficiency strategies, companies streamline operations, reduce costs, and gain a competitive edge. 2.2 Practical Applications of AI Across Industries 2.2.1 AI in Healthcare: Enhancing Patient Care and Operational Efficiency AI in operations management is transforming healthcare by optimizing both clinical and administrative processes. AI-driven diagnostics, such as IBM Watson Health, analyze vast medical datasets to improve disease detection and treatment recommendations. AI operational efficiency enhances hospital management by predicting patient admissions, optimizing bed allocation, and automating scheduling. Efficiency AI solutions also streamline administrative workflows, reducing paperwork and freeing medical staff for patient care. AI for operations in early disease detection identifies patterns in medical images, allowing for faster and more accurate diagnoses. Artificial intelligence in operations management not only improves patient outcomes but also reduces operational costs, making healthcare more efficient. 2.2.2 AI in Energy: Optimizing Grid Management and Predictive Maintenance The energy sector benefits significantly from AI operational efficiency, particularly in grid optimization and predictive maintenance. AI in operations enhances energy distribution, reduces downtime, and improves demand forecasting. AI-driven predictive analytics help energy companies anticipate equipment failures, extending the lifespan of critical infrastructure and minimizing costly repairs. TTMS has developed scalable AI efficiency solutions that consolidate multiple systems for a leading energy provider. By implementing artificial intelligence in operations management, companies in the energy sector can reduce operational costs, improve resource management, and enhance sustainability efforts. 2.2.3 AI for Legal: Automating Document Analysis and Risk Assessment AI in operations is revolutionizing legal services by automating time-consuming processes like contract review and risk assessment. AI-powered tools analyze thousands of legal documents in seconds, improving accuracy and reducing workload. Efficiency AI applications in law firms streamline case research, identify precedents, and predict litigation outcomes. AI operational efficiency enhances compliance monitoring, ensuring firms stay updated with regulatory changes. With artificial intelligence in operations management, legal teams improve productivity, minimize errors, and focus on higher-value tasks. 2.2.4 AI in Manufacturing: Quality Control and Predictive Maintenance Manufacturing is one of the most AI-driven industries, leveraging artificial intelligence efficiency to enhance production quality and reduce downtime. AI-powered predictive maintenance analyzes sensor data to prevent unexpected equipment failures, increasing productivity and reducing costs. Computer vision systems also play a key role in AI in operations by detecting defects with higher accuracy than manual inspections, improving product quality while minimizing waste. AI operational efficiency allows manufacturers to optimize supply chains and streamline production workflows. 2.2.5 AI in Retail: Personalized Customer Experiences and Supply Chain Optimization AI in operations management has reshaped retail by optimizing both customer interactions and logistics. AI-driven demand forecasting predicts inventory needs, reducing stock shortages and excess supply. AI operational efficiency enhances pricing strategies with real-time adjustments based on demand trends. On the customer-facing side, artificial intelligence in operations management personalizes shopping experiences with recommendation engines, increasing conversions and customer satisfaction. AI-powered chatbots further enhance efficiency AI solutions in customer support, resolving inquiries instantly. 2.2.6 AI in Finance: Fraud Detection and Risk Management Financial institutions leverage AI in operations to detect fraud and improve risk assessment. AI-driven fraud detection systems analyze thousands of transactions per second, identifying suspicious patterns and preventing fraudulent activities in real-time. AI operational efficiency also improves credit risk assessments by analyzing both traditional and alternative data sources, ensuring better lending decisions. Artificial intelligence efficiency in financial operations streamlines compliance monitoring and regulatory reporting. 2.2.7 AI in Telecom: Network Optimization and Event Forecasting AI for operations in telecom focuses on network optimization and congestion management. AI-driven systems analyze historical network data, event calendars, and real-time demand to prevent service disruptions. By leveraging AI operational efficiency, telecom providers can allocate network resources dynamically, ensuring uninterrupted service during peak demand. Artificial intelligence in operations management enhances customer satisfaction while optimizing infrastructure investments. 3. AI Technologies Driving Operational Transformation 3.1 Machine Learning: Smarter, Adaptive Decision-Making Machine learning is the backbone of AI in operations, enabling systems to continuously learn and improve. Unlike static automation, machine learning-driven AI operational efficiency enhances decision-making by analyzing vast datasets and detecting hidden patterns. AI for operations leverages predictive analytics to optimize maintenance schedules, detect anomalies, and refine resource allocation. Deep learning, a subset of machine learning, expands artificial intelligence efficiency by processing unstructured data, such as images and speech, further enhancing operational insights. 