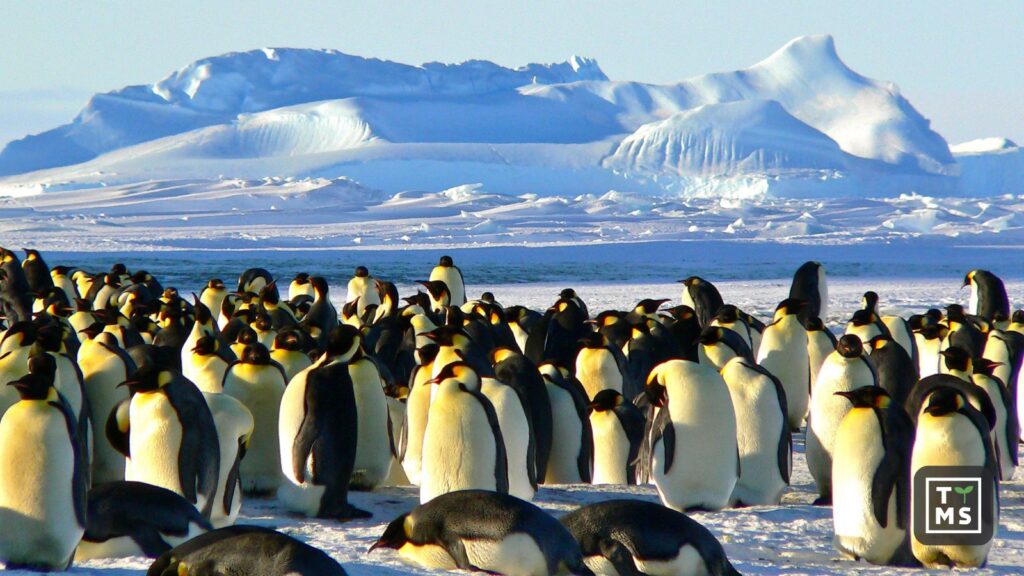
Some species of penguins fall asleep 10,000 times a day. It’s a bit like parents of young children who wake up every five minutes to check if the baby is breathing, to change a diaper, or to feed the infant. Falling asleep 10,000 times a day sounds unbelievable but also fascinating. Nature can be almost as surprising as the idea of penguin researchers studying their sleep habits might seem to the average person. When I think about the amount of data that must have been collected regarding this phenomenon, questions come to mind:
How can something like this be studied? How can such a vast amount of data, stemming from this gigantic number of sleep cycles, be analyzed?
1. Why does humanity collect information in the first place?
To understand the intricate nature of researchers who had enough determination to observe penguins’ habitats, let’s go back to the beginning.
Humanity has been gathering information for thousands of years. Our species intuitively knows that more information leads to better and faster decision-making, a better understanding of the root of problems and complex issues, as well as safety and threat prevention. Initially, information was collected through oral transmissions, then cave paintings, and later increasingly advanced forms of writing.
The transmission of knowledge through writing became commonplace. Initially, the ability to read and write was available only to the wealthiest and the clergy during the Middle Ages. These were the two social groups that once had exclusive access to information conveyed in writing. Over time—with the development of content duplication techniques like printing—the transmission of information became the cause of rapid growth in education and knowledge. The swift development of available printed materials fueled the popularization of literacy skills. More people with these skills accelerated the development of science and industry. The faster advancement of science and industry, in turn, meant that humans could allocate more resources to further scientific progress and conduct more complex research.
At a certain point, we reached a stage where processing data obtained during experiments in paper form was not efficient. Data began to be collected electronically. The emergence of the global internet network was another impulse that accelerated the amount of data being collected, processed, and disseminated.