3.2 Natural Language Processing (NLP): Automating Communication and Data Analysis AI efficiency solutions powered by NLP transform how businesses handle communication and documentation. AI in operations management enables chatbots and virtual assistants to handle customer inquiries 24/7, reducing response times and improving service quality. NLP also streamlines internal operations by analyzing and summarizing vast amounts of text data, such as contracts, emails, and reports. AI operational efficiency in this area eliminates manual review, reducing processing times and improving accuracy. 3.3 Robotic Process Automation (RPA): Automating Routine Tasks with AI AI-enhanced RPA automates repetitive, rules-based tasks with precision, freeing employees for higher-value work. AI in operations allows businesses to integrate automation with machine learning, enabling systems to adapt to process variations rather than following rigid scripts. Efficiency AI applications in RPA are widely used for data entry, invoice processing, and workflow automation. AI operational efficiency ensures near-perfect accuracy and faster execution, reducing costs and minimizing errors. 3.4 Computer Vision: Enhancing Quality Control and Security AI operational efficiency extends beyond digital processes through computer vision, which interprets visual data for real-world applications. AI in operations management improves manufacturing quality control, detecting product defects more accurately than human inspectors. In security and logistics, AI for operations enhances monitoring by analyzing surveillance footage in real-time, identifying safety hazards and unauthorized access. Artificial intelligence efficiency in these applications improves safety, compliance, and operational performance. 4. Concluding Insights: Embracing AI for Sustainable Operational Success 4.1 Key Strategies for Business Leaders To boost operational efficiency with AI, businesses must take a strategic approach. Successful AI adoption starts with identifying critical inefficiencies and selecting AI solutions that deliver measurable value. AI operational efficiency depends on high-quality data—without a solid data infrastructure, even advanced AI systems will underperform. Cross-functional collaboration is crucial. AI in operations management works best when technical teams, business leaders, and end-users align their goals. Training employees to work alongside AI enhances adoption and maximizes returns. AI operational efficiency should complement human expertise, not replace it. Governance and ethical oversight are equally important. Organizations must ensure AI in operations adheres to regulatory standards while maintaining transparency and accountability. A well-structured AI strategy prevents risks while driving long-term benefits. 4.2 Long-Term Benefits of AI Adoption AI efficiency compounds over time, delivering enhanced operational efficiency through continuous learning and adaptation. Businesses leveraging AI for operations gain agility, allowing them to respond faster to market changes and customer needs. AI operational efficiency also improves decision-making by refining analytics models, leading to smarter, data-driven strategies. Additionally, artificial intelligence efficiency in customer interactions increases satisfaction and retention, driving revenue growth. Companies that integrate AI in operations management effectively will achieve a sustainable competitive edge. The key is ongoing refinement—organizations must continuously optimize their AI strategies to stay ahead in an increasingly AI-driven business landscape. 5. How TTMS can help you with implementing AI for Boosting Operational Efficiency? 5.1 How TTMS Can Help You Implement AI for Boosting Operational Efficiency At TTMS, we specialize in delivering AI-powered solutions that enhance operational efficiency across industries. Our expertise in AI in operations management allows businesses to streamline workflows, reduce costs, and gain a competitive edge. 5.1.1 Tailored AI Strategies for Your Business We start with an in-depth analysis of your current processes to identify key areas where AI operational efficiency can deliver measurable improvements. Our experts develop customized AI solutions that integrate seamlessly with your existing infrastructure, ensuring minimal disruption and maximum impact. 5.1.2 Seamless AI Implementation and Integration TTMS specializes in deploying AI for operations with a focus on scalability and flexibility. Whether it’s process automation, predictive analytics, or AI-driven decision-making, we provide end-to-end implementation tailored to your needs. As partners of leading technology providers such as AEM, Salesforce, and Microsoft, we ensure that our solutions align with industry best practices. 5.1.3 AI-Powered Process Automation and Business Intelligence We help businesses automate repetitive tasks. Our Business Intelligence (BI) solutions, powered by tools like Snowflake DWH and Power BI, transform raw data into actionable insights, supporting enhanced operational efficiency and data-driven decision-making. 5.1.4 Long-Term Support and Continuous Optimization AI is not a one-time implementation—it requires ongoing optimization. TTMS has extensive experience in building long-term partnerships, continuously supporting clients in optimizing and evolving their AI solutions. Our dedicated teams ensure that your AI-driven processes remain efficient, adaptable, and aligned with your business goals as they grow and change. By partnering with TTMS, you gain access to a team that understands AI in operations and is committed to delivering artificial intelligence efficiency that drives sustainable success. 5.2 Let’s Talk About AI for Your Business Looking to boost operational efficiency with AI? Get in touch with our experts at TTMS to explore how AI can transform your business operations. We’ll help you identify opportunities, implement tailored solutions, and support you every step of the way. FAQ How does AI improve efficiency? AI boosts operational efficiency by automating repetitive tasks, reducing human errors, and optimizing decision-making. AI-driven analytics process vast amounts of data, uncovering patterns that improve workflows, resource allocation, and predictive maintenance. With AI in operations, businesses shift from reactive to proactive strategies, minimizing downtime and maximizing productivity. What is operational efficiency in business? Operational efficiency is the ability to deliver products or services with minimal waste while maintaining quality. AI operational efficiency enhances process automation, resource utilization, and decision-making, helping businesses reduce costs and improve performance. How does AI increase efficiency? AI increases efficiency by automating workflows, analyzing data for better decision-making, and predicting outcomes to optimize operations. AI for operations reduces errors, speeds up processes, and ensures optimal resource allocation, resulting in cost savings and improved performance. How can artificial intelligence help managers enhance business operations? AI in operations management helps managers make data-driven decisions, optimize resource allocation, and improve forecasting. AI operational efficiency automates routine tasks, allowing managers to focus on strategic initiatives while enhancing overall business agility and performance.
ReadAI Security Risks Explained: What You Need to Know in 2025
In an era where artificial intelligence reshapes our digital landscape, the security implications are becoming increasingly critical. With AI-powered cyberattacks projected to surge by 50% in 2024 compared to 2021, organizations face unprecedented challenges in protecting their digital assets. As the AI security market races toward a staggering $60.24 billion by 2029, understanding and addressing AI security risks isn’t just important—it’s imperative for survival in our tech-driven world. 1. AI Security Risks in 2025: A Practical Overview The cybersecurity landscape is undergoing a dramatic transformation as we approach 2025. According to recent studies, 93% of security leaders expect their organizations to face daily AI-driven attacks by 2025. This stark reality demands immediate attention and strategic preparation from businesses of all sizes to protect their digital infrastructure against increasingly sophisticated threats. 1.1 AI’s Role in Security: Opportunities and Risks Artificial intelligence presents a double-edged sword in the cybersecurity realm. On the defensive side, AI systems excel at detecting patterns in vast datasets, identifying potential threats before they materialize, and automating security responses at speeds impossible for human analysts. The market growth, projected at a CAGR of 19.02% between 2024-2029, reflects the increasing adoption of AI-powered security solutions. However, this technological advancement comes with inherent vulnerabilities. While AI strengthens our defense mechanisms, it also introduces new attack vectors that malicious actors can exploit. The complexity of AI systems makes them susceptible to data poisoning, where attackers can manipulate the training data to compromise the AI’s decision-making process. The challenge lies in balancing AI’s transformative potential with its security implications. Organizations must navigate this landscape carefully, implementing robust security frameworks while leveraging AI’s capabilities. This delicate balance requires a deep understanding of both the opportunities and risks associated with AI integration in security systems. 2. Key Security Risks of Artificial Intelligence The security risks of artificial intelligence represent a growing concern across industries. As AI systems become more sophisticated, the potential vulnerabilities and threats multiply, creating complex challenges for organizations implementing these technologies. 2.1 AI-Driven Cyberattacks Among the most pressing AI security risks, AI-powered cyberattacks stand out for their sophistication and scale. These attacks leverage machine learning algorithms to bypass traditional security measures with unprecedented precision. Cybercriminals are now using AI to automate attacks, making them more efficient and harder to detect. The ability of AI systems to learn and adapt means that attack patterns can evolve in real-time, presenting a significant challenge for conventional security measures. 2.2 Manipulating AI: Adversarial Attacks and Data Poisoning One of the critical security risks of AI involves the manipulation of AI systems through adversarial attacks and data poisoning. Attackers can subtly alter input data to confuse AI models, causing them to make incorrect decisions. For instance, slight modifications to traffic signs could mislead autonomous vehicles, while corrupted training data might compromise facial recognition systems. These attacks are particularly concerning because they can be difficult to detect until significant damage has occurred. 