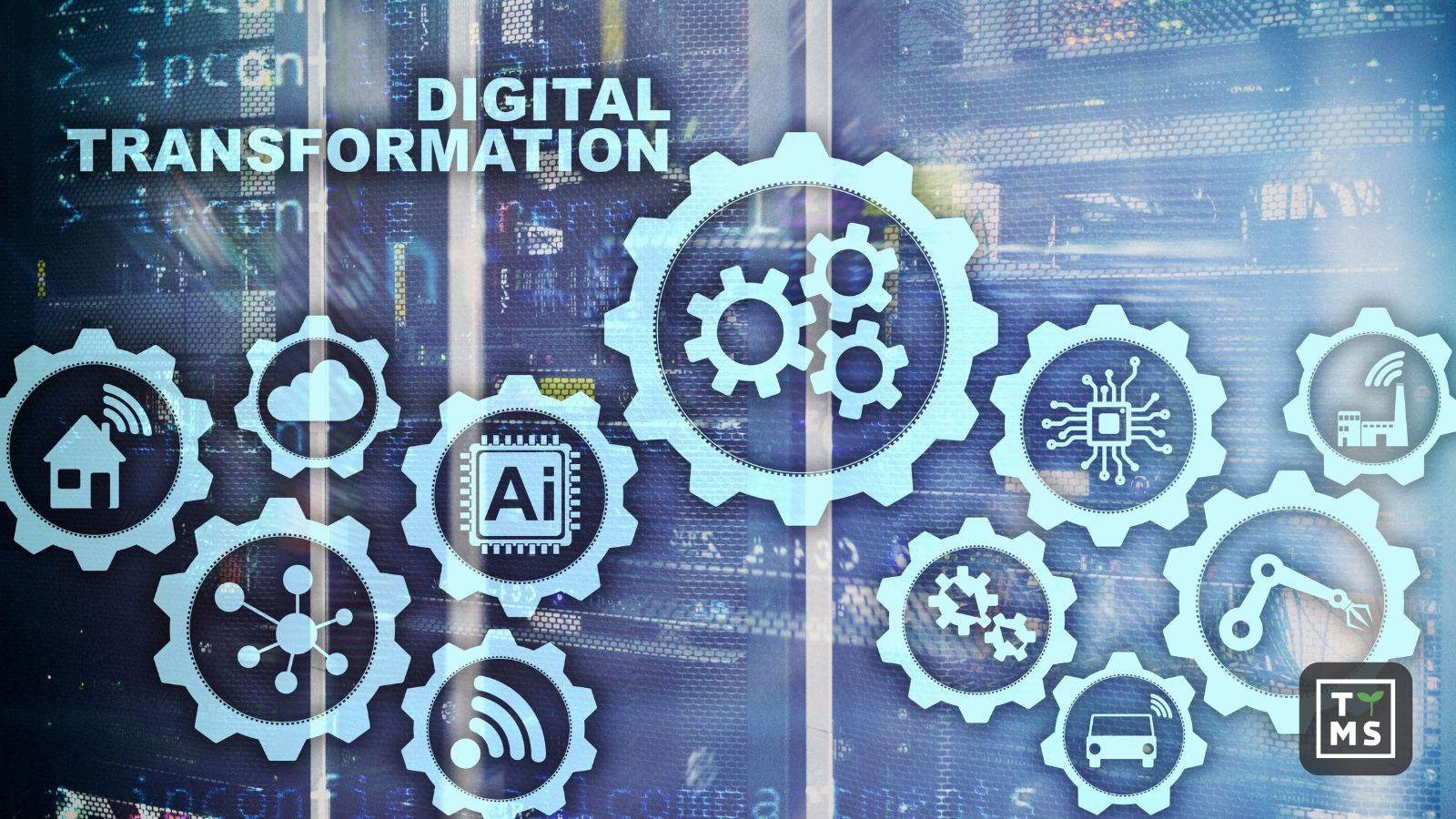
2. The Excel Era
Let’s take a moment to jump back in time to 1985. That’s when Excel was born—a marvelous tool for collecting, processing, distributing, and visualizing data. It allowed users to create forecasts, charts, tables, complex formulas, and even macros that helped quickly process large amounts of data.