2.3 Prototype Theft and Unauthorized Use The theft of AI model prototypes represents another significant artificial intelligence security risk. Sophisticated attackers can reverse-engineer AI models to steal intellectual property or identify vulnerabilities. This not only compromises competitive advantages but also enables malicious actors to create unauthorized copies of proprietary AI systems, potentially bypassing built-in safety measures. 2.4 Using an Unauthorized Language Model to Develop Software The deployment of unauthorized language models in software development introduces substantial security risks of artificial intelligence. When developers use unverified or compromised AI models, they risk incorporating vulnerabilities or backdoors into their applications. These security gaps can remain undetected for extended periods, creating potential entry points for cyberattacks. 2.5 Ethical and Privacy Challenges AI systems often process vast amounts of sensitive data, raising significant privacy concerns. The AI security risk extends beyond technical vulnerabilities to include ethical considerations about data handling and user privacy. Organizations must carefully balance the benefits of AI implementation with the need to protect individual privacy rights and maintain ethical standards. 2.6 Transparency Issues in AI Models The “black box” nature of many AI systems presents a unique security risk of ai. When organizations can’t fully understand how their AI makes decisions, it becomes challenging to identify potential vulnerabilities or biases. This lack of transparency can lead to undetected security breaches or discriminatory outcomes, making it crucial for organizations to implement explainable AI practices. 2.7 AI-Generated Deepfakes and Misinformation Perhaps one of the most visible security risks of artificial intelligence is the creation of sophisticated deepfakes and misinformation. AI-powered tools can generate increasingly convincing fake content, from manipulated videos to synthetic voice recordings. This capability poses serious threats to information security, reputation management, and social stability, requiring robust detection mechanisms and verification protocols. 3. Strengthening AI Security: Solutions and Best Practices As organizations increasingly adopt AI technologies, implementing robust security measures becomes crucial. Understanding how to leverage AI for cybersecurity while protecting against potential threats requires a comprehensive approach combining technical controls, verification processes, and regular assessments. 3.1 Improving Model Security and Access Controls The foundation of strong AI security lies in implementing robust model protection and access controls. Organizations must establish multi-layered security protocols that include encryption of model parameters, secure API endpoints, and granular access permissions. By implementing role-based access control (RBAC) and monitoring systems, companies can track who interacts with AI models and detect potential security breaches early. 3.2 Verification of artificial intelligence models used in the company and by suppliers The AI impact on cybersecurity extends beyond internal systems to include third-party AI models and services. Organizations should establish rigorous verification processes for all AI models, whether developed in-house or provided by suppliers. This includes conducting thorough security assessments, reviewing model documentation, and ensuring compliance with security standards. Regular validation of model behavior helps identify potential vulnerabilities or unauthorized modifications. 3.3 Using AI for Threat Detection and Prevention Using AI for cybersecurity represents a powerful approach to protecting digital assets. Advanced AI systems can analyze vast amounts of data in real-time, identifying patterns and anomalies that might indicate security threats. These systems can: Monitor network traffic for suspicious activities Detect and respond to potential security breaches automatically Predict and prevent future security incidents based on historical data Enhance traditional security measures with AI-powered insights 3.4 Conducting Regular Security Audits and Incident Response Drills The relationship between generative AI and cybersecurity necessitates regular security assessments and preparedness testing. Organizations should implement: Scheduled security audits to evaluate AI system vulnerabilities Regular penetration testing to identify potential security gaps Incident response drills that simulate various AI-related security scenarios Documentation and review of security incidents for continuous improvement These practices ensure that security measures remain effective and that teams are prepared to respond to emerging threats in the rapidly evolving landscape of AI security. 4. The Future of AI and Cybersecurity The evolving landscape of artificial intelligence is reshaping cybersecurity practices, presenting both unprecedented challenges and innovative solutions. As we look toward the future, understanding the intersection of these technologies becomes crucial for organizational security. 4.1 Generative AI: Risks and Opportunities The security risks of generative AI are becoming increasingly complex as these technologies advance. While generative AI offers powerful capabilities for creating content and automating processes, it also introduces significant vulnerabilities. Organizations face challenges such as: AI-powered social engineering attacks becoming more sophisticated and harder to detect Automated creation of convincing phishing emails and malicious code Generation of deepfakes for corporate espionage or reputation damage However, the AI impact on cybersecurity isn’t entirely negative. Generative AI also provides valuable defensive capabilities: Enhanced threat detection through pattern recognition Automated response to emerging security threats Creation of more robust security protocols and testing scenarios 4.2 Preparing for AI Security Challenges Ahead As AI cybersecurity threats continue to evolve, organizations must adopt forward-thinking strategies to stay protected. The relationship between generative AI and cybersecurity requires a multi-faceted approach to future preparedness: Investment in Advanced Security Infrastructure Implementing AI-powered security tools Developing robust incident response capabilities Creating adaptive security frameworks that evolve with threats Workforce Development Training security teams in AI-specific threat detection Building expertise in AI security assessment Fostering collaboration between AI developers and security professionals Risk Management Strategies Regular assessment of emerging generative AI risks Development of AI-specific security policies Creation of incident response plans tailored to AI-related threats The future demands a balanced approach that leverages AI’s benefits while maintaining strong defenses against its potential misuse. Organizations that prepare now for tomorrow’s challenges will be better positioned to protect their assets and maintain security in an AI-driven world. 5. How TTMS Can Help Minimize Security Risks of Artificial Intelligence In today’s rapidly evolving technological landscape, organizations need expert guidance to navigate the complex world of AI security. TTMS stands at the forefront of AI security solutions, offering comprehensive services designed to protect your AI investments and digital assets. Our approach combines deep technical expertise with practical implementation strategies. TTMS provides: Comprehensive AI Security Assessments Thorough evaluation of existing AI systems Identification of potential vulnerabilities Custom-tailored security recommendations Risk analysis and mitigation strategies Advanced Protection Solutions Implementation of robust security frameworks Development of secure AI model architectures Integration of cutting-edge security protocols Regular security updates and maintenance Expert Consultation Services Guidance on AI security best practices Strategic planning for AI implementation Compliance and regulatory advisory Ongoing technical support Training and Development Custom security awareness programs Technical training for IT teams Best practices workshops Regular updates on emerging threats By partnering with TTMS, organizations gain access to industry-leading expertise and proven methodologies for securing their AI systems. Our commitment to staying ahead of emerging threats ensures that your AI investments remain protected in an ever-changing security landscape. Contact us today to learn how we can help strengthen your AI security posture and protect your organization’s valuable assets. Check our AI related Case Studies: AI-Driven SEO Meta Optimization in AEM: Stäubli Case Study Global Coaching Transformation at BVB with Coachbetter App Case Study – AI Implementation for Court Document Analysis Using AI in Corporate Training Development: Case Study Pharma AI – Implementation Case Study at Takeda Pharma What are the security risks of using AI? The security risks of AI encompass various critical vulnerabilities that organizations must address. These include: Data breaches through compromised AI systems Model manipulation through adversarial attacks Privacy violations during data processing Unauthorized access to AI models Biased decision-making due to flawed training data Each of these risks requires specific security measures and ongoing monitoring to ensure AI systems remain secure and reliable. What are the top AI threats in cybersecurity? Current AI cybersecurity threats are becoming increasingly sophisticated. The most significant include: AI-powered phishing attacks that can mimic human behavior Automated hacking attempts using machine learning Deepfake creation for social engineering Data poisoning attacks targeting AI training sets Model extraction and intellectual property theft These AI security threats require organizations to implement robust defense mechanisms and maintain constant vigilance. What are 3 dangers of AI? The three most critical security risks of AI that organizations need to address are: Advanced Cyber Attacks: AI-powered tools can automate and enhance traditional attack methods Privacy Breaches: AI systems may inadvertently expose sensitive data through processing or storage System Manipulation: Adversaries can compromise AI models through targeted attacks and data poisoning What is the biggest risk from AI? The most significant AI security risk lies in adversarial attacks that can manipulate AI systems into making incorrect decisions. These attacks are particularly dangerous because: They can be difficult to detect They exploit fundamental vulnerabilities in AI algorithms They can cause widespread damage before being discovered They often require complex solutions to address What are the risks of relying too much on AI? Over-dependence on AI systems presents several security risk of AI concerns: Reduced human oversight leading to missed security threats Increased vulnerability to AI-specific attack vectors Potential for systematic errors due to AI biases Difficulty in detecting subtle security breaches Challenge in maintaining control over complex AI systems Organizations must maintain a balanced approach, combining AI capabilities with human expertise to ensure robust security measures.