The possibilities of using spreadsheets were essentially limited only by the users’ imagination. However, over time, we began to hit the spreadsheet wall. Using them as databases, scheduling tools, or for statistical analysis of vast amounts of data led to the creation of “monsters” several gigabytes in size that could bring any computer to a halt. They also made it impossible to use them on multiple devices simultaneously. Spreadsheets were also unsuitable for storing important information due to technical limitations, such as the lack of version history for changes made to the file. It’s no surprise that over time, this tool began to encounter its own limitations. Applying Excel to tasks it wasn’t originally designed for—like database management or complex statistical analysis—led to performance and technical problems. As a result, despite its versatility, Excel did not meet all the requirements of modern organizations, highlighting the need for more advanced tools.
3. I Appreciate You, but I’m Leaving: Saying Goodbye to Excel
Every enterprise must mature enough to step out of the “Excel cage.” Its versatility means it’s pretty good for everything… but only pretty good. As processes become more complex, it’s necessary to use specialized tools that ensure security and quick analysis of key data and processes.
To illustrate this problem, one might attempt to draw a timeline with specific points: from a finger smeared in dye, through cuneiform writing, paper, Excel, all the way to AI. There’s no going back to cave paintings—that seems logical to us. Yet we still have to convince others that the paper era is over, and Excel’s time has just ended.
In times when humanity is producing more and more data every day, can we afford to use paper? Paper, which is only slightly better than a clay tablet?
These are, of course, rhetorical questions.
Regarding the penguins—to check if a bird is sleeping, it was necessary to analyze much more than just its sleep. Parameters such as brain electrical activity (independently for each hemisphere), body movements, neck muscle activity, and even the depths at which the birds hunted fish in the ocean were examined. The observation results were surprising. It turned out that the birds didn’t sleep for long periods. An average penguin nap lasted 1–4 seconds. However, it’s worth mentioning that there could be several hundred such naps per hour. When summing up all the moments devoted to sleep, it turned out that the animals could sleep up to 15 hours a day.
In this particular case, Excel was sufficient because the analysis was conducted for only 14 individuals. However, as you might guess, with a larger number, computational tool performance issues could arise.
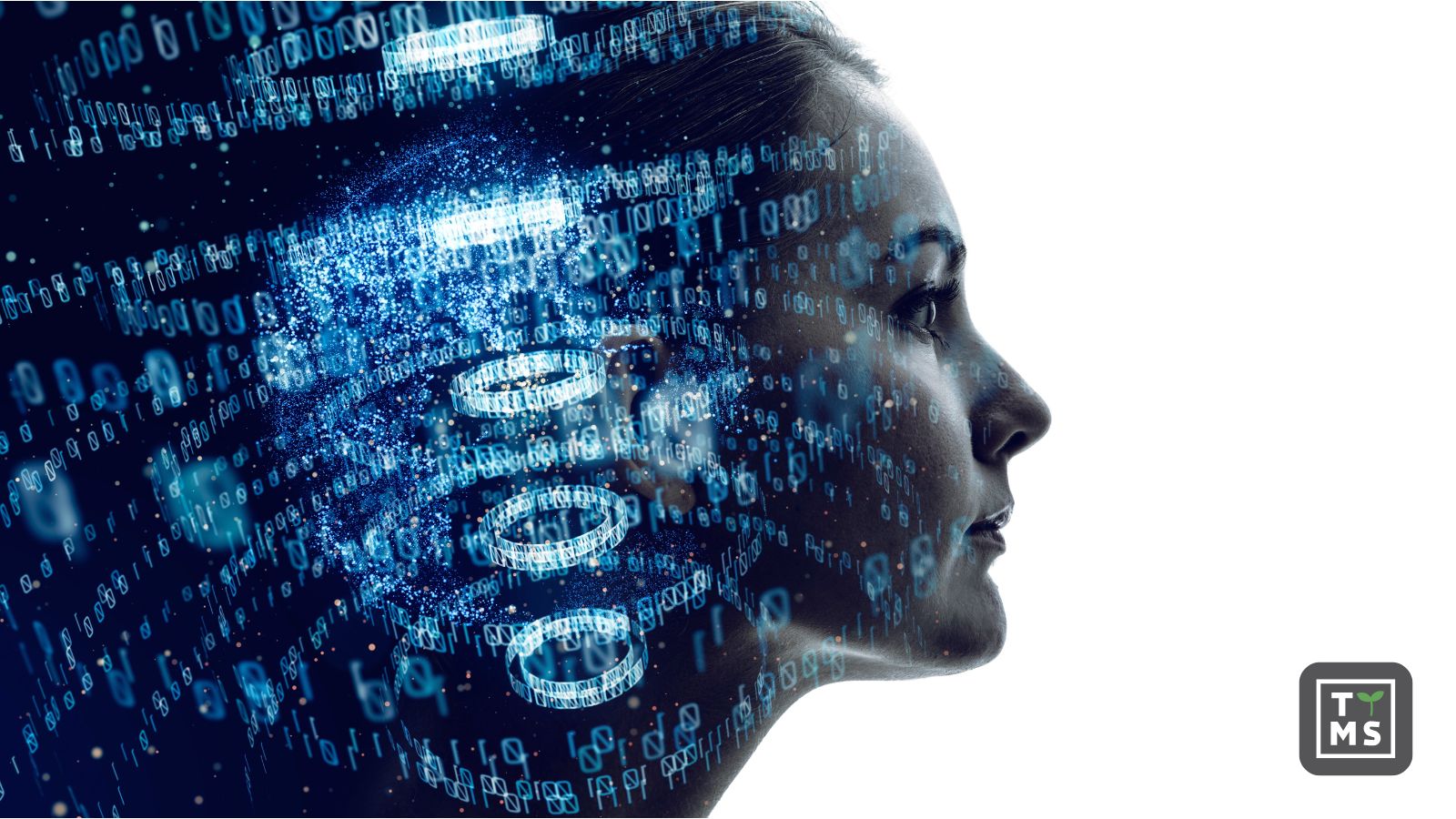
4. How to Analyze, Process, and Store Data in 2024