ReadThe EU AI Act is Here: What It Means for Business and AI Innovation
On February 3, 2025, the European Union’s Artificial Intelligence Regulation 2024/1689, widely known as the EU AI Act, officially came into force, marking a significant milestone in AI governance. The regulation introduces comprehensive guidelines for AI development, deployment, and compliance across industries, aiming to balance technological innovation with ethical considerations and security measures. More details on the AI Act can be found on the official AI Act website. A New Era for AI Compliance in the EU The regulation establishes a risk-based classification system for AI applications, categorizing them into prohibited, high-risk, and minimal-risk systems. High-risk AI applications, such as those used in critical infrastructure, healthcare, and employment, will now be subject to stringent compliance measures, including mandatory impact assessments, transparency requirements, and continuous monitoring. One of the most notable aspects of this regulation is the exemption of AI systems used exclusively for military and national security purposes. This decision reflects the EU’s recognition that defence applications require a separate regulatory framework, allowing for continued innovation without the bureaucratic hurdles imposed on civilian AI solutions. AI in Defence: Exemptions and Strategic Considerations The decision to exclude military AI from regulation has significant implications for the defence industry. AI-driven solutions in defence include autonomous threat detection, cybersecurity enhancements, predictive maintenance for military equipment, and battlefield analytics. These applications play a critical role in national security and operational efficiency, requiring agility, secrecy, and technological superiority. The exclusion of military AI from regulatory constraints ensures that defence organizations can continue developing cutting-edge technologies without facing compliance delays. It also highlights the growing intersection between AI and modern warfare, where machine learning models assist in reconnaissance, logistics, and mission planning. Heightened AI Compliance for Civilian Applications While military AI remains unrestricted, companies operating in civilian sectors—including healthcare, finance, and customer service—must adhere to the new EU requirements. The AI Office of the European Commission will oversee compliance, ensuring that AI systems align with ethical principles and human oversight remains a fundamental aspect of AI operations. Additionally, EU member states will play a role in monitoring and enforcing national AI frameworks, as outlined in the AI Pact initiative. Another key aspect of the regulation is its emphasis on transparency and accountability. Organizations using AI must provide clear documentation on their models, detailing training data sources, potential biases, and decision-making processes. This is particularly relevant in sectors where AI influences critical decisions, such as hiring, lending, and medical diagnostics. AI and Data Security: Addressing Concerns The regulation also places a strong focus on data security and privacy, requiring AI developers to implement robust security measures. Companies must ensure that AI systems do not pose cybersecurity risks or facilitate unlawful surveillance and discrimination. One of the regulation’s core goals is to mitigate the risks of AI-generated misinformation