The aforementioned penguins were studied for parameters that could indicate they were falling asleep. They would fall asleep for a few seconds, even up to 600 times an hour. This means that measurements had to be taken at a frequency of at least every 0.5 seconds. One parameter would occupy 170,000 cells in a spreadsheet for just one bird per day. Over 10 days, this amount would increase to 1,700,000. Multiplying this result by 14 (the total number of studied individuals), we get nearly 24 million cells. If we then multiply this by 10 different parameters (which were also studied), we obtain 240 million cells filled with the vital parameters of 14 penguins.
If we measure even more parameters, we hit the wall of the spreadsheet’s cell limit.
A similar problem occurs in any quality process we might want to conduct using Excel. If the process requires implementing an audit trail (a chronological record of all operations), the spreadsheet’s size begins to increase very rapidly.
Of course, Excel is a much better place to store data than the clay tablets and papyrus mentioned multiple times in this article. However, it is not suitable for use as a database. That’s why dedicated tools are used for data collection. Here are a few of them:
- MES Systems (Manufacturing Execution System): Systems for supervising and controlling the production process, ensuring proper parameters and efficiency, allowing you to monitor and plan production processes.
- ERP Systems (Enterprise Resource Planning): Help in managing the entire enterprise.
- EDMS (Electronic Document Management System): Enable and facilitate control over the quality and availability of documents.
All the above categories (and many others) require proper infrastructure and maintenance. It’s worth mentioning that each of these systems can be supported to some extent by AI. Performing scoring and analyzing vast amounts of data is something AI excels at. This allows for optimizing processes in ways not available in simple tools like Excel.
In many cases, including the validation of computerized systems, determining user requirements and properly understanding their needs is crucial for the safe and efficient operation of any computer system—by the user themselves as well.
“Blind optimism” from the client, which may arise after an excellent sales presentation of a system’s demo version, is not good practice because it usually doesn’t consider the real business needs, system capabilities, and infrastructure.
Suppliers are generally interested in good cooperation with the client and providing what they genuinely need, but they often can’t spend enough time with them to assess their real needs. This is especially true when implementing a tool is something new, and the user isn’t always aware of their needs (e.g., validation and change tracking, which may turn out to be required—something Excel does not support to the extent needed for large amounts of data. An improperly chosen tool may require unfeasible customizations to implement the proper solution).
For example, the penguin researchers started by developing a methodology, checking previous publications on the subject, and considering what needed to be studied to obtain specific data.
In computer systems, this stage is called “defining user requirements”.
To enable a company to effectively determine what functionalities a new system for collecting, storing, and processing data—meant to replace Excel—should have, it’s worth going through several key steps:
- Analysis of Business Requirements: The company should gather and analyze all needs and goals related to data across the organization. It’s important to understand which processes are to be supported by the new system and what specific problems need to be solved.
- Engagement of Key Users: It’s beneficial to conduct interviews, surveys, or workshops with employees who currently use Excel. This way, you can learn about their daily needs and discover the limitations and challenges they face.
- Mapping Processes and Data Flows: The company should trace how data flows in different departments. Clear process mapping allows for identifying which functionalities are needed to automate, streamline, or integrate different stages of working with data.
- Identification of Key Functions and Excel’s Shortcomings: Analyzing where Excel does not meet expectations is crucial. For example, there may be a lack of simultaneous multi-user access, advanced reporting, real-time analysis, integration with other systems, or ensuring an appropriate level of security.
- Analysis of Available Technologies and Solutions: The company should explore market-available solutions that offer functionalities required to achieve the set goals.
- Defining Priorities and Essential Functionalities: After gathering all requirements, the company should create a prioritized list of functionalities. Key functions may include storing large volumes of data, real-time analytics, automatic reporting, data security, or the ability to integrate with other systems.
- Testing Solutions: If possible, it’s worth conducting tests of selected solutions with the participation of end-users. This will allow the company to assess how new functions work in practice and whether they meet the employees’ needs.
- Selecting a Vendor and Pilot Implementation: After initial tests, the company can choose a system vendor that best meets the requirements and conduct a pilot implementation. Such a pilot allows for adapting the system to the company’s specific work environment before full deployment.
By going through these steps, the company will be able to precisely determine what functionalities are needed in the new system and choose a solution that best fits its requirements.