and deepfake content. AI tools capable of generating hyper-realistic media are now required to incorporate watermarking and authentication mechanisms to prevent the spread of manipulated information. The Future of AI Governance in the EU The EU’s AI Act sets a global precedent for AI regulation, influencing discussions on AI ethics, security, and responsible innovation. Policymakers worldwide are likely to monitor its implementation closely, shaping future international frameworks. Looking ahead, businesses and organizations using AI must remain proactive in adapting to evolving compliance requirements. The regulatory landscape is expected to continue evolving, with potential updates focusing on emerging AI technologies, human-machine collaboration, and ethical considerations. TTMS and AI Innovation TTMS actively contributes to AI-driven solutions across industries, ensuring that its technologies align with regulatory frameworks while driving innovation. Our expertise in AI, cloud computing, and cybersecurity enables us to support clients in navigating AI compliance and optimizing AI-powered transformation. By leveraging responsible AI practices, we help businesses harness the potential of AI while ensuring transparency, security, and ethical deployment. For more information on how AI is shaping industries, visit our AI Solutions and explore how AI is transforming the defence sector on our Defence page. What is the main purpose of the EU AI Act? The EU AI Act aims to regulate AI technologies by ensuring they align with ethical principles, mitigate risks, and enhance transparency. It classifies AI systems based on risk levels and sets compliance requirements accordingly. Which AI applications are considered high-risk under the Act? High-risk AI applications include those used in critical infrastructure, healthcare, finance, hiring processes, law enforcement, and biometric surveillance. These require strict regulatory oversight, including transparency and human oversight measures. Does the EU AI Act apply to AI used in military applications? No, the EU AI Act explicitly excludes AI systems developed and deployed exclusively for military and national security purposes. This exemption ensures that defence innovation is not hindered by regulatory co How does the EU AI Act impact businesses using AI? Businesses must comply with the regulation’s transparency, data protection, and accountability measures. Companies using AI in decision-making must document their models, ensure human oversight, and comply with ethical guidelines. Where can businesses find guidance on compliance with the AI Act? Businesses can consult the official AI Act website, the AI Office of the European Commission, and legal experts specializing in AI regulation for compliance support.
ReadThe world’s largest corporations have trusted us

We hereby declare that Transition Technologies MS provides IT services on time, with high quality and in accordance with the signed agreement. We recommend TTMS as a trustworthy and reliable provider of Salesforce IT services.

TTMS has really helped us thorough the years in the field of configuration and management of protection relays with the use of various technologies. I do confirm, that the services provided by TTMS are implemented in a timely manner, in accordance with the agreement and duly.
Ready to take your business to the next level?
Let’s talk about how TTMS can help.
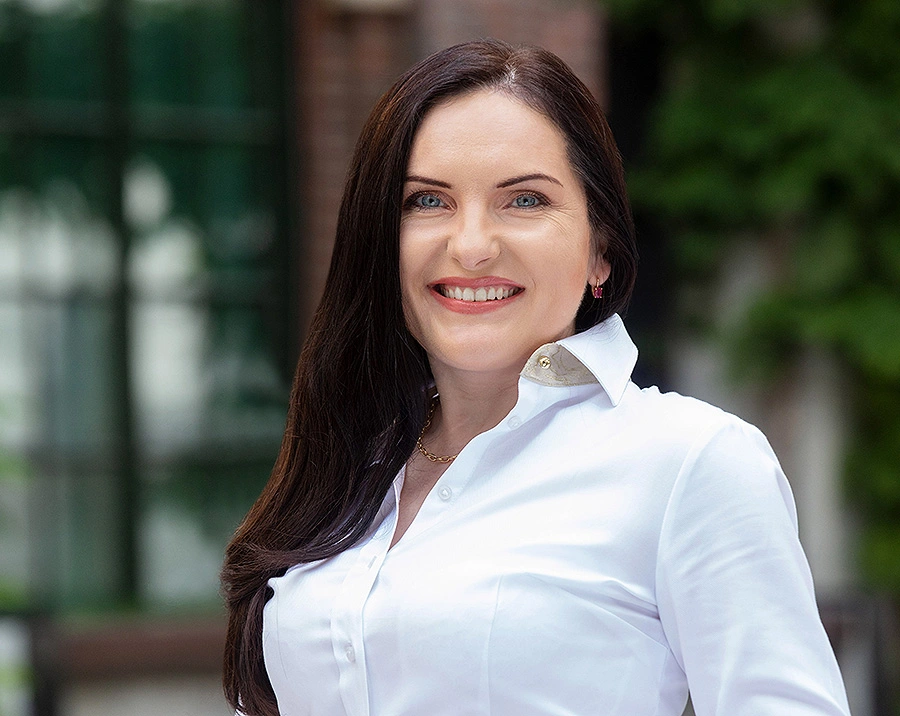