That’s why more and more enterprises, especially in industries where data collection is crucial, are moving away from spreadsheets in favor of more advanced tools. This is an obvious consequence resulting from the growth of every company.
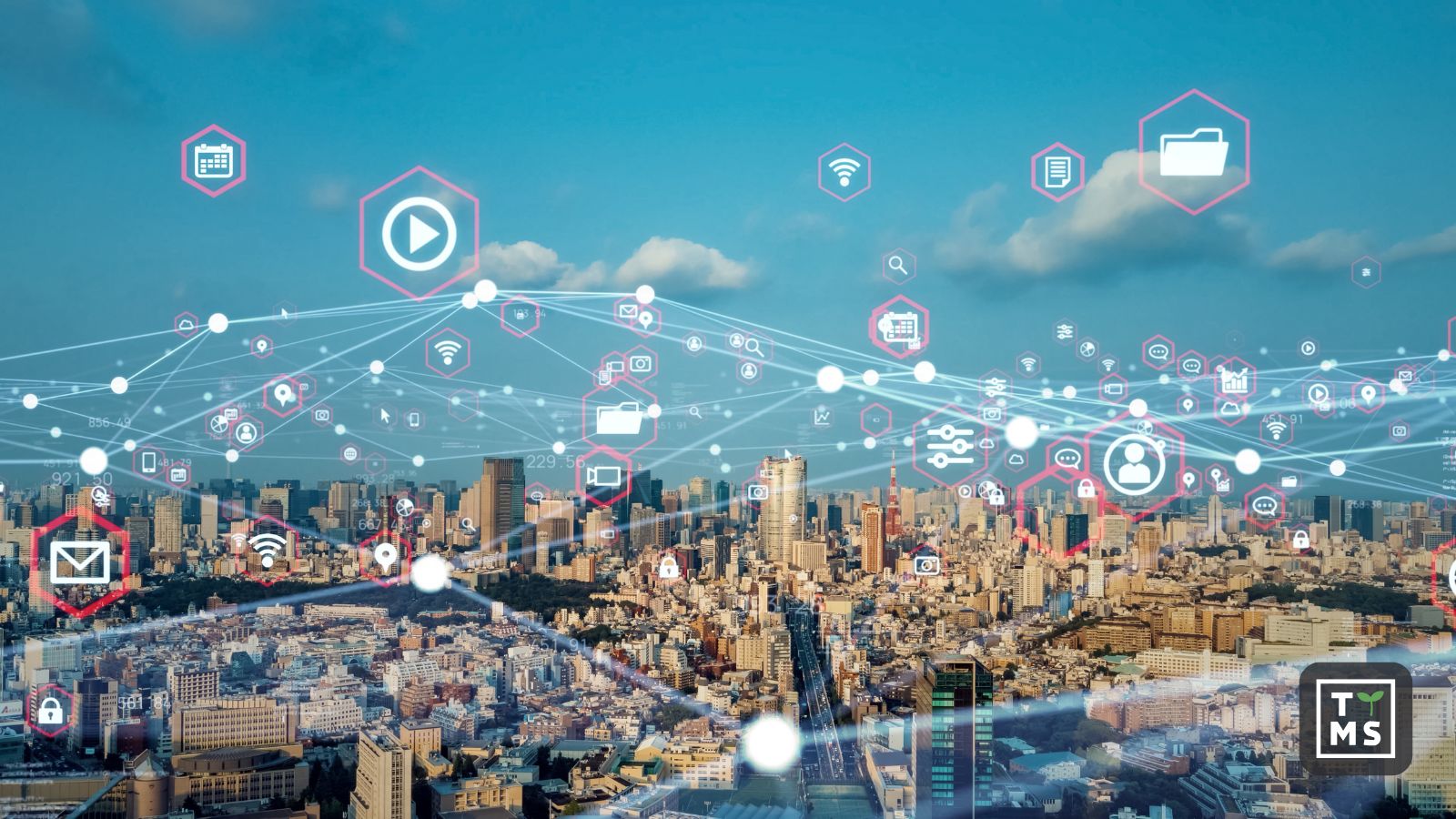
5. Modern Data Management – Summary and Conclusions
I have addressed the complexity of collecting, storing, and analyzing data using the example of research on penguin sleep to show that transitioning from Excel to dedicated systems like ERP, MES, and EDMS is necessary. Such solutions are indispensable for companies processing vast amounts of data.
In the digital age, traditional spreadsheets no longer meet the needs of dynamic businesses, and optimal data management requires professional tools and an understanding of real user requirements.
Returning to the topic of the penguins themselves—imagine someone falling asleep and waking up 600 times an hour. You could say they are almost always asleep, waking only to take care of something. This resembles a user during system implementation who hasn’t thoroughly considered their needs. It is the user and their needs that are crucial in any implementation. Often, the user cannot properly define their expectations, is unaware of the necessity of conducting validation, or conversely—thinks it is essential, leading to unnecessary expenses.
“Overquality”—this is a word I just invented, but it aptly describes the phenomenon of excessive attention to quality.
And what about “underquality”? Here, the issue is much more serious—it can lead to disruptions in key business processes, endangering patient safety, product quality, and reducing the company’s profitability.
And what does this have to do with Excel? Every enterprise should care about the safety and efficiency of its business processes. To achieve this, these processes should be supported by appropriate tools that specialists in this field can provide.
If this article interested you, if you have questions or need support in digital transformation, leave us a message. Our team of Quality Management experts will help meet your expectations in the area of digital change.
Meet our case studies in data management and digital transformation:
- Automated Workforce Management System Case Study
- Supply Chain Management Case Study: Cost Improvement
- Case Study: Customized Finance Management System in Enterprise
- Example of How We Improved Reporting and Data Analysis Efficiency
- Consent Management Platform Integration in Pharma Case Study
- Effective Consent Lifecycle Management in Pharma Case Study
- Global Coaching Transformation at BVB with Coachbetter App
- A Pharma Platform Case Study – Implementing a Digital Health
- Operation Crocodile – building a new service center